FRNet: An MCS Framework for Efficient and Secure Data Sensing and Privacy Protection in IoVs
IEEE Internet of Things Journal(2023)
摘要
Mobile crowdsensing (MCS) is a kind of sensing mode based on mobile nodes, which provides a cost-effective solution for data collection of urban sensing. However, when participants collect and upload sensing data in MCS system, they often overlap in time and space. Therefore, MCS system obtains a lot of redundant data, and ultimately cannot meet the MCS task requirements. Meanwhile, existing schemes cannot realize the dynamic allocation of sensing tasks and the dynamic selection of task participants. In addition, task participants will generate and transfer a large amount of sensitive information, such as location, identity, personal habits, etc., in the process of uploading sensing data and completing sensing tasks, thus leading to the risk of privacy leakage. Presently, many schemes use federated learning and other technologies to protect privacy, but there are still challenges that adversaries can extract parameters information during model training. Therefore, in order to the above problems, this article puts forward an efficient and secure MCS framework FRNet in Internet of Vehicles (IoVs). Specifically, MCS task completion is described as a two-phase process. This article not only proposes a method to dynamically select candidate participants in MCS vehicular networks to collect high-quality sensing data, but also establishes a data privacy protection mechanism for the whole process of model training in federated learning. Finally, the effectiveness of FRNet is verified through security analysis and experimental evaluation. Experimental results show that FRNet can effectively improve the quality of sensing data, maximize TCV, and enhance data privacy protection ability.
更多查看译文
关键词
secure data sensing,mcs framework,privacy protection
AI 理解论文
溯源树
样例
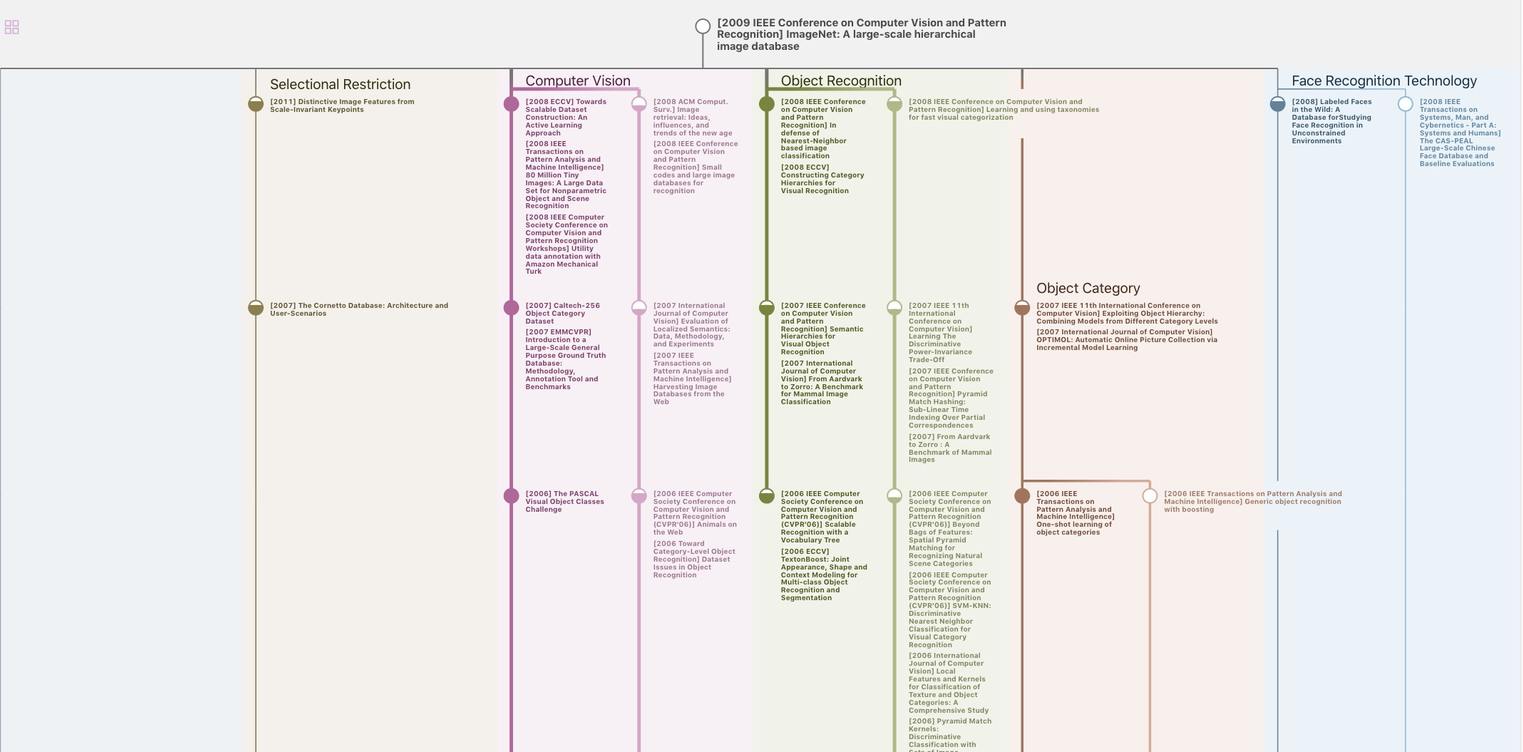
生成溯源树,研究论文发展脉络
Chat Paper
正在生成论文摘要