Compatible intent-based interest modeling for personalized recommendation
Applied Intelligence(2023)
摘要
The conventional uniform embeddings lack diversity to infer users’ interests and make suboptimal recommendations for users. Fortunately, users’ interactions imply a complex and hybrid composition of users’ interests with multiple compatible intents. Therefore, this work strives to investigate fine-grained interest modeling from the diversified composition of interest with the intent hypothesis. We propose a cross-intent transformer embedding (CITE) for personalized recommendation, which extracts collaborative filtering (CF) signals by propagating interests within intent subgraphs and between compatible intents. In the scenario of interaction sparsity, intent-aware interest propagation employs graph convolution to ensure interest consistency in each intent subgraph. It builds intent-aware embeddings with interaction confidences learned iteratively on each intent subgraph. In addition, the transformer evaluates inter-intent compatibility to perform cross-intent interest propagation. It updates intent embeddings with CF signals between intents. The resulting multiple fine-grained intent embeddings model the hybrid composition of users’ interests for personalized recommendation. Extensive experiments on three real-world datasets demonstrate the effectiveness of the proposed CITE and verify the active role of the compatible intents for interest modeling.
更多查看译文
关键词
Collaborative filtering,Personalized recommendation,User interest,Embedding learning
AI 理解论文
溯源树
样例
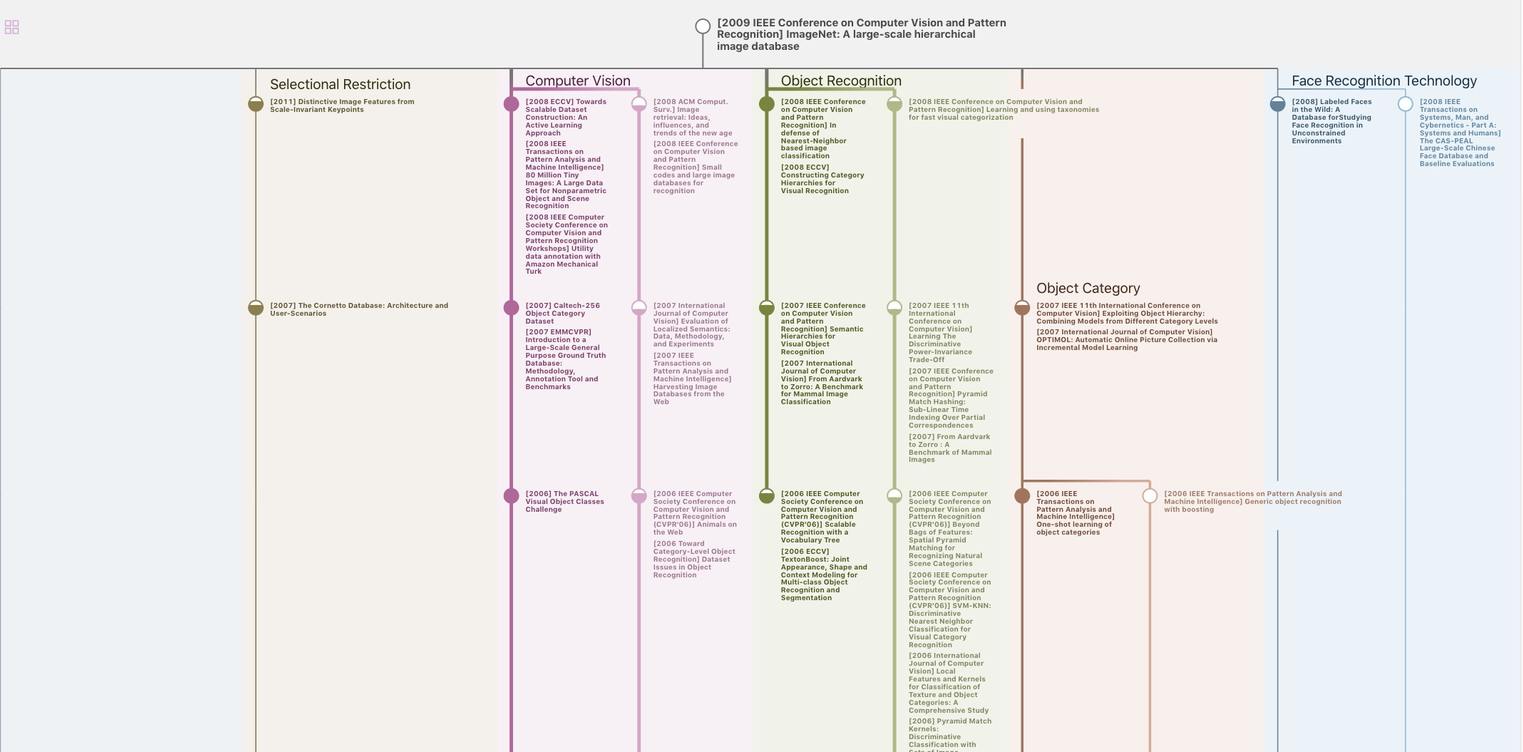
生成溯源树,研究论文发展脉络
Chat Paper
正在生成论文摘要