Gait Recognition Using EigenfeetNet
2023 IEEE Sensors Applications Symposium (SAS)(2023)
摘要
Due to a growing emphasis on personal privacy and non-intrusive methods of person authentication, researchers have sought to evaluate new and emerging biometric technologies. One promising approach is gait recognition using pressure-sensitive flooring (or footstep recognition) which strives to verify a person's identity using the patterns of pressures exerted on the floor while they walk. In this study, we describe the development of a solution for person verification based on a fused feature selection process inspired by the popular PCA-based eigenfaces approach and a deep learning framework. Dynamic three-dimensional (3D) foot pressure patterns recording during walking were first reduced to ten different 2D pre-feature images. Using the eigenfeet extracted from the peak pressure, a nearest neighbour balanced accuracy (BACC) of 91.1% was obtained based on a single footstep when verifying subjects. Selecting discriminatory eigenfeet, using a minimum-redundancy-maximum-relevance (mRMR), further improved the performance (93.4% BACC), and when fused with a convolutional neural network (CNN) architecture into a stacking PCA network (PCANet+), the maximum verification performance of 96.2% BACC was found. These results show that the proposed selective EigenfeetNet method (i.e., peak pressure, PCANet+, and mRMR) provides a promising platform for the further development of floor sensor-based gait recognition for person verification.
更多查看译文
关键词
biometric,convolutional neural network,CNN,deep learning,eigenface,eigenfeet,gait recognition,principal component analysis,PCA,plantar pressure
AI 理解论文
溯源树
样例
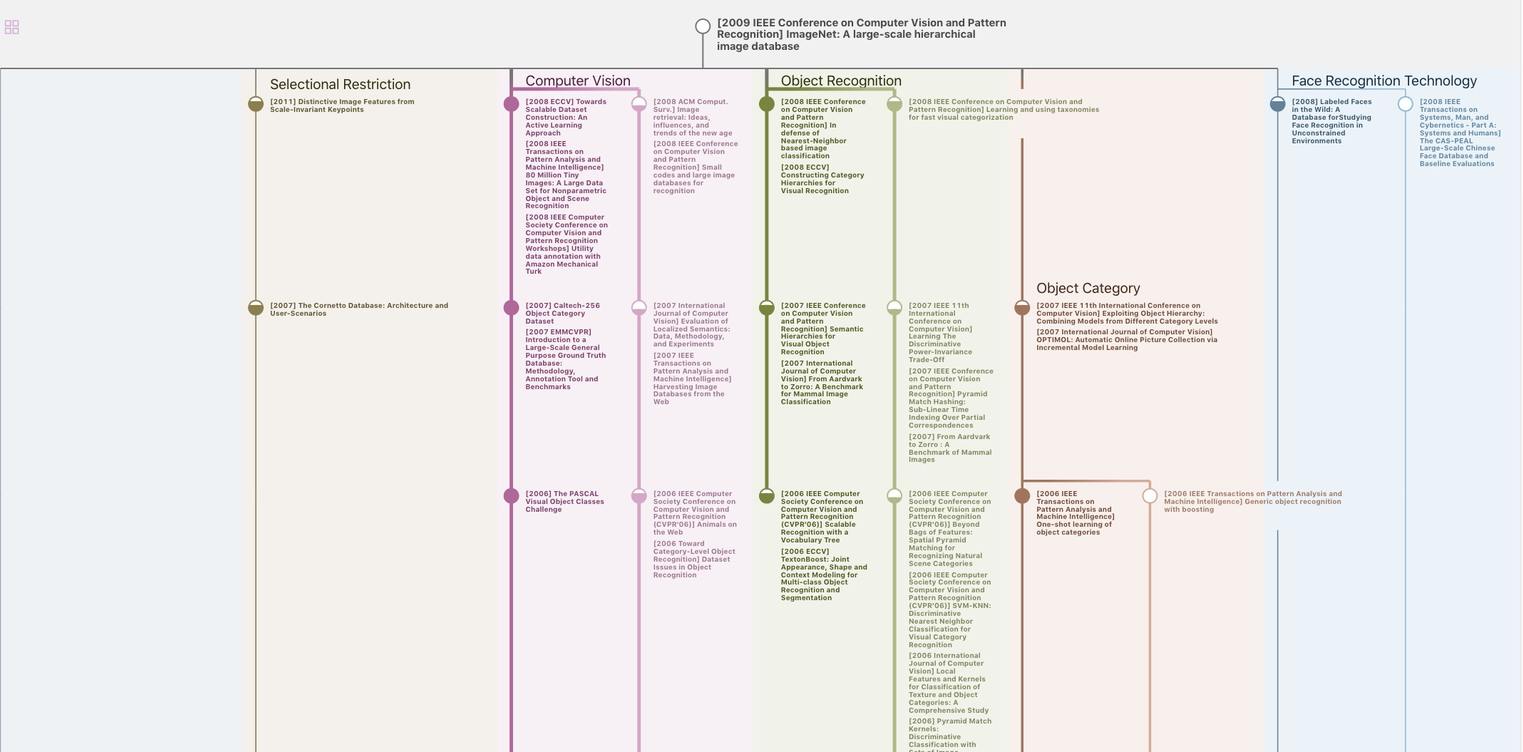
生成溯源树,研究论文发展脉络
Chat Paper
正在生成论文摘要