Classification of Attention Deficit/Hyperactivity Disorder based on EEG signals using a EEG-Transformer model.
Journal of neural engineering(2023)
摘要
Attention-deficit/hyperactivity disorder (ADHD) is the most common neurodevelopmental disorder in adolescents that can seriously impair a person's attention function, cognitive processes, and learning ability. Currently, clinicians primarily diagnose patients based on the subjective assessments of the Diagnostic and Statistical Manual of Mental Disorders (DMS-5), which can lead to delayed diagnosis of ADHD and even misdiagnosis due to low diagnostic efficiency and lack of well-trained diagnostic experts. Deep learning of electroencephalogram (EEG) signals recorded from ADHD patients could provide an objective and accurate method to assist physicians in clinical diagnosis. This paper proposes the EEG-Transformer deep learning model, which is based on the attention mechanism in the traditional Transformer model, and can perform feature extraction and signal classification processing for the characteristics of EEG signals. A comprehensive comparison was made between the proposed transformer model and three existing convolutional neural network models. The results showed that the proposed EEG-Transformer model achieved an average accuracy of 95.85% and an average AUC value of 0.9926 with the fastest convergence speed, outperforming the other three models. The function and relationship of each module of the module are studied by ablation experiments. The model with optimal performance was identified by the optimization experiment. The EEG-Transformer model proposed in this paper can be used as an auxiliary tool for clinical diagnosis of ADHD, and at the same time provides a basic model for transferable learning in the field of EEG signal classification.
更多查看译文
关键词
attention deficit/hyperactivity deficit/hyperactivity disorder,eeg signals,deficit/hyperactivity disorder,attention deficit/hyperactivity,eeg-transformer
AI 理解论文
溯源树
样例
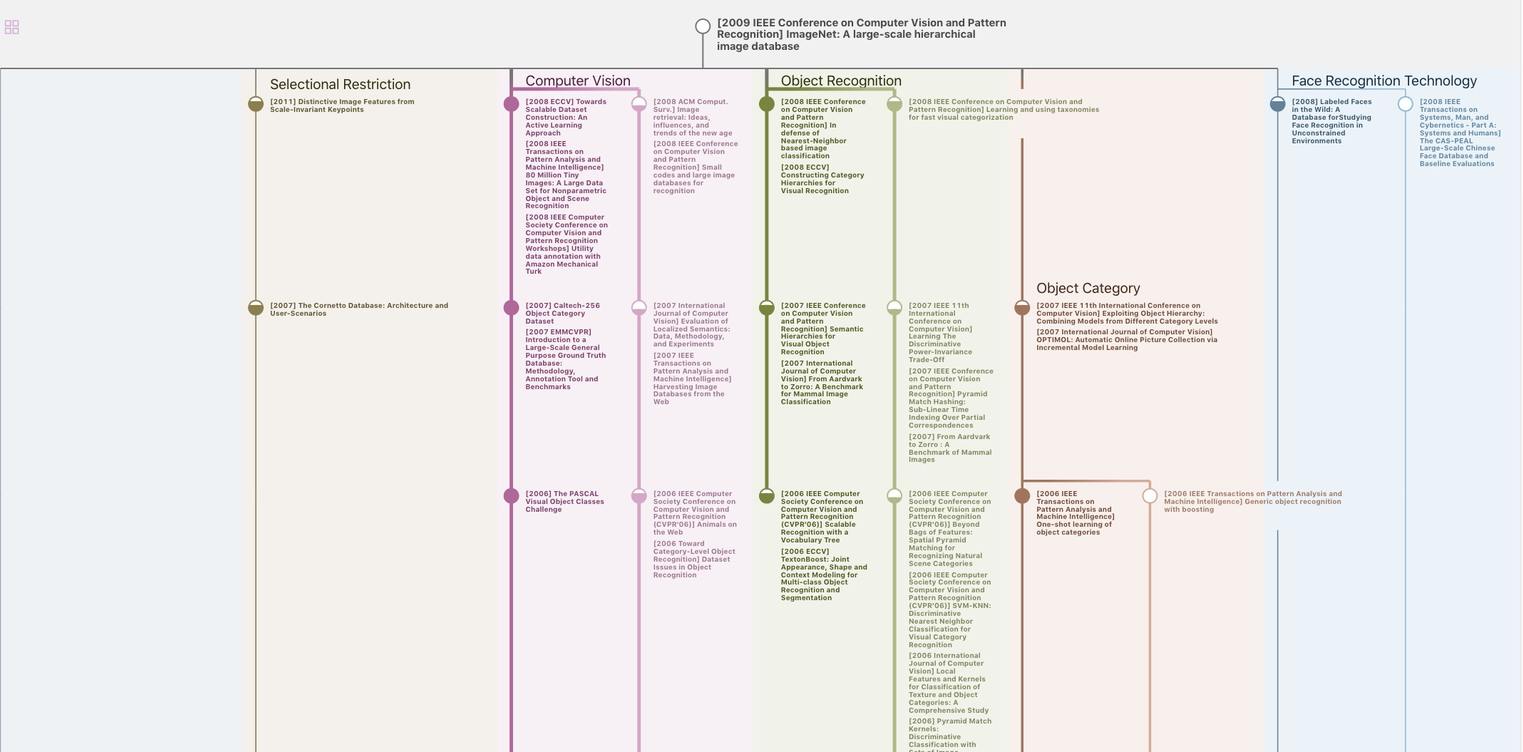
生成溯源树,研究论文发展脉络
Chat Paper
正在生成论文摘要