153. Machine learning generates phenotypes of ASD patients: classification with 2-year prognostic value
The Spine Journal(2023)
摘要
BACKGROUND CONTEXT Adult spinal deformity (ASD) patients (pts) are heterogenous, limiting comparisons in research & clinical practice. We define novel phenotypes based on preoperative(preop) health, age, and baseline(b/l) patient reported outcomes (PROs). PURPOSE To employ machine learning algorithms to reduce the heterogeneity among ASD pts by identifying clusters or “phenotypes” that are defined by baseline health-related quality of life (HRQL) data, frailty, age, and overall health upon presentation. We also assessed the applicability and prognostic value of the classification scheme in a separate, single center cohort. STUDY DESIGN/SETTING Prospective multicenter and retrospective single center/surgeon PATIENT SAMPLE Both (training & validation) cohorts comprised of pts undergoing posterior spinal fusion (PSF) for deformity defined as ≥1: PI-LL≥25°, TPA≥30°, SVA≥15cm, thoracic scoliosis≥70°, or thoracolumbar scoliosis≥50°. The machine learning (ML) algorithm was trained using a prospective multicenter (N=18 centers) cohort (563 pts) and validated on a single center/surgeon cohort with 2Y PROs (242 pts). There was no patient overlap between the two cohorts. OUTCOME MEASURES For both (training & validation) cohorts, we evaluated differences among Clusters 1-4 in demographic, spinal alignment, and Scoliosis Research Society (SRS) Score and Oswestry Disability Index (ODI) (Collectively referred to as “PROs”) upon presentation. For only the validation cohort, we evaluated PROs at 2Y postop. METHODS K-means clustering is a machine learning (ML) algorithm that identifies clusters within heterogenous cohorts. Applied to our multi-institution cohort, clustering based on b/l PROs, frailty, age, and overall health, generated 4 clusters(C1-C4). To determine applicability of our classification in discerning postop trajectories, a second ML algorithm assigned our single-center pts to clusters C1-C4, and we compared clusters’ 2Y PROs using Chi-Squared and ANOVA with pairwise comparisons. RESULTS B/l characteristics of clusters generated from training cohort: C1 pts were older[67.9(0.72), P<0.001 vs C2-4], had high frailty[3.76(0.16), P<0.0001 vs C3-4], frequently had prior fusions[47.4%(74) pts, P<0.0001 vs C3-4], worst sagittal alignment[T1PA 29.0(1.03), P<0.05 vs C2-4] and worst b/l PROs[P<0.0001 vs C2-4), and least coronal deformity [MaxCobb,30.5(1.59), P<0.001 vs C3-4]. In contrast, C4 pts were youngest (38.9[2.31], P<0.0001 vs C1-3), least frail (0.84 [0.15], P<0.0001 vs C1-3), best sagittal alignment (T1PA 11.9 [1.74], P<0.0001 vs C1-3), greatest coronal deformity (54.0 [3.15], P<0.001 vs C1-3), and best b/l PROs (P<0.0001 vs all). C2&C3 had intermediate age (C2 61.4 vs C3 62.3 [1.08]) and intermediate sagittal malalignment (T1PA C2 24.9 vs C3 24.6, P=0.99). C2 (vs C3) had higher frailty (4.72 vs 2.1, P<0.0001), more frequent revision(C2 43.3% vs C3 29.4%, P=0.01), and worse b/l PROs (P<0.0001). Compared to multi-institution clusters, validation cohort clusters had same relative differences in b/l characteristics, indicating that identical “phenotypes” were identified. At 2Y postop, C1 then C2 had worst PROs, and C4 had the best (2Y ODI: 37.5 vs 31.7 vs 18.0 vs 8.88, P=0.69 for C1 vs C2; P<0.001 for other pairs). CONCLUSIONS Phenotypes of ASD pts exist with distinct b/l characteristics that prognosticate outcomes. Heterogeneity among the “elderly revision” cluster in prior classifications (Ames C et al., 2019) can be mitigated by employing b/l PROs & frailty. FDA Device/Drug Status This abstract does not discuss or include any applicable devices or drugs. Adult spinal deformity (ASD) patients (pts) are heterogenous, limiting comparisons in research & clinical practice. We define novel phenotypes based on preoperative(preop) health, age, and baseline(b/l) patient reported outcomes (PROs). To employ machine learning algorithms to reduce the heterogeneity among ASD pts by identifying clusters or “phenotypes” that are defined by baseline health-related quality of life (HRQL) data, frailty, age, and overall health upon presentation. We also assessed the applicability and prognostic value of the classification scheme in a separate, single center cohort. Prospective multicenter and retrospective single center/surgeon Both (training & validation) cohorts comprised of pts undergoing posterior spinal fusion (PSF) for deformity defined as ≥1: PI-LL≥25°, TPA≥30°, SVA≥15cm, thoracic scoliosis≥70°, or thoracolumbar scoliosis≥50°. The machine learning (ML) algorithm was trained using a prospective multicenter (N=18 centers) cohort (563 pts) and validated on a single center/surgeon cohort with 2Y PROs (242 pts). There was no patient overlap between the two cohorts. For both (training & validation) cohorts, we evaluated differences among Clusters 1-4 in demographic, spinal alignment, and Scoliosis Research Society (SRS) Score and Oswestry Disability Index (ODI) (Collectively referred to as “PROs”) upon presentation. For only the validation cohort, we evaluated PROs at 2Y postop. K-means clustering is a machine learning (ML) algorithm that identifies clusters within heterogenous cohorts. Applied to our multi-institution cohort, clustering based on b/l PROs, frailty, age, and overall health, generated 4 clusters(C1-C4). To determine applicability of our classification in discerning postop trajectories, a second ML algorithm assigned our single-center pts to clusters C1-C4, and we compared clusters’ 2Y PROs using Chi-Squared and ANOVA with pairwise comparisons. B/l characteristics of clusters generated from training cohort: C1 pts were older[67.9(0.72), P<0.001 vs C2-4], had high frailty[3.76(0.16), P<0.0001 vs C3-4], frequently had prior fusions[47.4%(74) pts, P<0.0001 vs C3-4], worst sagittal alignment[T1PA 29.0(1.03), P<0.05 vs C2-4] and worst b/l PROs[P<0.0001 vs C2-4), and least coronal deformity [MaxCobb,30.5(1.59), P<0.001 vs C3-4]. In contrast, C4 pts were youngest (38.9[2.31], P<0.0001 vs C1-3), least frail (0.84 [0.15], P<0.0001 vs C1-3), best sagittal alignment (T1PA 11.9 [1.74], P<0.0001 vs C1-3), greatest coronal deformity (54.0 [3.15], P<0.001 vs C1-3), and best b/l PROs (P<0.0001 vs all). C2&C3 had intermediate age (C2 61.4 vs C3 62.3 [1.08]) and intermediate sagittal malalignment (T1PA C2 24.9 vs C3 24.6, P=0.99). C2 (vs C3) had higher frailty (4.72 vs 2.1, P<0.0001), more frequent revision(C2 43.3% vs C3 29.4%, P=0.01), and worse b/l PROs (P<0.0001). Compared to multi-institution clusters, validation cohort clusters had same relative differences in b/l characteristics, indicating that identical “phenotypes” were identified. At 2Y postop, C1 then C2 had worst PROs, and C4 had the best (2Y ODI: 37.5 vs 31.7 vs 18.0 vs 8.88, P=0.69 for C1 vs C2; P<0.001 for other pairs). Phenotypes of ASD pts exist with distinct b/l characteristics that prognosticate outcomes. Heterogeneity among the “elderly revision” cluster in prior classifications (Ames C et al., 2019) can be mitigated by employing b/l PROs & frailty.
