EAD-YOLO: Improved YOLOv5 for Endoscopic Artefact Detection
2023 International Conference on Communications, Computing and Artificial Intelligence (CCCAI)(2023)
摘要
Endoscopic artefacts seriously affect the visualization of the lesion area during endoscopy and the results of automated detection and analysis. Therefore, accurate detection of clinical endoscopic artefacts has become a key bottleneck. In this paper, aiming at the problems of data imbalance and severe multi-scale distribution of objects in the endoscope artefact dataset, we propose a multi-scale detection algorithm EADYOLO. Based on the baseline network YOLOv5, the algorithm has been improved in three aspects. First, the data imbalance is alleviated by adopting data augmentation methods. Second, by constructing a multi-scale detection layer to improve the detection effect of the algorithm on endoscopic artefact targets of different scales. The depthwise separable convolution is used to reduce the number of parameters of the network and improve the feature extraction capability of the backbone network. Finally, Atrous Spatial Pyramid Pooling (ASPP) module is used to further enhance the expression ability of multi-scale target features. Compared with the baseline network, EAD-YOLO can improve the detection accuracy and recall rate of small and large targets by 3.23%, 7.64%, 2.37%, and 4.45% respectively. The comparison with classical and effective algorithms further proves that EAD-YOLO can better adapt to the severe size changes of endoscopic artefacts, and is more beneficial to the task of endoscopic artefact target detection.
更多查看译文
关键词
multi-scale object detection,endoscopy artefact detection,improved YOLOv5,data imbalance,enhanced backbone network,multi-scale feature construction
AI 理解论文
溯源树
样例
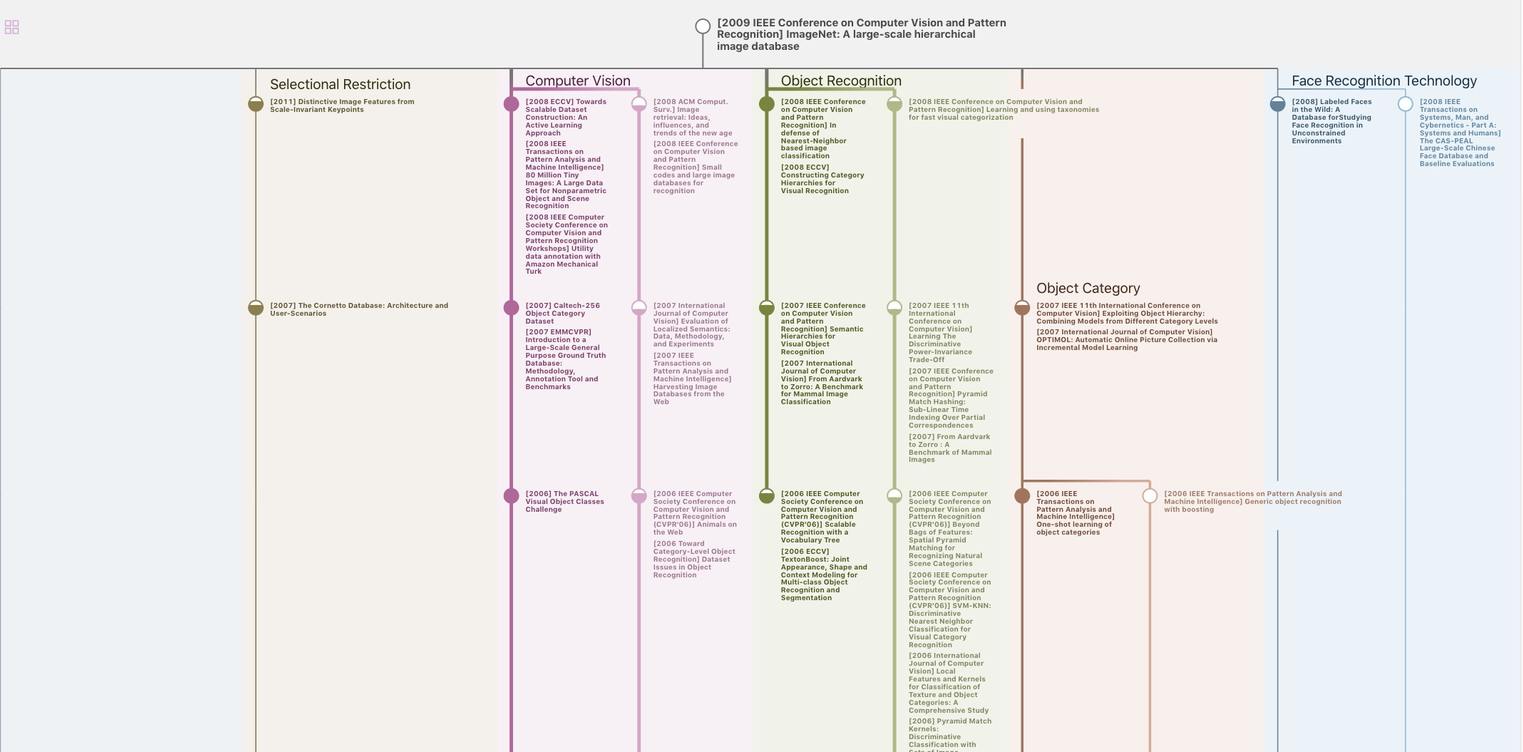
生成溯源树,研究论文发展脉络
Chat Paper
正在生成论文摘要