SAGPCN: Self-Attention Graph Pooling Convolutional Network for Battery State of Health Estimation
2023 IEEE 3rd International Conference on Industrial Electronics for Sustainable Energy Systems (IESES)(2023)
摘要
Lithium-ion batteries are widely applied in sustainable energy conversion system. Consequently, it is of great research significance to accurately estimate the state of health (SOH) of batteries. To effectively model the input features at the spatial level, this article proposes a self-attention graph pooling convolutional network (SAGPCN) to estimate the SOH. The advantages of SAGPCN proposed in this paper can be reflected as follows: (1) The SAGPCN can consider node characteristics and graph topology, which focuses the attention on key parts of the graph. (2) The SAGPCN designs a self-attention mechanism to reserve significant nodes and delete secondary nodes, so as to optimize the network structure. A real-world dataset is adopted to evaluate the proposed battery SOH estimation approach in this paper. Experimental results represent that the estimation performance of the proposed SAGPCN is better than some data-driven SOH prediction approaches.
更多查看译文
关键词
Lithium-ion battery,Self-attention graph pooling convolutional network,State of health,Estimation
AI 理解论文
溯源树
样例
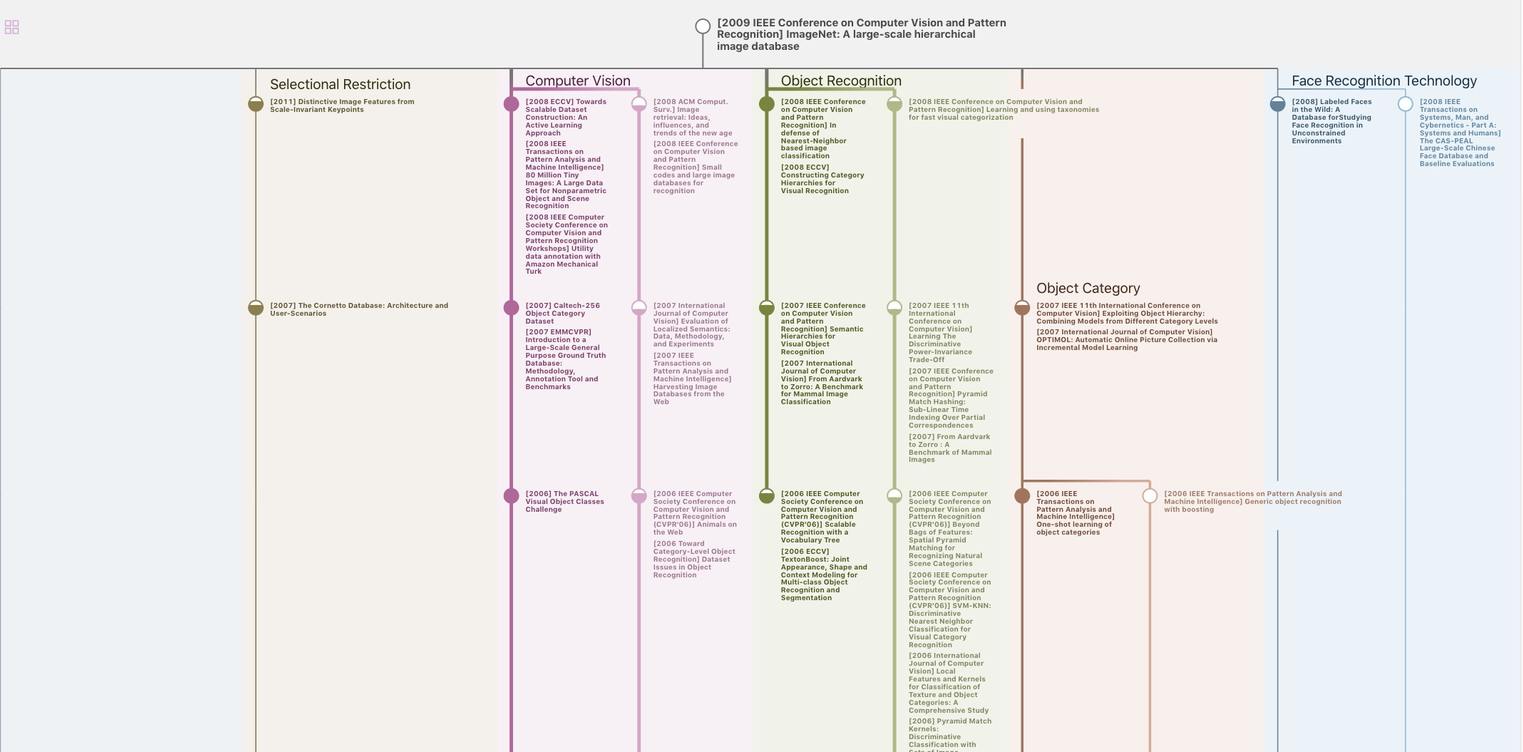
生成溯源树,研究论文发展脉络
Chat Paper
正在生成论文摘要