Efficient and Robust KPI Outlier Detection for Large-Scale Datacenters
IEEE Transactions on Computers(2023)
摘要
To ensure the performance of large-scale datacenters, operators need to monitor up to tens of millions of various-type KPIs, e.g., CPU utilization, memory utilization. For each KPI, it is crucial but challenging to detect outliers that deviate from its historical patterns or the patterns of other KPIs in the same period. In this work, we propose
OutSpot
, an unsupervised outlier detection framework that integrates hierarchical agglomerative clustering (HAC) with conditional variational autoencoder (CVAE), which significantly improves computational efficiency and comprehensively learns the above two patterns. Additionally, two simple yet effective techniques, soft threshold and median filter, are applied to precisely determine outlier KPIs. Using two real-world datasets collected from the datacenters owned by a top-tier global short video service provider and a top-tier domestic operator,respectively. It demonstrates that
OutSpot
achieves the best F1 score of 0.95 and 0.91, AUC of 0.99 and 0.99 on the two datasets, significantly outperforming seven baseline outlier detection methods.
更多查看译文
关键词
robust kpi outlier detection,large-scale
AI 理解论文
溯源树
样例
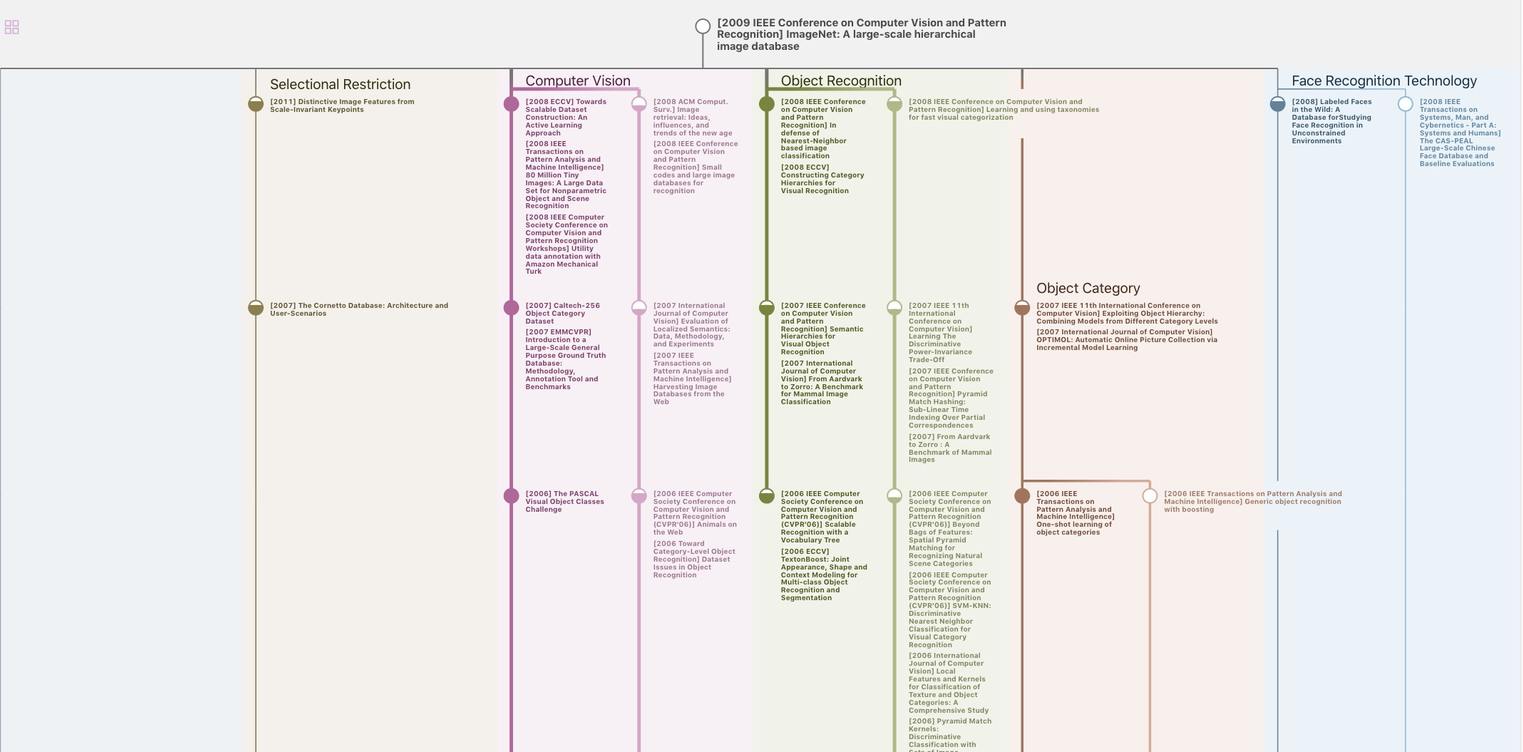
生成溯源树,研究论文发展脉络
Chat Paper
正在生成论文摘要