Tomo2Mesh: Fast Porosity Mapping and Visualization for Synchrotron Tomography
2023 IEEE 19th International Conference on e-Science (e-Science)(2023)
摘要
Applications of X-ray computed tomography (CT) in porosity characterization of engineering materials often involve an extensive data analysis workflow. This workflow includes CT reconstruction of raw projection data, binarization, labeling, and mesh extraction. Mapping porosity in larger samples presents a significant computational challenge, as it requires processing gigabytes of raw data to extract porosity information, which becomes a critical bottleneck in the analysis. In this study, we present algorithms and an implementation of an end-to-end porosity mapping framework. Our framework processes raw projection data obtained from a synchrotron CT instrument, generating a porosity map and a visualization in the form of a triangular face mesh. To achieve this objective, we introduce a novel subset reconstruction scheme for X-ray CT, combining filtered back-projection and a convolutional neural network. This scheme allows us to reconstruct subsets of a tomography object with arbitrary shapes. Building upon this scheme, we have developed a fast and efficient framework for porosity mapping. Initially, our framework detects potential voids by performing a coarse reconstruction on down-sampled projections. Subsequently, we enhance the shape of these voids by reconstructing selected subsets from the original raw data, providing higher detail. To evaluate the performance of our framework, we measured the processing time from raw data to a triangular face mesh across multiple visualization scenarios. Our experiments were conducted on a single high-performance workstation equipped with a GPU. The results demonstrate that our framework enables the visualization of local porosity within an 8-gigavoxel CT volume (12 gigabytes raw data) in just 1 to 2 minutes. Moreover, for a larger 64-gigavoxel CT volume (100 gigabytes of raw data), the visualization can be generated within 3 to 7 minutes, showcasing the efficiency of our approach.
更多查看译文
关键词
X-ray Tomography,Computed Tomography,3D convolutional neural networks
AI 理解论文
溯源树
样例
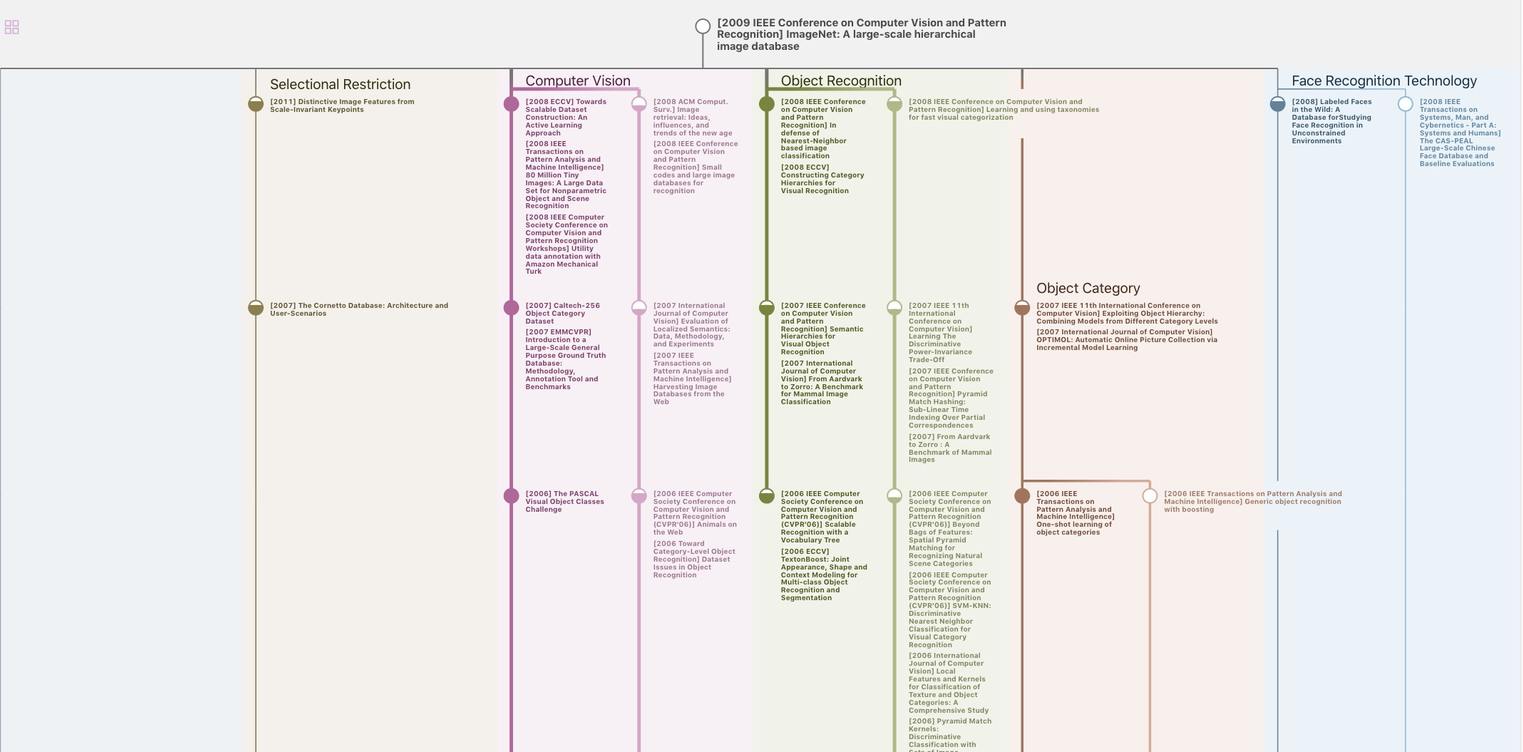
生成溯源树,研究论文发展脉络
Chat Paper
正在生成论文摘要