A Robust and Real-Time Hyper-Spectral Sensor-Fusion Model for Concrete Crack Segmentation
2023 IEEE Sensors Applications Symposium (SAS)(2023)
摘要
Structural defects in civil infrastructure, such as highways, roads, bridges, and dams, can severely impact their reliability and safety. Manual inspection of such infrastructure is labor-intensive and costly, creating the demand for automated damage-tracking systems. Data-driven techniques, such as machine learning, and statistical methods have been utilized in a remarkable number of Structural Health Monitoring (SHM) applications. However, real-time and high-accuracy concrete crack detection poses severe challenges for deployment on embedded systems and mobile robotic platforms, due to their computational constraints, low-latency requirements, and the robustness of model predictions in field deployments. This work proposes a robust and low-latency transformer-based deep-crack segmentation model that leverages both Red Green Blue (RGB) and Hyper Spectral (HYP) data for crack detection. The model is optimized for deployment on resource-constrained embedded systems, enabling structural health monitoring on robotic platforms, and studies the aleatoric and epistemic uncertainties of the estimated crack maps. The final model is deployed on one of the most popular robotic embedded computation platforms such as the NVIDIA Jetson Xavier NX, achieving state-of-the-art performance with an accuracy of 99.54% at an inference time of only 7.41ms using the RGB camera only. The proposed approach provides a promising solution for automating laborious SHM applications such as inspecting critical infrastructure.
更多查看译文
关键词
Smart-Cities,Machine Learning,Structural Health Monitoring
AI 理解论文
溯源树
样例
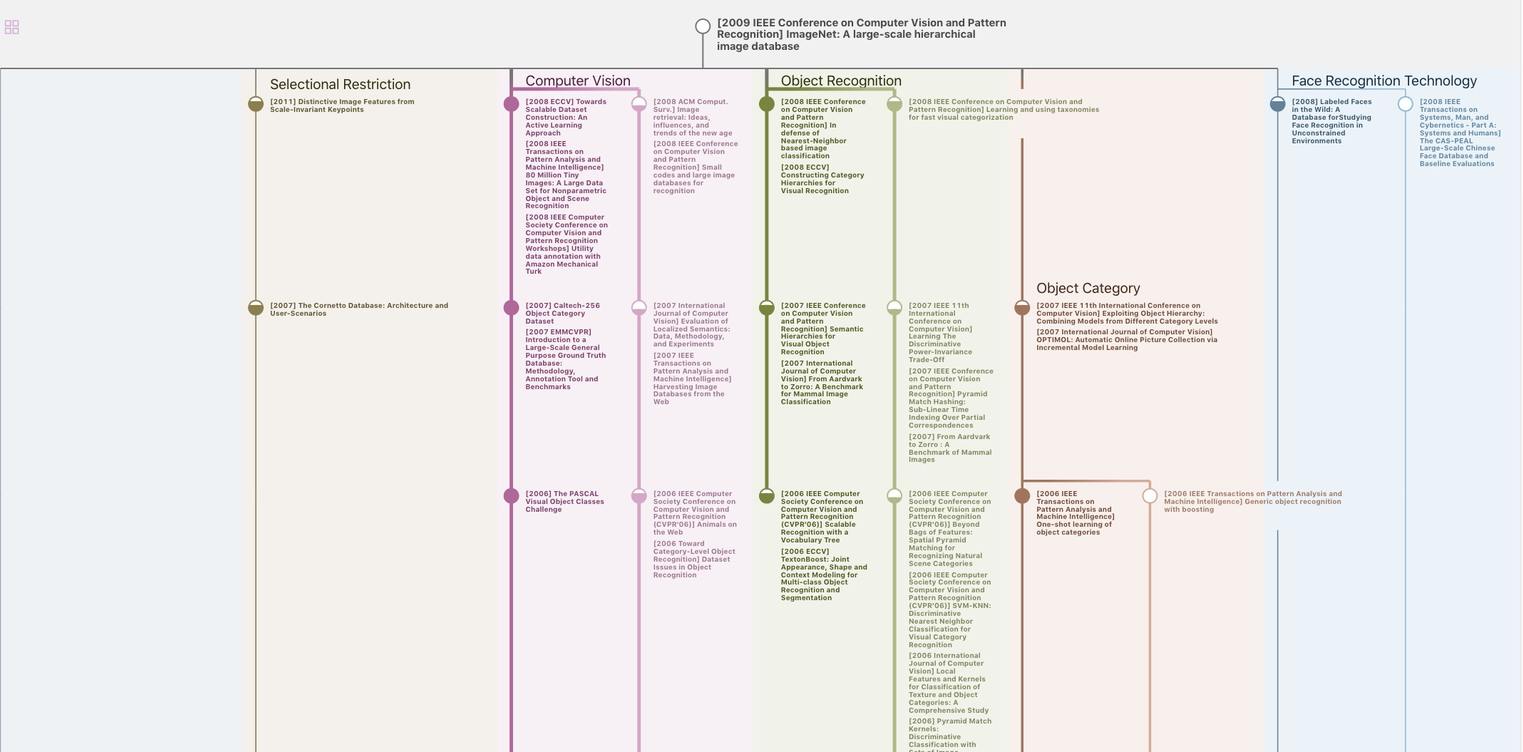
生成溯源树,研究论文发展脉络
Chat Paper
正在生成论文摘要