Double constrained discriminative least squares regression for image classification
Information Sciences(2023)
摘要
Discriminative Least Squares Regression (DLSR) is an approach that is more popularly used in multi-classification tasks, which employs an ε-draggings technique to enhance intra-class similarity. However, this approach fails to take into account the fact that the increased intra-class compactness may also widen the distance between similar classes. For addressing this problem, we propose a novel method called Double Constrained Discriminative Least Squares Regression (DCDLSR), which incorporates both inter- and intra-class distances to improve classification performance. First, the constrained relaxation labels of Fisher criterion are introduced into the DLSR model, which shortens the interval of similar samples. Second, we place the operation of matrix decomposition inside the label space to preserve as much of its manifold structure as possible. Additionally, we introduce l2,1-norm constraints to the noise matrix to mitigate the impact of outliers. Our experiments demonstrate that improving the double constraint and matrix decomposition significantly overcomes the limitations of DLSR and achieves better performance on multi-classification tasks.
更多查看译文
关键词
double constrained discriminative,image classification,regression
AI 理解论文
溯源树
样例
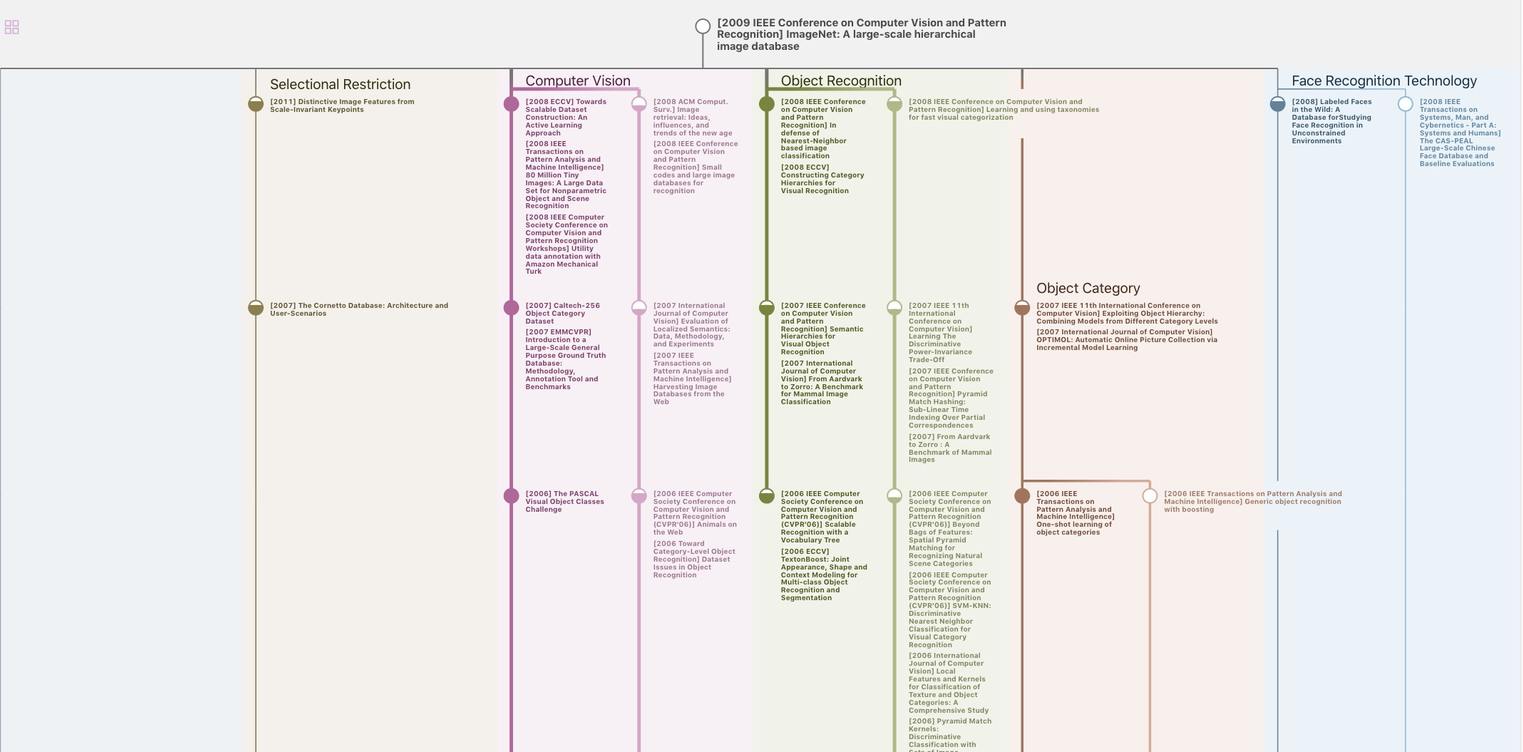
生成溯源树,研究论文发展脉络
Chat Paper
正在生成论文摘要