A dynamic mean-field statistical model of academic collaboration
arXiv (Cornell University)(2023)
摘要
There is empirical evidence that collaboration in academia has increased significantly during the past few decades, perhaps due to the breathtaking advancements in communication and technology during this period. Multi-author articles have become more frequent than single-author ones. Interdisciplinary collaboration is also on the rise. Although there have been several studies on the dynamical aspects of collaboration networks, systematic statistical models which theoretically explain various empirically observed features of such networks have been lacking. In this work, we propose a dynamic mean-field model and an associated estimation framework for academic collaboration networks. We primarily focus on how the degree of collaboration of a typical author, rather than the local structure of her collaboration network, changes over time. We consider several popular indices of collaboration from the literature and study their dynamics under the proposed model. In particular, we obtain exact formulae for the expectations and temporal rates of change of these indices. Through extensive simulation experiments, we demonstrate that the proposed model has enough flexibility to capture various phenomena characteristic of real-world collaboration networks. Using metadata on papers from the arXiv repository, we empirically study the mean-field collaboration dynamics in disciplines such as Computer Science, Mathematics and Physics.
更多查看译文
关键词
collaboration,statistical model,mean-field
AI 理解论文
溯源树
样例
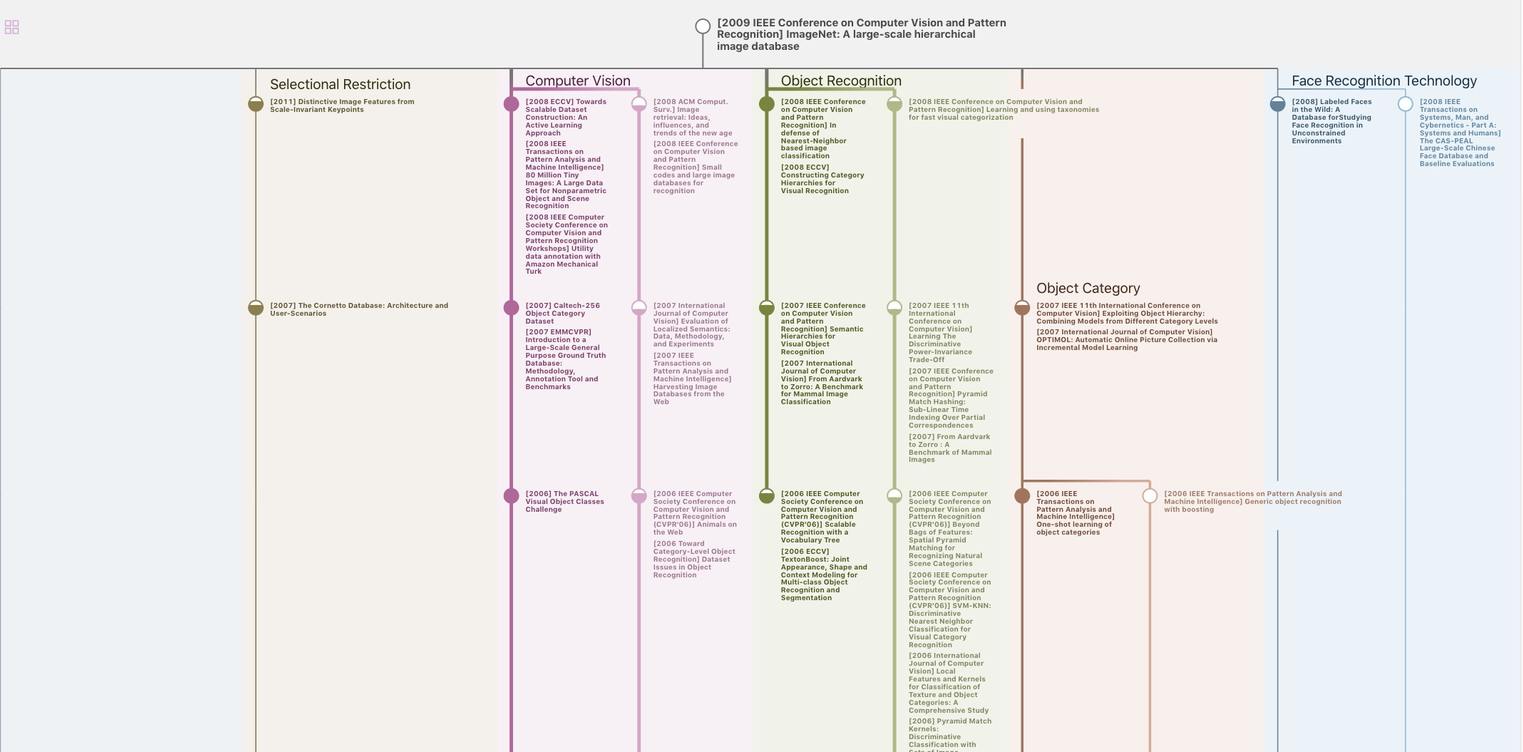
生成溯源树,研究论文发展脉络
Chat Paper
正在生成论文摘要