Improvement of a Rapid Method of Detecting Gasoline Detergency Based on the Image Recognition.
ACS omega(2023)
摘要
The detergency of motor gasoline is closely related to vehicle exhaust emissions and fuel economy. This paper proposed an improved method for the rapid detection of gasoline detergency based on the deposit images of test gasoline on aluminum plates produced by a multichannel gasoline detergency simulation test (MGST). The detection algorithm system was structured to recognize the deposit plate images by computer vision based on the convolutional neural networks (CNNs). Compared with the traditional simulation test, the improved MGST method resulted in significant reductions in fuel consumption, cost, and test time. The performance of three transfer learning models (Inception-ResNet-V2, Inception-V3, and ResNet50-V2) and a customized CNN was evaluated in the detection algorithm system, and their detection accuracies reached 94, 94, 88, and 82%. Inception-RsNet-V2 was selected due to its higher accuracy and better robustness. Based on the model interpretation, it is evident that the model undergoes feature extraction from the sediment deposits on the deposit plate. Subsequently, it employed the acquired deposit features to accurately detect gasoline samples that failed to meet detergency standards. This approach was proved to be effective in enhancing the detection process and ensuring reliable results for gasoline detergency evaluation. It is beneficial to environmental protection regulators for managing market gasoline detergency and urban mobile source pollution. In addition, a deposit plate image database should be established to further improve the detection model performance during the environmental regulation.
更多查看译文
关键词
detecting gasoline detergency,recognition
AI 理解论文
溯源树
样例
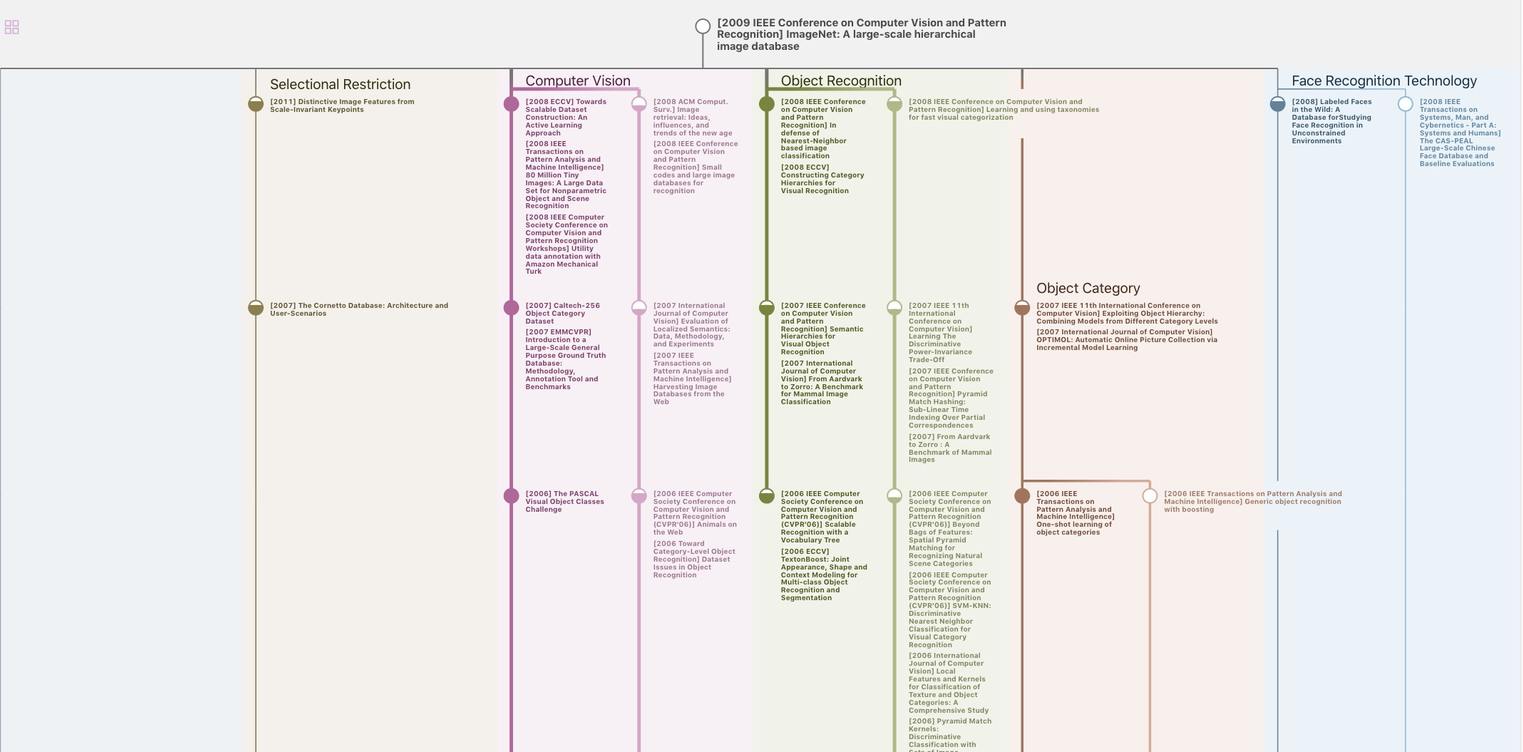
生成溯源树,研究论文发展脉络
Chat Paper
正在生成论文摘要