A Comparison Study to Detect Malware using Deep Learning and Machine learning Techniques
2023 IEEE 6th International Conference on Big Data and Artificial Intelligence (BDAI)(2023)
摘要
Malware creation has evolved from basic malware that is easy to detect to complicated malware that is obfuscated and quickly adaptive, raising the challenge of being easily detected. This study compares seven machine learning and deep learning techniques in detecting malware by using the extracted byte, opcode, and section codes. In this research, we aim to classify malware in nine different malware families correctly. First, the byte codes, the section codes, and the opcodes of the different malware applications are extracted and merged, and the classification is done by using the Random Forest, Decision Tree, Support Vector Machine, K-nearest neighbor, SGD, Logistic Regression, Näıve Bayes, and deep learning techniques. The result shows that the deep learning model outperforms other compared machine learning algorithms with an accuracy of 96%. Overall, the paper highlights the importance of using advanced machine learning and deep learning techniques for the detection of malware, particularly given the increasing complexity and adaptiveness of modern malware. The findings of the paper suggest that deep learning techniques may be particularly effective for detecting and correctly classifying malware.
更多查看译文
关键词
Machine learning,malware detection,byte codes,section,opcodes,random forest,decision tree,support vector machine (classifier),Knearest neighbor,SGD,Logistic regression,Näıve Bayes,Deep Learning Model,Malware Classification,Machine Learning,Windows PC Malware
AI 理解论文
溯源树
样例
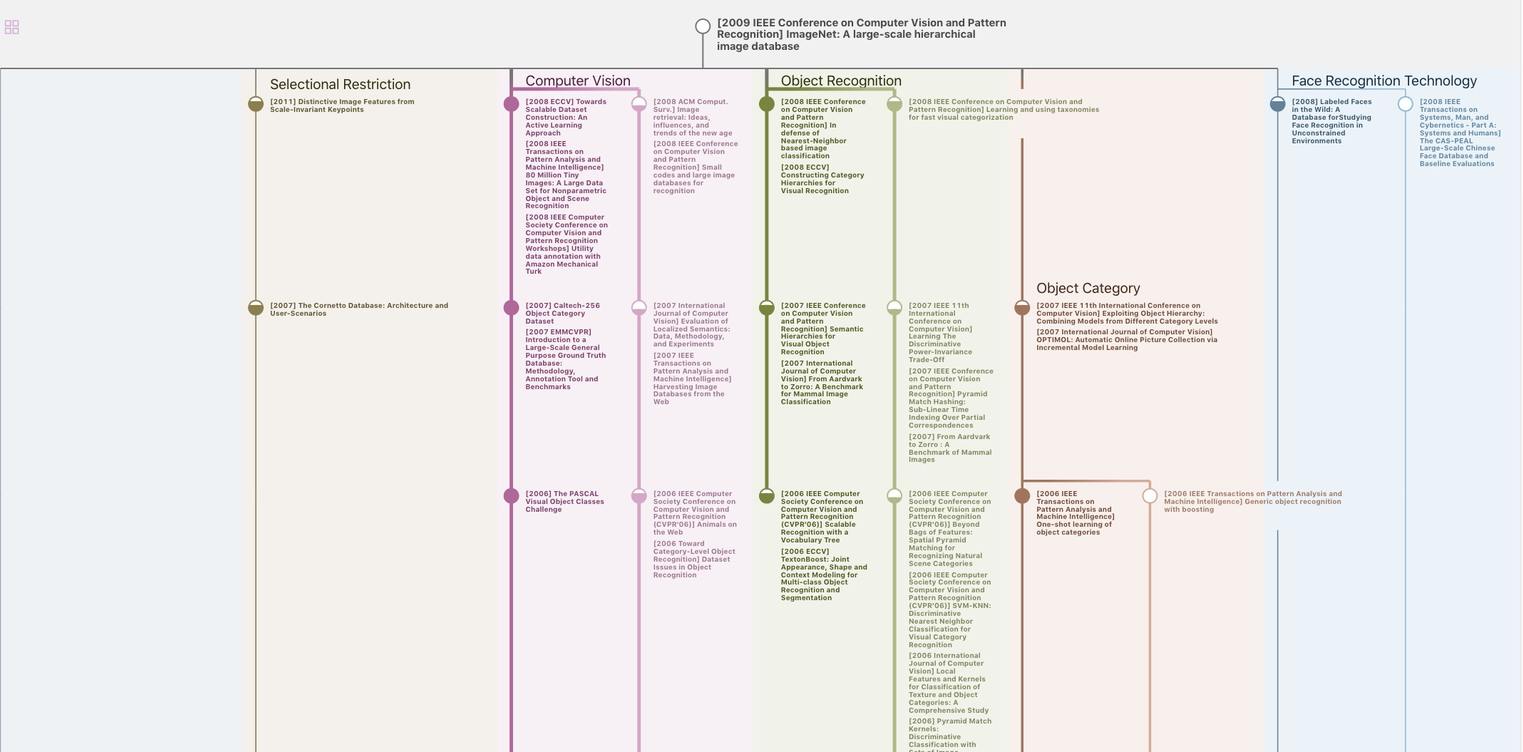
生成溯源树,研究论文发展脉络
Chat Paper
正在生成论文摘要