Metalens: Federated Meta-Learning Ensemble Using Flexible Classifiers on Non-IID Data
2023 24st Asia-Pacific Network Operations and Management Symposium (APNOMS)(2023)
摘要
With the blossoming of the Internet of Things (IoT), the explosive growth of data across edge devices rises unprecedentedly. However, most of the collected data is privacy sensitive and heterogeneous across various IoT devices, e.g., smartphones and wearable devices. To address the challenges, Federated Meta-Learning (FML) emerges as a prominent solution by training a global model that can be tailored to local user data on IoT devices. Existing FML algorithms require a global model with an output dimension large enough to cover the classes possessed by all users. In the face of performance degradation resulting from large numbers of classes, we propose an innovative paradigm: federated Meta-Learning Ensemble (Metalens), which enables users to train in synergy with a smaller output dimension by integrating FML and ensemble techniques. While a smaller output dimension has performance-wise advantages, it creates another issue, namely out-of-distribution (OOD) classes; in response to the challenge, we devise an elegant ensemble scheme to regulate OOD effects. The performance evaluations show that a smaller model output dimension is advantageous and outperforms other state-of-the-art baselines under various non-independently and identically distributed (non-IID) settings. Furthermore, our experiments show that Metalens could be further improved with OOD detection techniques.
更多查看译文
关键词
Federated Meta-Learning,Data Heterogeneity,Ensemble
AI 理解论文
溯源树
样例
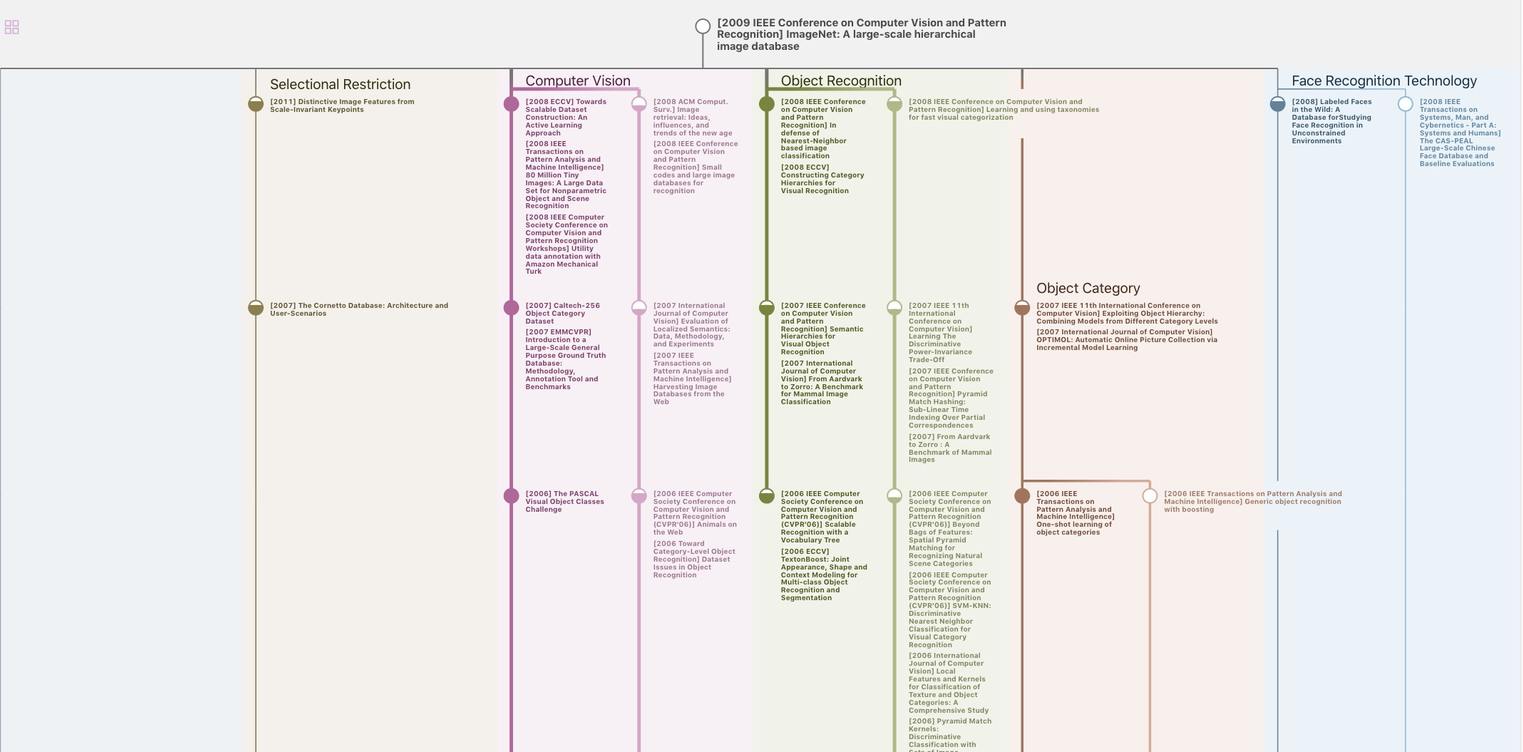
生成溯源树,研究论文发展脉络
Chat Paper
正在生成论文摘要