Detection of prodromal Parkinson’s disease using a urine proteomics panel and machine learning
medRxiv (Cold Spring Harbor Laboratory)(2023)
摘要
Parkinson’s disease is a progressive neurodegenerative disorder and idiopathic REM-sleep behaviour disorder (iRBD) has been identified as its single most specific early symptom. To facilitate the screening of individuals at high risk to develop Parkinson’s disease, we developed a multiplexed panel of urine proteomics using machine learning and targeted mass spectrometry to detect iRBD. Random urine samples from clinically and genetically well characterized patients with iRBD, idiopathic and hereditary forms of Parkinson’s disease, and matching controls, collected in two academic centres, were analysed in a standardized way.
First, a biomarker discovery and exploratory comparison of samples from randomly selected idiopathic Parkinson’s patients and age/sex-matched healthy controls were proteomically profiled and quantified (> 2500 proteins). The most differentially expressed biomarkers were combined into a high-throughput, multiplexed assay using targeted proteomics designed for use of tandem mass spectrometers for potential translation into clinical practise. This was then validated on independent patient and control samples (n=184). After detecting a major influence of sex on the proteome, we focused subsequent analyses on the larger group of available male samples ( n = 114) and report results based on iRBD ( n = 14), idiopathic ( n = 35) and young-onset Parkinson’s disease ( n = 15), carriers of LRRK2 ( n = 10), PARKIN-gene mutations ( n = 5), and healthy control subjects ( n = 35). After establishing excellent compatibility between the two study sites, orthogonal partial least squares discriminant analysis (OPLS-DA) excluded a relevant effect of aging, but detected significant differences between iRBD and healthy controls (ANOVA-CV P = 0.002), as well as the combination of iPD/iRBD and healthy controls (ANOVA-CV P = 0.01).
Uni- and multivariate analyses detected a shared expression pattern for the protein biomarkers UBC, NCAM1, MIEN1, SPP2, REG1B, ITIH2, BCHE and C3 between iRBD and idiopathic Parkinson’s disease. Utilizing split train/test-datasets in a multiple-regression classifier model resulted in a mean accuracy of 78% to detect iRBD, matching iRBD’s conversion rate to Parkinson’s disease. Hierarchical clustering revealed greater similarities in urine proteomic changes between iRBD and idiopathic than monogenic Parkinson’s disease. Several proteins identified correlated either with clinical severity (e.g. VCAM1, MSN, HPX), or risk for future conversion to Parkinson’s disease (VCAM1, MSN, MYO10, HSPAIL).
This demonstrates the power of machine learning and urine biomarkers to identify iRBD patients. As we develop new therapies and interventions, the ability to detect individuals at-risk of neurodegeneration in very early disease stage will be invaluable for treatment success.
### Competing Interest Statement
The authors have declared no competing interest.
### Funding Statement
SRS received support from the Horizon 2020 Framework Programme (grant number 634821, PROPAG-AGEING) and the Advanced Clinician Scientist Program by the Interdisciplinary Centre for Clinical Research, Wuerzburg, Germany, he is a Fellow of the Thiemann Foundation. KK, MS and EF received funding from the Slovak Grant and Development Agency under contracts no. APVV-18-0547 and APV-22-0279, and by the Slovak Scientific Grant Agency under contract no. VEGA 1/0712/22
### Author Declarations
I confirm all relevant ethical guidelines have been followed, and any necessary IRB and/or ethics committee approvals have been obtained.
Yes
The details of the IRB/oversight body that provided approval or exemption for the research described are given below:
IRB of University College London and Kosice gave ethical approval for this work.
I confirm that all necessary patient/participant consent has been obtained and the appropriate institutional forms have been archived, and that any patient/participant/sample identifiers included were not known to anyone (e.g., hospital staff, patients or participants themselves) outside the research group so cannot be used to identify individuals.
Yes
I understand that all clinical trials and any other prospective interventional studies must be registered with an ICMJE-approved registry, such as ClinicalTrials.gov. I confirm that any such study reported in the manuscript has been registered and the trial registration ID is provided (note: if posting a prospective study registered retrospectively, please provide a statement in the trial ID field explaining why the study was not registered in advance).
Yes
I have followed all appropriate research reporting guidelines, such as any relevant EQUATOR Network research reporting checklist(s) and other pertinent material, if applicable.
