Few-shot entity linking of food names
Information Processing & Management(2023)
摘要
Entity linking (EL), the task of automatically matching mentions in text to concepts in a target knowledge base, remains under-explored when it comes to the food domain, despite its many potential applications, e.g., finding the nutritional value of ingredients in databases. In this paper, we describe the creation of new resources supporting the development of EL methods applied to the food domain: the E.Care Knowledge Base (E.Care KB) which contains 664 food concepts and the E.Care dataset, a corpus of 468 cooking recipes where ingredient names have been manually linked to corresponding concepts in the E.Care KB. We developed and evaluated different methods for EL, namely, deep learning-based approaches underpinned by Siamese networks trained under a few-shot learning setting, traditional machine learning-based approaches underpinned by support vector machines (SVMs) and unsupervised approaches based on string matching algorithms. Combining the strengths of each of these approaches, we built a hybrid model for food EL that balances the trade-offs between performance and inference speed. Specifically, our hybrid model obtains 89.40% accuracy and links mentions at an average speed of 0.24 seconds per mention, whereas our best deep learning-based model, SVM model and unsupervised model obtain accuracies of 86.99%, 87.19% and 87.43% at inference speeds of 0.007, 0.66 and 0.02 seconds per mention, respectively.
更多查看译文
关键词
Entity linking,Natural language processing,Machine learning,Food knowledge base
AI 理解论文
溯源树
样例
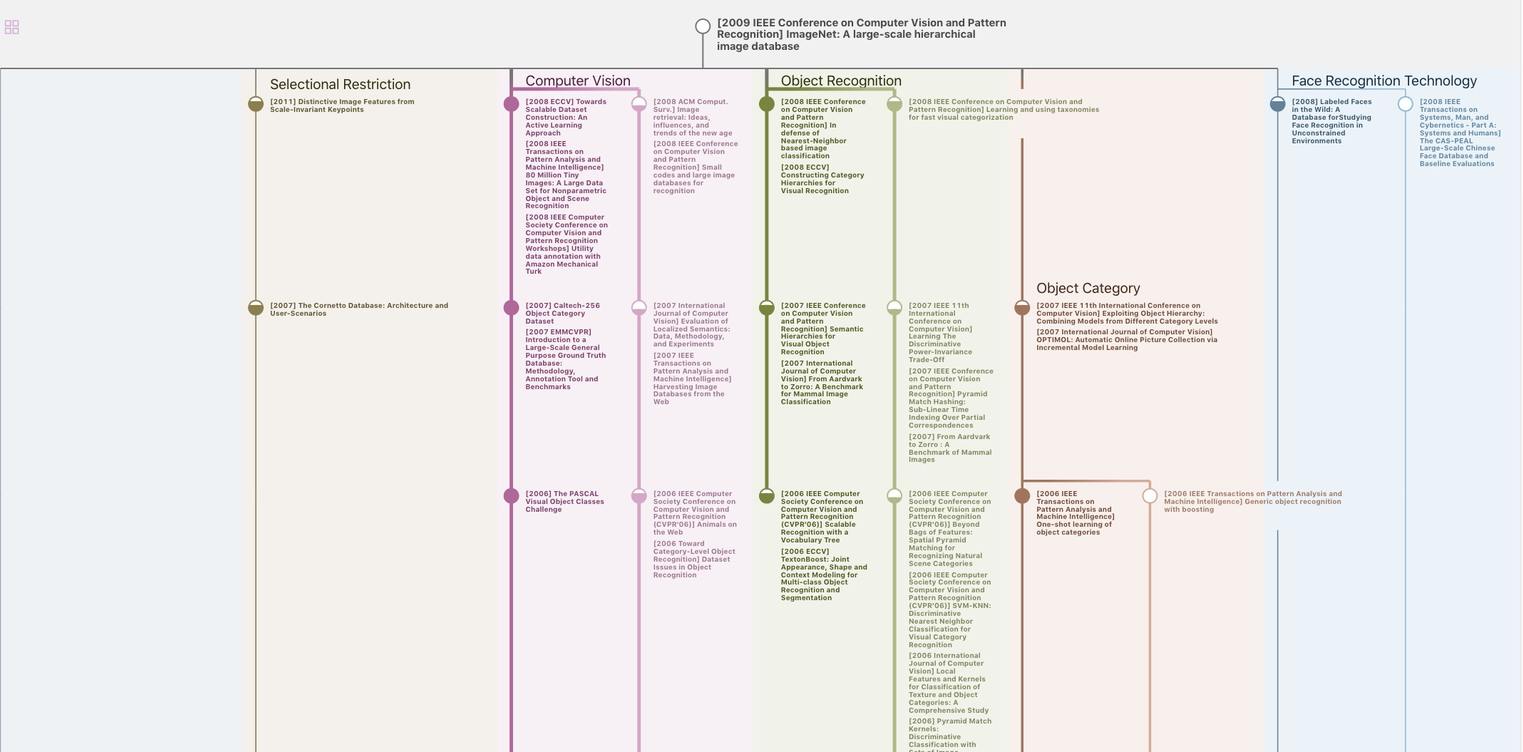
生成溯源树,研究论文发展脉络
Chat Paper
正在生成论文摘要