Cost-Sensitive Online Adaptive Kernel Learning for Large-Scale Imbalanced Classification
IEEE Transactions on Knowledge and Data Engineering(2023)
摘要
Imbalanced classification is a challenging task in the fields of machine learning, data mining and pattern recognition. Cost-sensitive online algorithms are very important methods for large-scale imbalanced classification problems. At present, most of the cost-sensitive classification algorithms focus on the accuracy of the minority class and ignore the accuracy of the majority class. In order to better balance the accuracy between the minority class and the majority class, in this article, a misclassification cost is presented to ensure that the cost-sensitive online algorithm can better deal with the imbalanced classification problems without signifificantly reducing the accuracy of the majority class. Based on the proposed misclassification cost, a novel cost-sensitive online adaptive kernel learning algorithm is proposed to boost the adaptability of kernel function when data arrives one by one. According to the essential characteristics of the imbalanced binary classification, a cost-sensitive online adaptive kernel learning algorithm is given to handle the large-scale imbalanced multi-class classification problems. Theoretical analysis of the proposed algorithms are provided. Extensive experiments demonstrate that compared with the state-of-the-art imbalanced classification algorithms, the proposed algorithms can significantly improve the classification performances on most of the large-scale imbalanced data sets.
更多查看译文
关键词
Adaptive algorithms,cost function,classification algorithms,kernel,machine learning algorithms
AI 理解论文
溯源树
样例
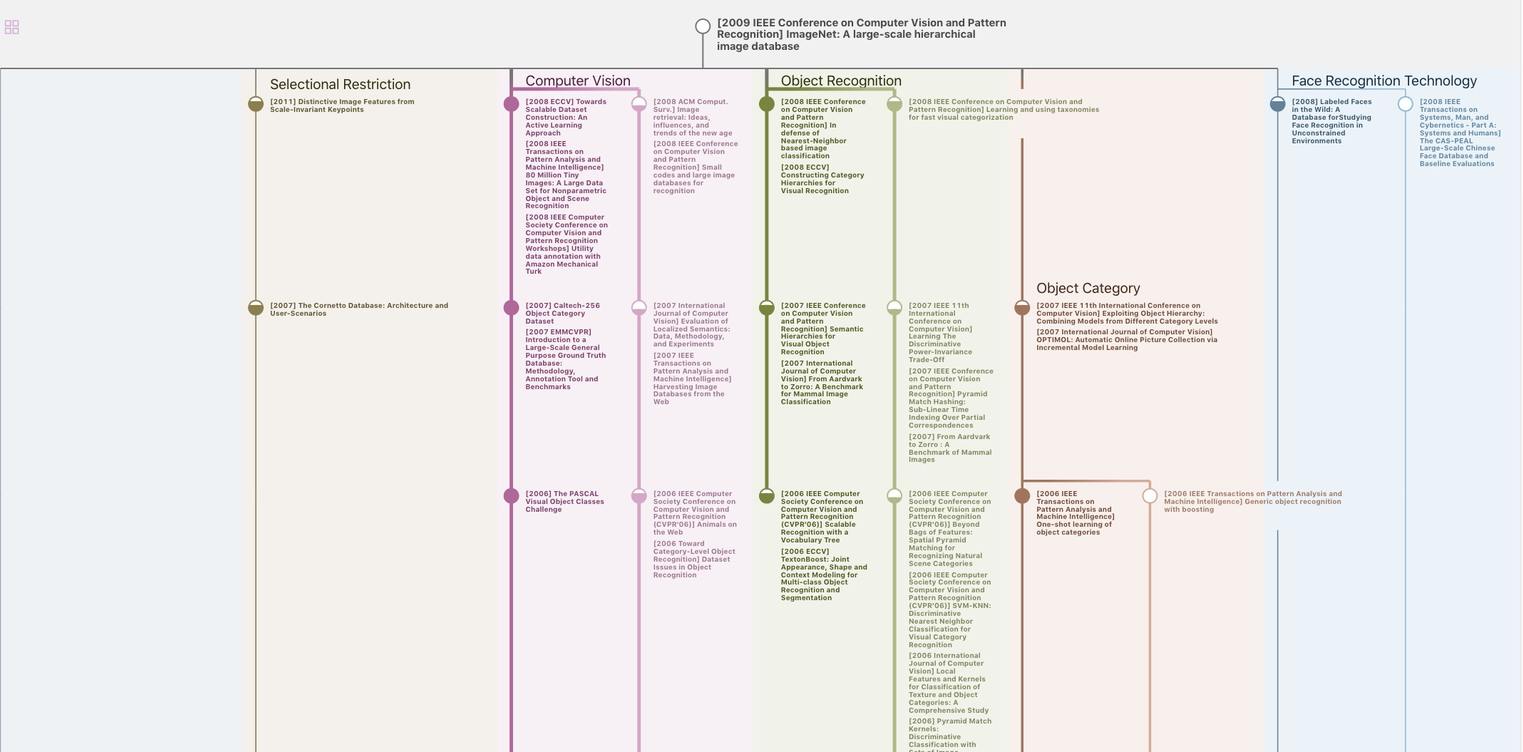
生成溯源树,研究论文发展脉络
Chat Paper
正在生成论文摘要