Federated Deep Equilibrium Learning: A Compact Shared Representation for Edge Communication Efficiency
CoRR(2023)
摘要
Federated Learning (FL) is a prominent distributed learning paradigm facilitating collaboration among nodes within an edge network to co-train a global model without centralizing data. By shifting computation to the network edge, FL offers robust and responsive edge-AI solutions and enhance privacy-preservation. However, deploying deep FL models within edge environments is often hindered by communication bottlenecks, data heterogeneity, and memory limitations. To address these challenges jointly, we introduce FeDEQ, a pioneering FL framework that effectively employs deep equilibrium learning and consensus optimization to exploit a compact shared data representation across edge nodes, allowing the derivation of personalized models specific to each node. We delve into a unique model structure composed of an equilibrium layer followed by traditional neural network layers. Here, the equilibrium layer functions as a global feature representation that edge nodes can adapt to personalize their local layers. Capitalizing on FeDEQ's compactness and representation power, we present a novel distributed algorithm rooted in the alternating direction method of multipliers (ADMM) consensus optimization and theoretically establish its convergence for smooth objectives. Experiments across various benchmarks demonstrate that FeDEQ achieves performance comparable to state-of-the-art personalized methods while employing models of up to 4 times smaller in communication size and 1.5 times lower memory footprint during training.
更多查看译文
关键词
compact shared representation,communication,equilibrium,learning
AI 理解论文
溯源树
样例
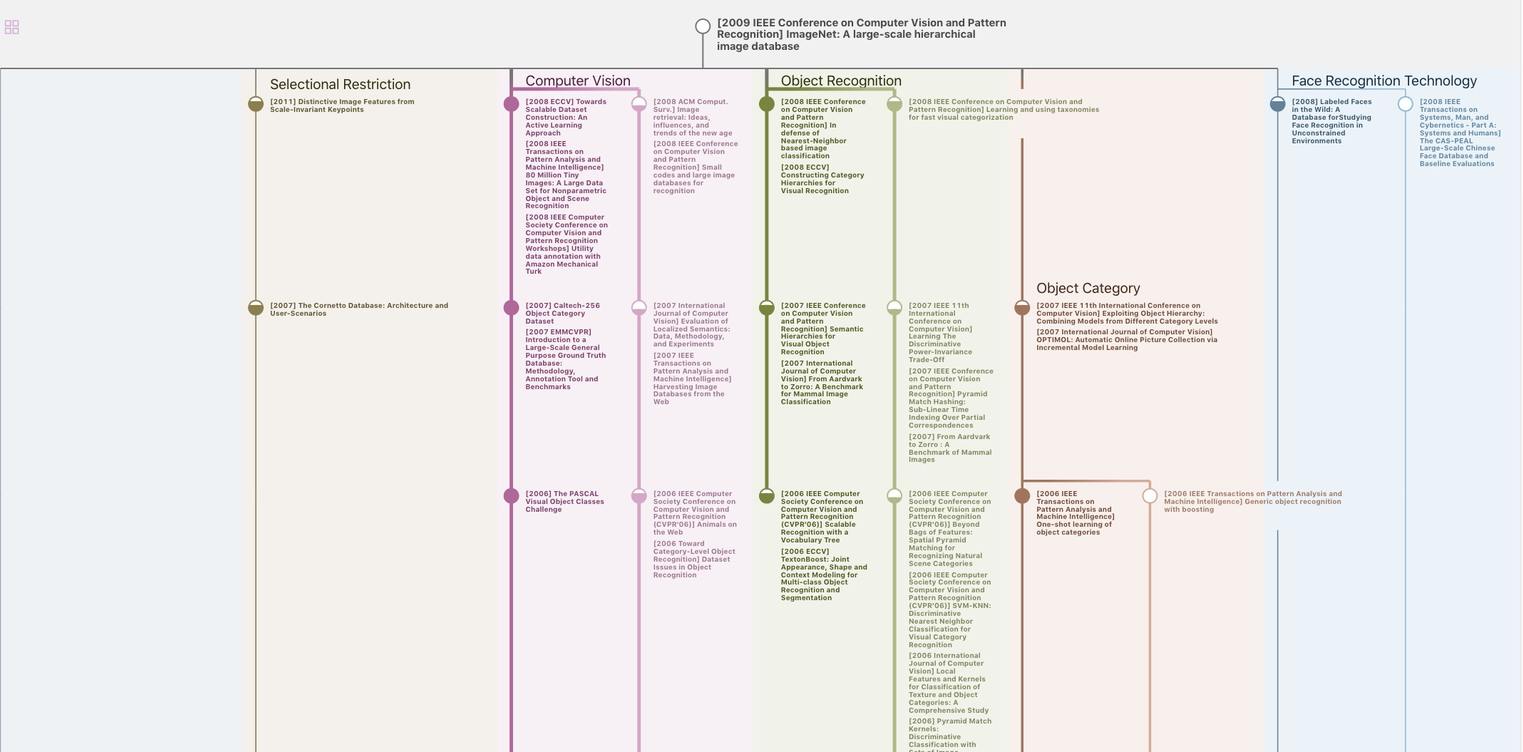
生成溯源树,研究论文发展脉络
Chat Paper
正在生成论文摘要