更多查看译文
关键词
asd patients,phenotypes,prognostic value,machine learning
AI 理解论文
溯源树
样例
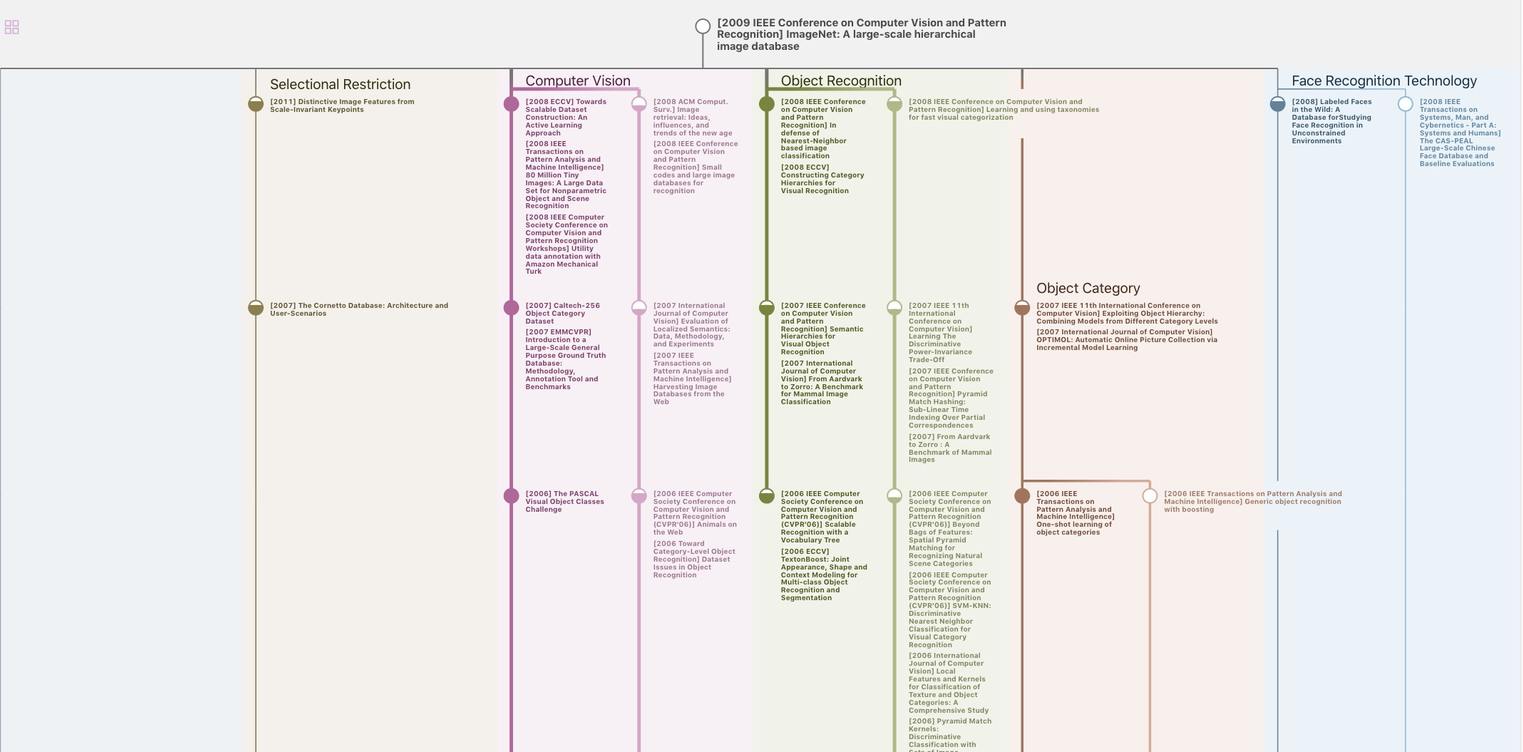
生成溯源树,研究论文发展脉络
Chat Paper
正在生成论文摘要