A Transferable Adversarial Attack Algorithm Based On Spatial Transformation
2023 IEEE 6th International Conference on Big Data and Artificial Intelligence (BDAI)(2023)
摘要
Artificial intelligence technology represented by deep learning has been widely used in the real world, and its security has elicited increasing interest. Recent studies have shown that compared to white-box attacks, black-box adversarial attacks pose more serious security threats to deep neural networks. Therefore, evaluating model robustness by studying black-box adversarial attacks has become a research hotspot in the field of deep learning security. However, the existing adversarial attack methods generally suffer from the conflict between attack strength and imperceptibility. To solve this problem, this paper explores adversarial samples that are more in line with human perception of the more transferable intermediate layer features and proposes an intermediate layer adversarial attack algorithm stFA based on pixel spatial transformation. The proposed stFA promotes the model to misclassify adversarial samples as target labels, while encouraging the intermediate-layer feature representation of clean images to move away from the original labels. Extensive experiments have shown that stFA has both perfect imperceptibility and black-box transferability, which improves the success rate of targeted and untargeted attacks by 7.8% and 4.4% compared to stAdv.
更多查看译文
关键词
deep learning,adversarial samples,spatial transformation,transferability,intermediate layer features
AI 理解论文
溯源树
样例
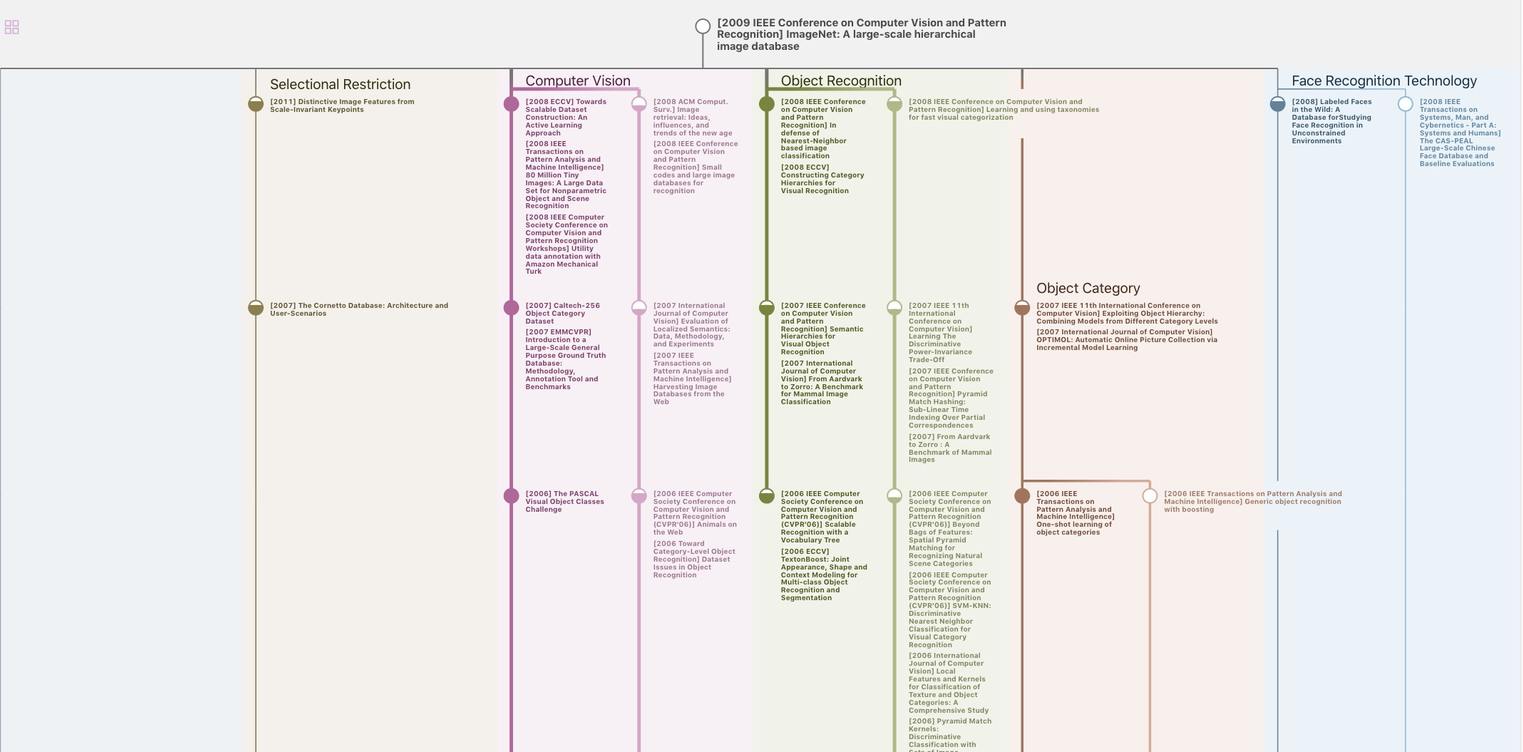
生成溯源树,研究论文发展脉络
Chat Paper
正在生成论文摘要