Enabling DVFS Side-Channel Attacks for Neural Network Fingerprinting in Edge Inference Services
2023 IEEE/ACM International Symposium on Low Power Electronics and Design (ISLPED)(2023)
摘要
The Inference-as-a-Service (IaaS) delivery model provides users access to pre-trained deep neural networks while safeguarding network code and weights. However, IaaS is not immune to security threats, like side-channel attacks (SCAs), that exploit unintended information leakage from the physical characteristics of the target device. Exposure to such threats grows when IaaS is deployed on distributed computing nodes at the edge. This work identifies a potential vulnerability of low-power CPUs that facilitates stealing the deep neural network architecture without physical access to the hardware or interference with the execution flow. Our approach relies on a Dynamic Voltage and Frequency Scaling (DVFS) side-channel attack, which monitors the CPU frequency state during the inference stages. Specifically, we introduce a dedicated load-testing methodology that imprints distinguishable signatures of the network on the frequency traces. A machine learning classifier is then used to infer the victim architecture. Experimental results on two commercial ARM Cortex-A CPUs, the A72 and A57, demonstrate the attack can identify the target architecture from a pool of 12 convolutional neural networks with an average accuracy of 98.7% and 92.4%
更多查看译文
关键词
Inference-as-a-Service,Convolutional Neural Networks,Side-Channel Attacks,DVFS
AI 理解论文
溯源树
样例
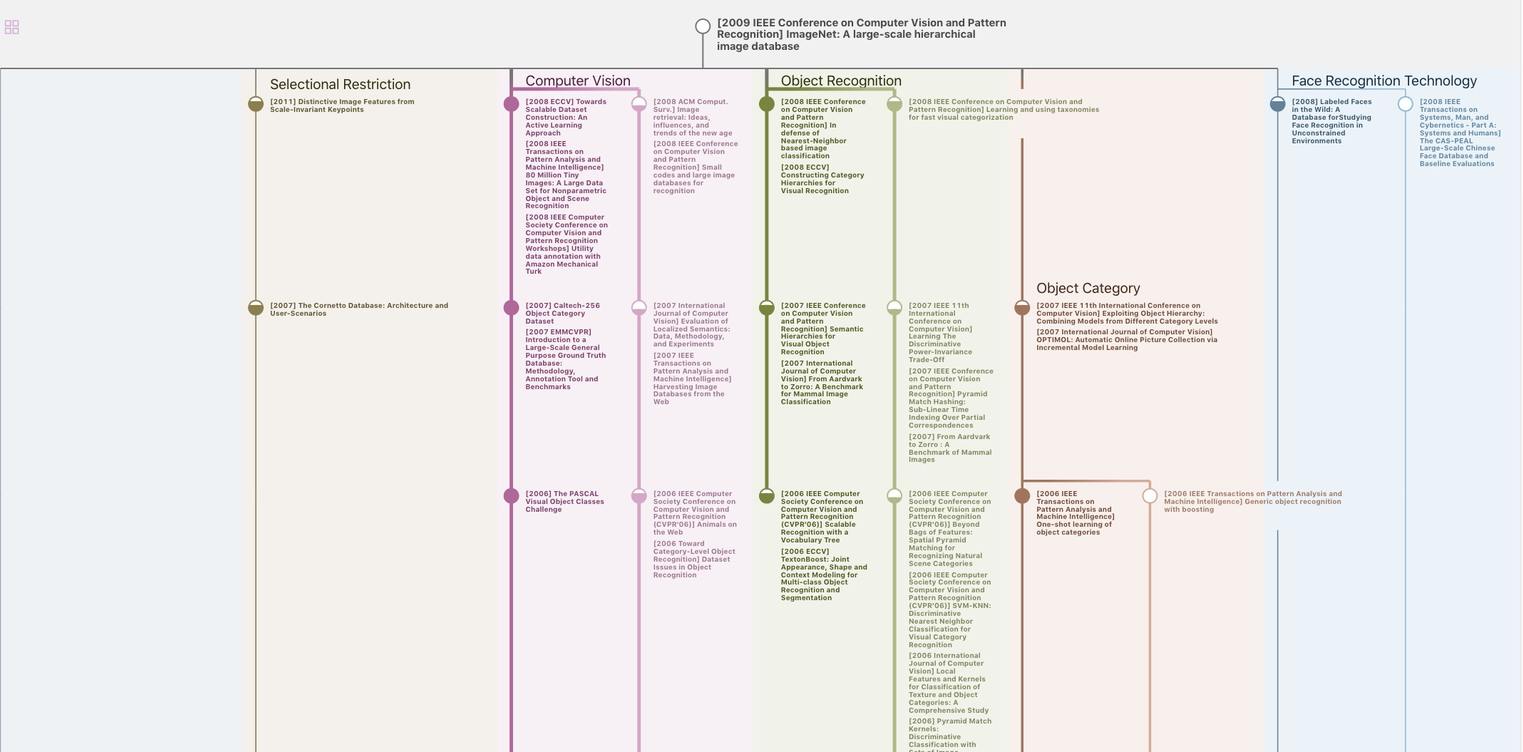
生成溯源树,研究论文发展脉络
Chat Paper
正在生成论文摘要