Multi-unit soft sensing permits few-shot learning
CoRR(2023)
摘要
Recent literature has explored various ways to improve soft sensors using learning algorithms with transferability. Broadly put, the performance of a soft sensor may be strengthened when it is learned by solving multiple tasks. The usefulness of transferability depends on how strongly related the devised learning tasks are. A particularly relevant case for transferability, is when a soft sensor is to be developed for a process of which there are many realizations, e.g. system or device with many implementations from which data is available. Then, each realization presents a soft sensor learning task, and it is reasonable to expect that the different tasks are strongly related. Applying transferability in this setting leads to what we call multi-unit soft sensing, where a soft sensor models a process by learning from data from all of its realizations. This paper explores the learning abilities of a multi-unit soft sensor, which is formulated as a hierarchical model and implemented using a deep neural network. In particular, we investigate how well the soft sensor generalizes as the number of units increase. Using a large industrial dataset, we demonstrate that, when the soft sensor is learned from a sufficient number of tasks, it permits few-shot learning on data from new units. Surprisingly, regarding the difficulty of the task, few-shot learning on 1-3 data points often leads to a high performance on new units.
更多查看译文
关键词
learning,sensing,multi-unit,few-shot
AI 理解论文
溯源树
样例
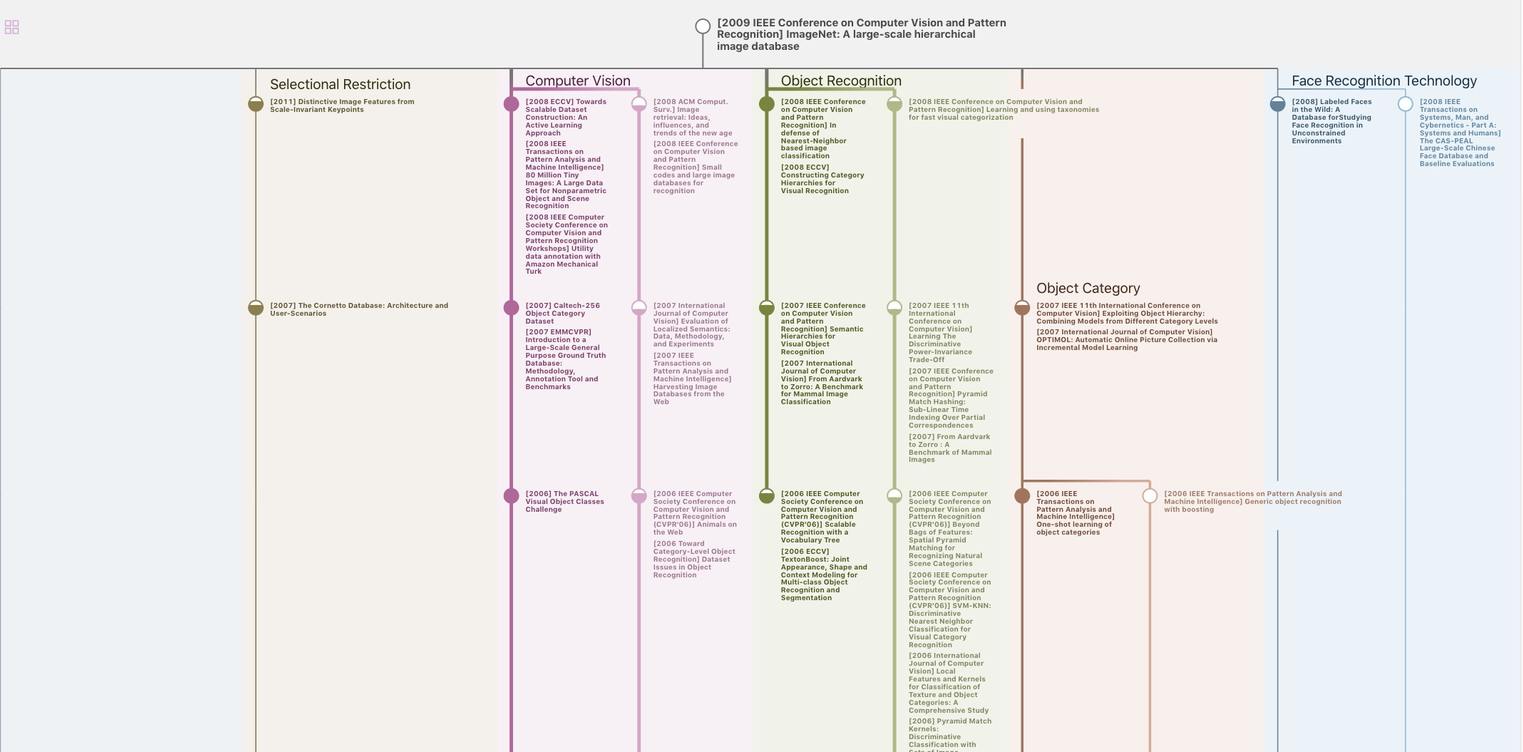
生成溯源树,研究论文发展脉络
Chat Paper
正在生成论文摘要