VDU: VMS Dataset Upsampling via Multi-Source Data Fusion for Trawlers
OCEANS 2023 - Limerick(2023)
摘要
Vessel Monitoring Systems (VMS) equipped on trawlers has recorded a large amount of trajectory data. However, due to the EU standard of sampling rate being two hours, many VMS datasets face the problem of low sampling rates, which hinders the analysis of fishing effort distributions. Previous studies apply mathematical and deep learning methods as interpolation methods to upsample the VMS dataset. However, these methods always lose precision when the sampling rate is required from two hours to six minutes because most movement features will be lost in two hours. This paper proposes a novel VMS Dataset Upsampling (VDU) method via multi-source data fusion for trawlers, which incorporates additional marine environmental factors (MEF) to help the model capture the movement features. VDU is designed on deep learning, including Multi-Layer Perceptron (MLP), Residual Network (ResNet), and Long Short-Term Memory (LSTM) neural network. MLP is used to extract VMS record features, ResNet is employed for MEF data, and LSTM is applied to generate the high sampling rate records. By applying the VMS records of 1855 trawlers in the East China Sea and the MEF in the corresponding area as a case study, VDU increases the sampling rate from two hours to six minutes with a low average interpolation error of 0.77 km. We also conduct an extensive study to verify the efficacy of multi-source data fusion for interpolation.
更多查看译文
AI 理解论文
溯源树
样例
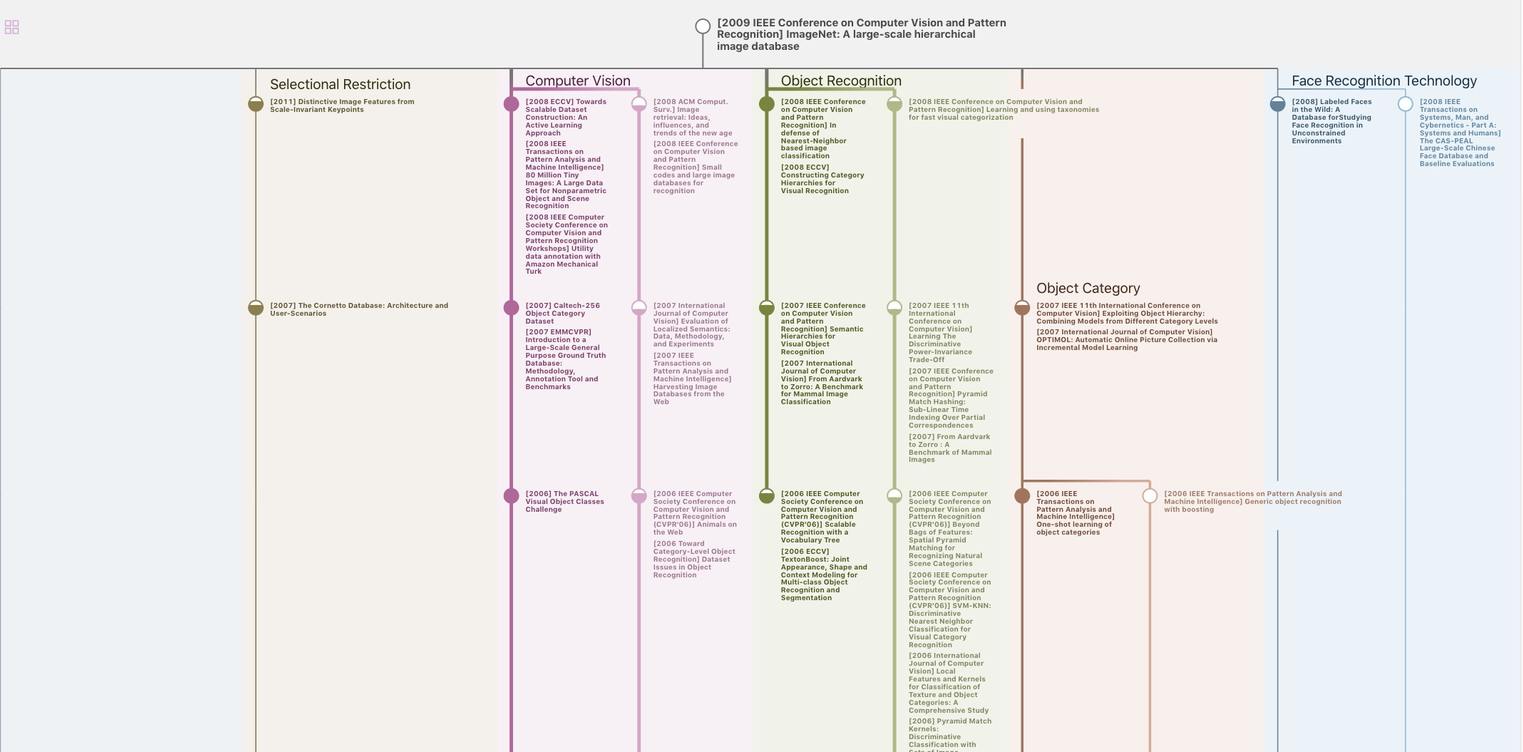
生成溯源树,研究论文发展脉络
Chat Paper
正在生成论文摘要