Fed4ReID: Federated Learning with Data Augmentation for Person Re-identification Service in Edge Computing
2023 IEEE International Conference on Web Services (ICWS)(2023)
摘要
Federated learning is a new distributed privacy-preserving learning paradigm which perfectly meets the requirements of many large service systems such as banking, healthcare, and smart city. Meanwhile, person re-identification, as a technology to associate the images of the same person from different data sources, has been widely used in many smart services such as smart logistics, smart surveillance, and many public searching and rescue missions. Therefore, it is a promising solution to use federated learning for person re-identification to improve the model accuracy while protecting the data privacy. However, the common problem of non-independent and identically distributed (Non-IID) data with heterogeneous clients in federated learning often causes undesirable model accuracy. To address such a problem, in this paper, we propose a novel strategy named federated learning with data augmentation for person re-identification (Fed4ReID). Specifically, to alleviate the impact of Non-IID data, we utilise a pre-trained DCGAN (Deep Convolutional Generative Adversarial Network) model for data augmentation at each edge servers. Experiments on public datasets show that our proposed strategy can outperform baseline method in general accuracy.
更多查看译文
关键词
Federated learning,Edge computing,DCGAN,Non-IID,Person re-identification
AI 理解论文
溯源树
样例
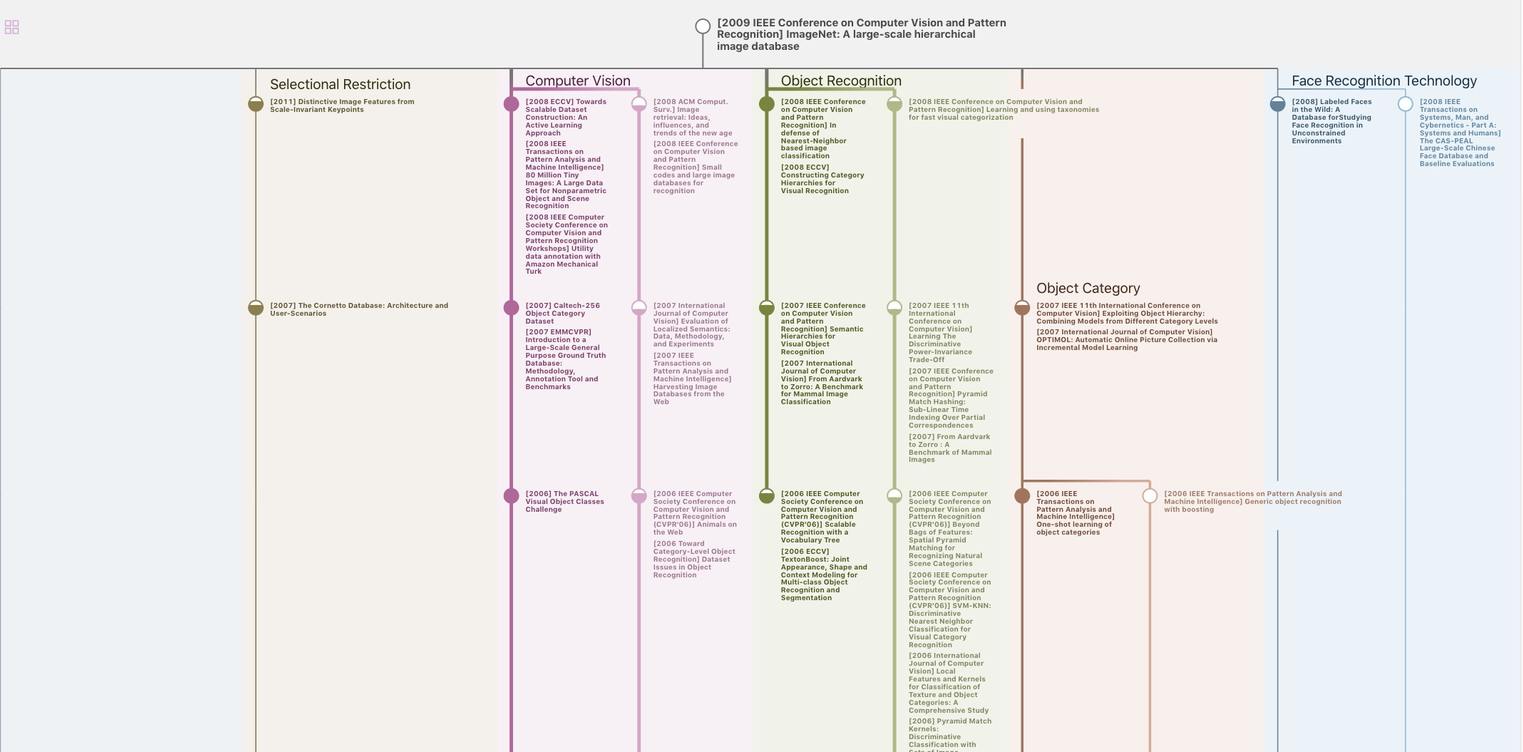
生成溯源树,研究论文发展脉络
Chat Paper
正在生成论文摘要