FDPR: A Novel Fog Data Prediction and Recovery Using Efficient DL in IoT Networks
IEEE Internet of Things Journal(2023)
摘要
The goal of this study is to offer a novel fog data prediction and recovery (FDPR) algorithm that uses deep learning (DL) to forecast and recover missing sensor data in an Internet of Things (IoT) network. Because of the fog layer’s unique qualities compared to other IoT environment layers, the FDPR algorithm is employed in this layer. The most recent studies generally concentrate on data recovery or prediction, with few assessment metrics. In this work, an algorithm that can handle both data prediction and recovery is provided. With the proposed FDPR approach, data prediction and recovery are dealt with by an effective DL network, namely, a deep concatenated multilayer perceptron (DC-MLP). The algorithm consists of a prediction function that forecasts future sensor data for a specified round of data transmission and a recovery function that recover one or two missing data points. The evaluation of the proposed algorithm is performed with simulation and experimental works. Initially, a data set is collected, preprocessed, and fed to various DL models using
$K$
-fold cross-validation. These DL models are then converted and embedded into a fog layer in the experimental work with nine edge devices. In both simulation and experimental evaluation, the FDPR with DC-MLP can predict future data and recover missing data with an average accuracy of 99.89% while slightly increasing network delay by 2.5 ms compared to traditional IoT. Aside from a slightly increased delay, a 121% improvement in IoT device lifetime is achieved using the FDPR algorithm due to data transmission reduction.
更多查看译文
关键词
novel fog data prediction,iot,efficient dl
AI 理解论文
溯源树
样例
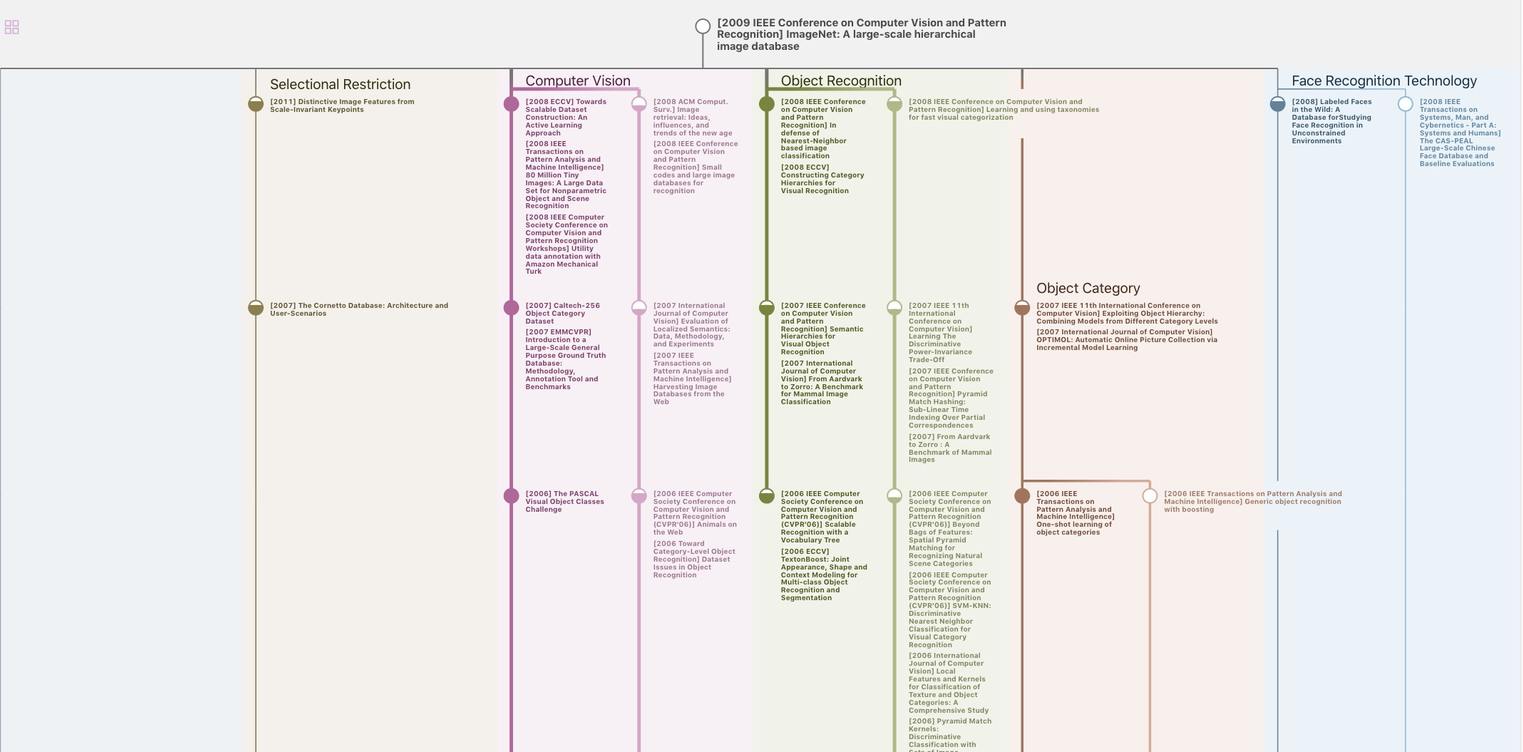
生成溯源树,研究论文发展脉络
Chat Paper
正在生成论文摘要