Exploring and validating the prognostic value of pathomics signatures and genomics in patients with cutaneous melanoma based on bioinformatics and deep learning.
Medical physics(2023)
摘要
The clinical traits for 468 CM samples and the genomic data and pathology images for 471 CM samples were obtained from the TCGA database. The EDA results combined with multiple machine learning (ML) models suggested that the top 5 clinical traits in terms of importance were age, biopsy site, T stage, N stage and overall disease stage, and the eight ML models had a precision lower than 0.56. A total of 60 differentially expressed genes were obtained by comparing sequencing data. A total of 413 available quantitative signatures of each pathomics image were obtained with CellProfile software. The precision of the binary classification model based on pathomics signatures was 0.99, with a loss value of 1.7119e-04. The precision of the binary classification model based on differentially expressed genes was 0.98, with a loss value of 0.1101. The precision of the binary classification model based on pathomics signatures and differentially expressed genes was 0.97, with a loss value of 0.2088. The survival analyses showed that the survival rate of the high-risk group based on gene expression and pathomics signatures was significantly lower than that of the low-risk group. A total of 222 pathomics signatures and 51 differentially expressed genes were analyzed for survival with p-values of less than 0.05. There was a certain correlation between some pathomics signatures and differential gene expression involving ANO2, LINC00158, NDNF, ADAMTS15, and ADGRB3, etc. CONCLUSION: This study evaluated the prognostic significance of pathomics signatures and differentially expressed genes in CM patients. Three ANN models were developed, and all achieved accuracy rates higher than 97%. Specifically, the pathomics signature-based ANN model maintained a remarkable accuracy of 99%. These findings highlight the CellProfile + ANN model as an excellent choice for prognostic prediction in CM patients. Furthermore, our correlation analysis experimentally demonstrated a preliminary link between disease quantification and qualitative changes. Among various features, including M stage and treatments received, special attention should be given to age, biopsy site, T stage, N stage, and overall disease stage in CM patients.
更多查看译文
AI 理解论文
溯源树
样例
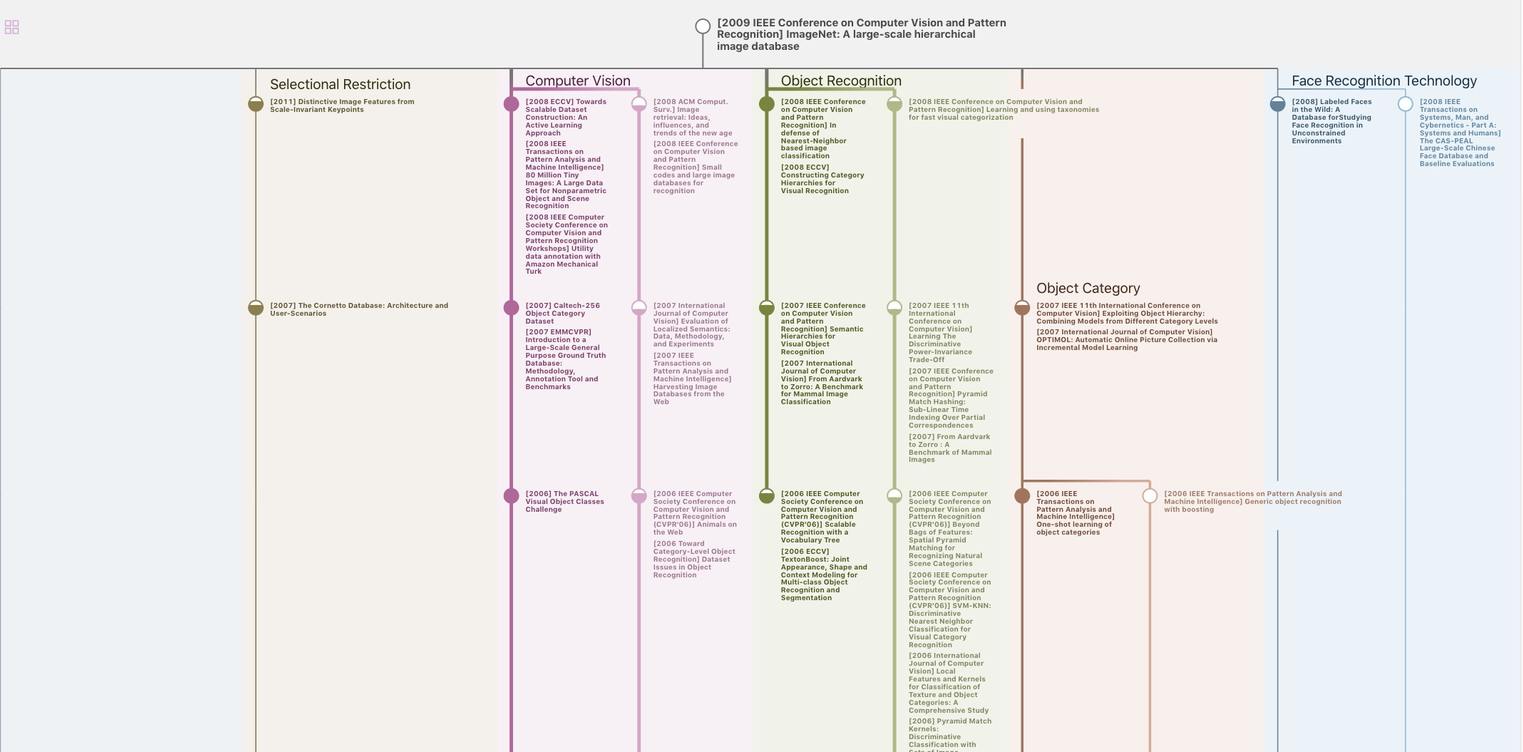
生成溯源树,研究论文发展脉络
Chat Paper
正在生成论文摘要