Interpretable XGBoost-SHAP Model Predicts Nanoparticles Delivery Efficiency Based on Tumor Genomic Mutations and Nanoparticle Properties.
ACS applied bio materials(2023)
摘要
Understanding the complex interaction between nanoparticles (NPs) and tumors in vivo and how it dominates the delivery efficiency of NPs is critical for the translation of nanomedicine. Herein, we proposed an interpretable XGBoost-SHAP model by integrating the information on NPs physicochemical properties and tumor genomic profile to predict the delivery efficiency. The correlation coefficients were 0.66, 0.75, and 0.54 for the prediction of maximum delivery efficiency, delivery efficiency at 24 and 168 h postinjection for test sets. The analysis of the feature importance revealed that the tumor genomic mutations and their interaction with NPs properties played important roles in the delivery of NPs. The biological pathways of the NP-delivery-related genes were further explored through gene ontology enrichment analysis. Our work provides a pipeline to predict and explain the delivery efficiency of NPs to heterogeneous tumors and highlights the power of simultaneously using omics data and interpretable machine learning algorithms for discovering interactions between NPs and individual tumors, which is important for the development of personalized precision nanomedicine.
更多查看译文
关键词
nanoparticles,tumor genomic mutations,xgboost-shap
AI 理解论文
溯源树
样例
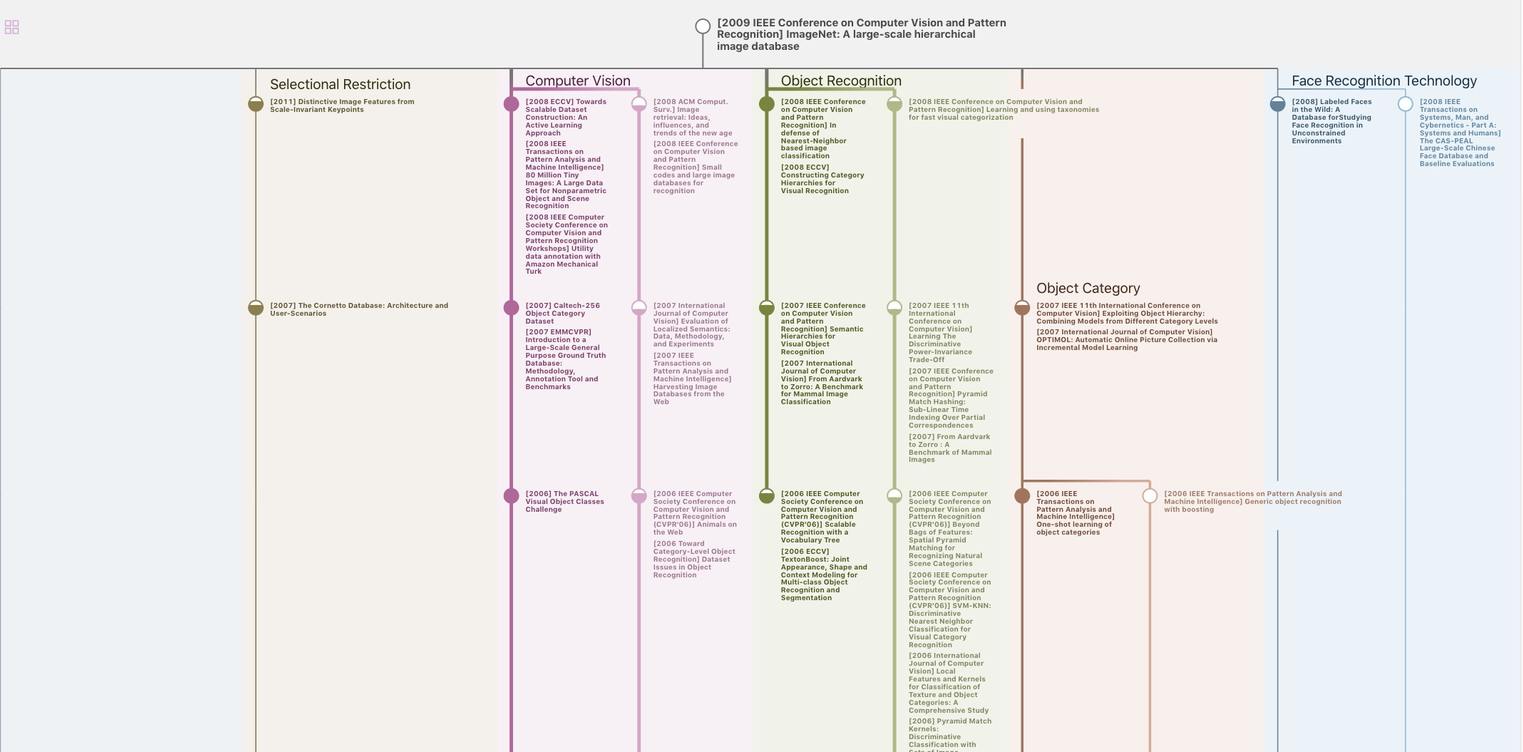
生成溯源树,研究论文发展脉络
Chat Paper
正在生成论文摘要