Short-term power load forecasting system based on rough set, information granule and multi-objective optimization
Applied Soft Computing(2023)
摘要
Accurately forecasting power load is essential for utilities to effectively manage their resources, reduce operational costs, and provide improved customer service. However, the current load forecasting lacks the ability to deeply explore data, thus failing to accurately predict both short-term trends and volatility ranges. To address this issue, we construct a novel combined forecasting system based on rough sets, information granulation, deep learning, and multi-objective optimization. In this study, we follow the reasonable granulation criterion for granular computing, which aims to improve the reasonableness and specificity of granular interval prediction under the determination of granularity level, and innovatively propose a novel multi-objective optimization algorithm that can simultaneously constrain the reasonable granulation criterion and theoretically demonstrate the obtained Pareto-optimal solution. Four simulation experiments were conducted using the Australian dataset to evaluate the performance of our proposed system in predicting trend changes and fluctuation ranges of power load. Our results demonstrate that the developed system effectively predicts the trend changes and fluctuation range of power load. Specifically, our system showed a deterministic prediction performance improvement of 13.39% and a granularity interval prediction performance improvement of 6.67% compared to the baseline model. Moreover, we conducted a series of discussion tests to validate the superiority of our system, which further confirmed the effectiveness of our proposed approach.
更多查看译文
关键词
rough set,information granule,optimization,short-term,multi-objective
AI 理解论文
溯源树
样例
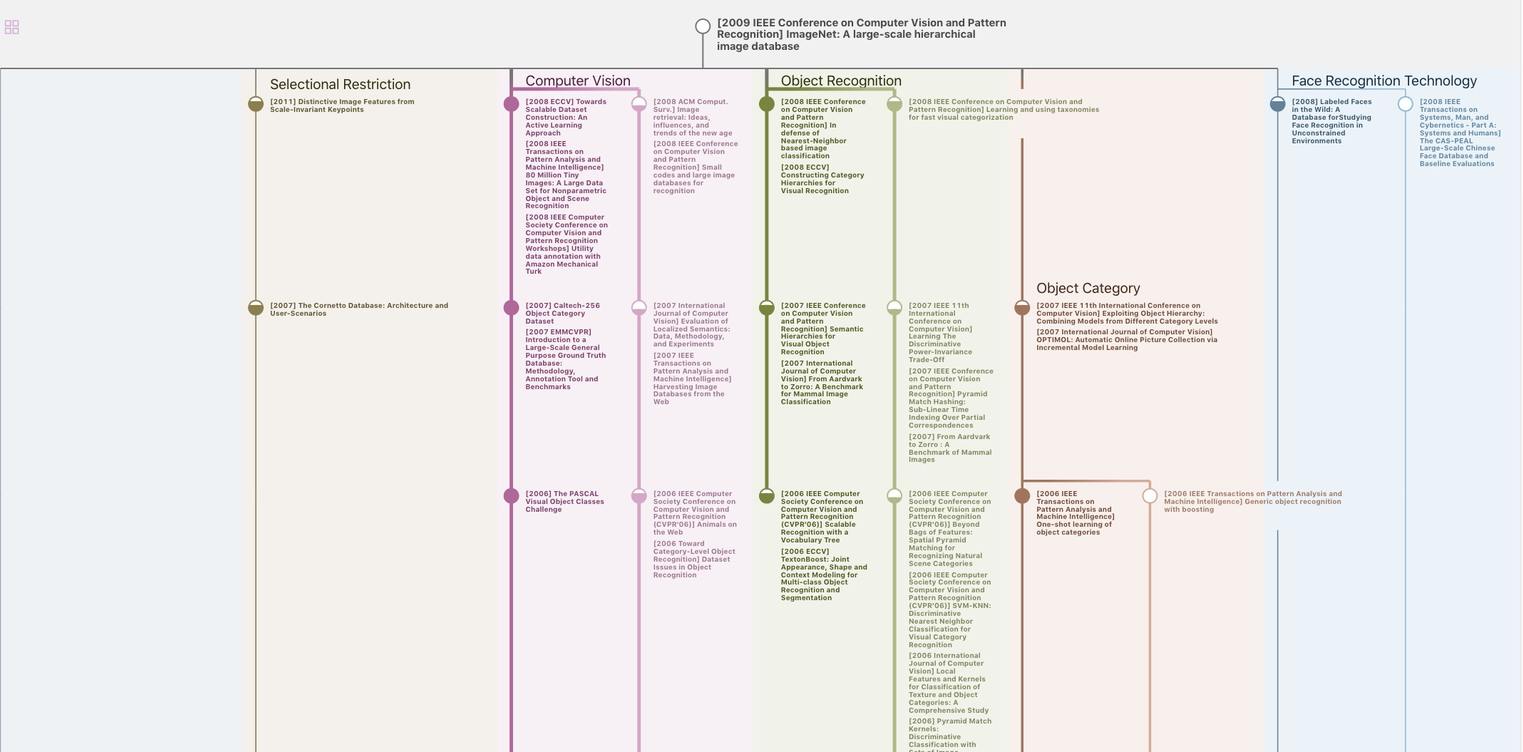
生成溯源树,研究论文发展脉络
Chat Paper
正在生成论文摘要