Yes
All data produced in the present study are available upon reasonable request to the authors
[https://panoramaweb.org/urine\_irbd\_trgt_proteomics.url][1].
[1]: https://panoramaweb.org/urine_irbd_trgt_proteomics.url
更多查看译文
关键词
urine proteomics,prodromal parkinson,machine learning
AI 理解论文
溯源树
样例
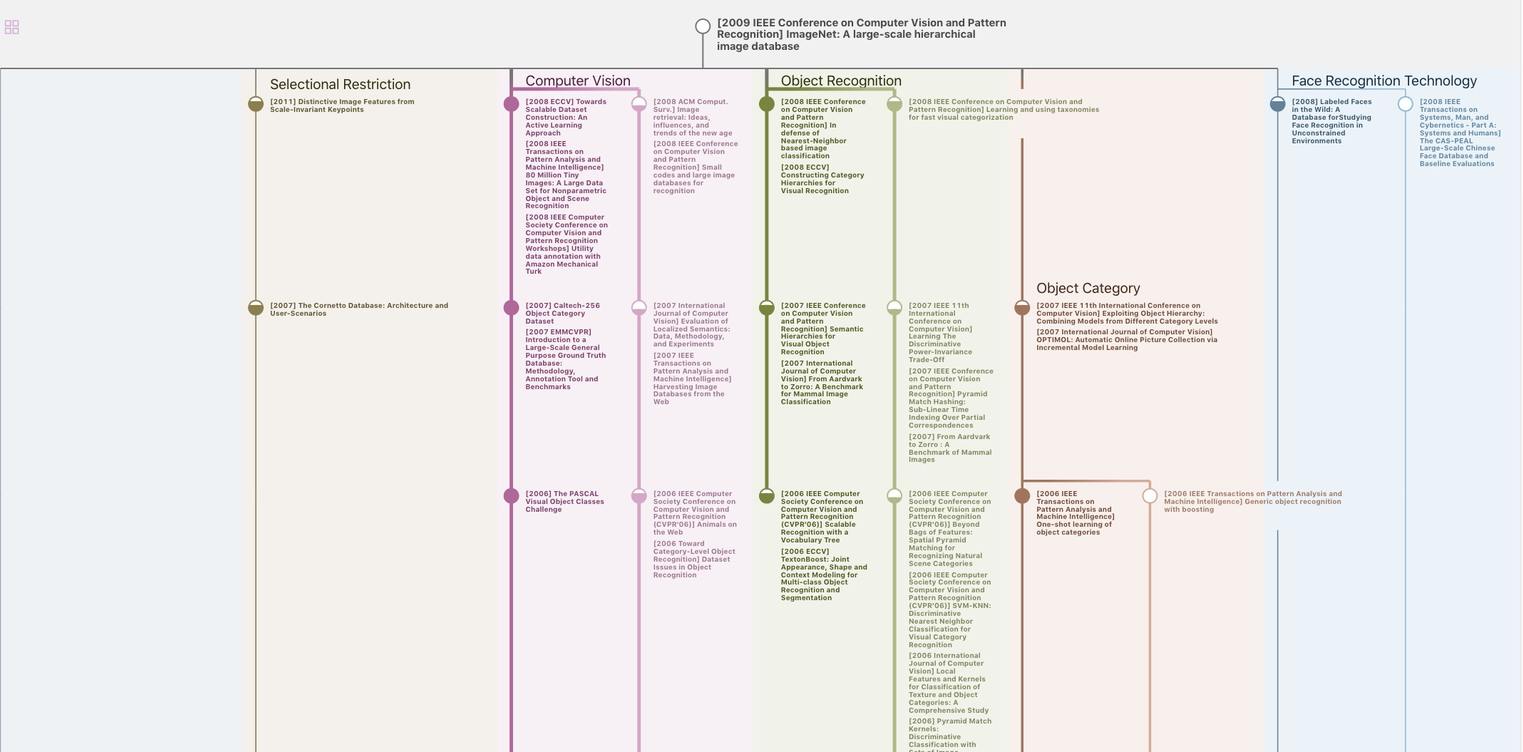
生成溯源树,研究论文发展脉络
Chat Paper
正在生成论文摘要