Robust Low-Rank Graph Multi-View Clustering via Cauchy Norm Minimization
MATHEMATICS(2023)
摘要
Graph-based multi-view clustering methods aim to explore the partition patterns by utilizing a similarity graph. However, many existing methods construct a consensus similarity graph based on the original multi-view space, which may result in the lack of information on the underlying low-dimensional space. Additionally, these methods often fail to effectively handle the noise present in the graph. To address these issues, a novel graph-based multi-view clustering method which combines spectral embedding, non-convex low-rank approximation and noise processing into a unit framework is proposed. In detail, the proposed method constructs a tensor by stacking the inner product of normalized spectral embedding matrices obtained from each similarity matrix. Then, the obtained tensor is decomposed into a low-rank tensor and a noise tensor. The low-rank tensor is constrained via nonconvex low-rank tensor approximation and a novel Cauchy norm with an upper bound is proposed to handle the noise. Finally, we derive the consensus similarity graph from the denoised low-rank tensor. The experiments on five datasets demonstrate that the proposed method outperforms other state-of-the-art methods on five datasets.
更多查看译文
关键词
multi-view clustering, graph-based method, low-rank tensor learning, denoise handling
AI 理解论文
溯源树
样例
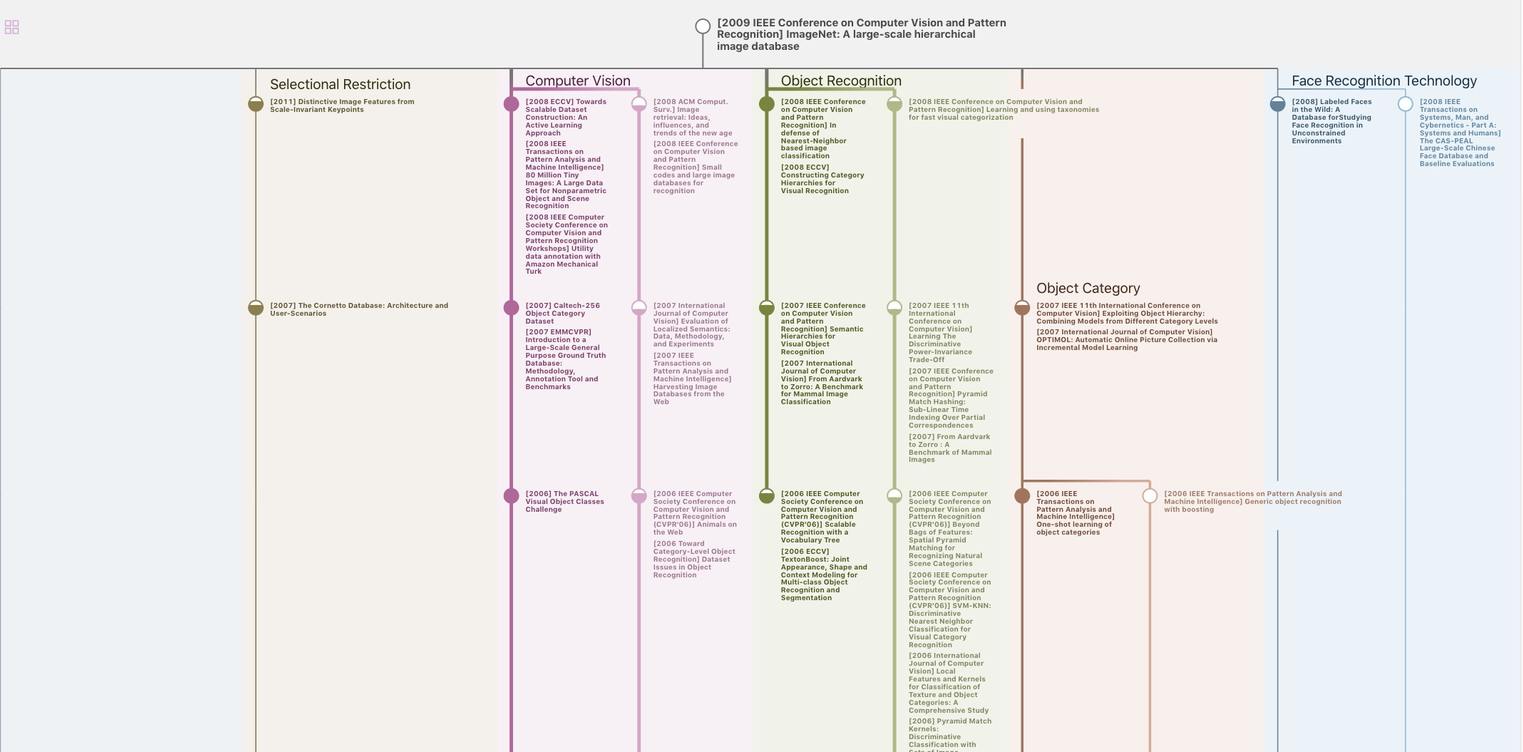
生成溯源树,研究论文发展脉络
Chat Paper
正在生成论文摘要