Variable Selection for Meaningful Clustering of Multitopic Territorial Data
MATHEMATICS(2023)
摘要
This paper proposes a new methodology to improve territorial cohesion in clustering processes where many variables from different topics are considered. Clustering techniques provide added value to identify typologies, but there are still unsolved challenges when data contain an unbalanced number of variables from different topics. The territorial feature selection method (TFSM) is presented as a method to select the representative variable of each topic such that the interpretability of resulting clusters is preserved and the geographical cohesion is improved with respect to classical approaches. This paper also introduces the thermometer as a new knowledge acquisition tool that allows experts to transfer semantics to the data mining process. TFSM proposes the index of potential explainability (E-k) as the criteria to select the most promising variables for clustering. E-k is based on the combination of inferential testing and metrics such as support. The proposal is applied with the INSESS-COVID19 database, where territorial groups of vulnerable populations were found. A set of 195 variables with 21 unbalanced thematic blocks is used to compare the results with a traditional multiview clustering analysis with promising results from both the geographical and the thematic point of view and the capacity to support further decision making.
更多查看译文
关键词
data science, intelligent decision support, COVID-19, traffic light panels, thermometer, feature selection, explainable AI, maps, Catalonia
AI 理解论文
溯源树
样例
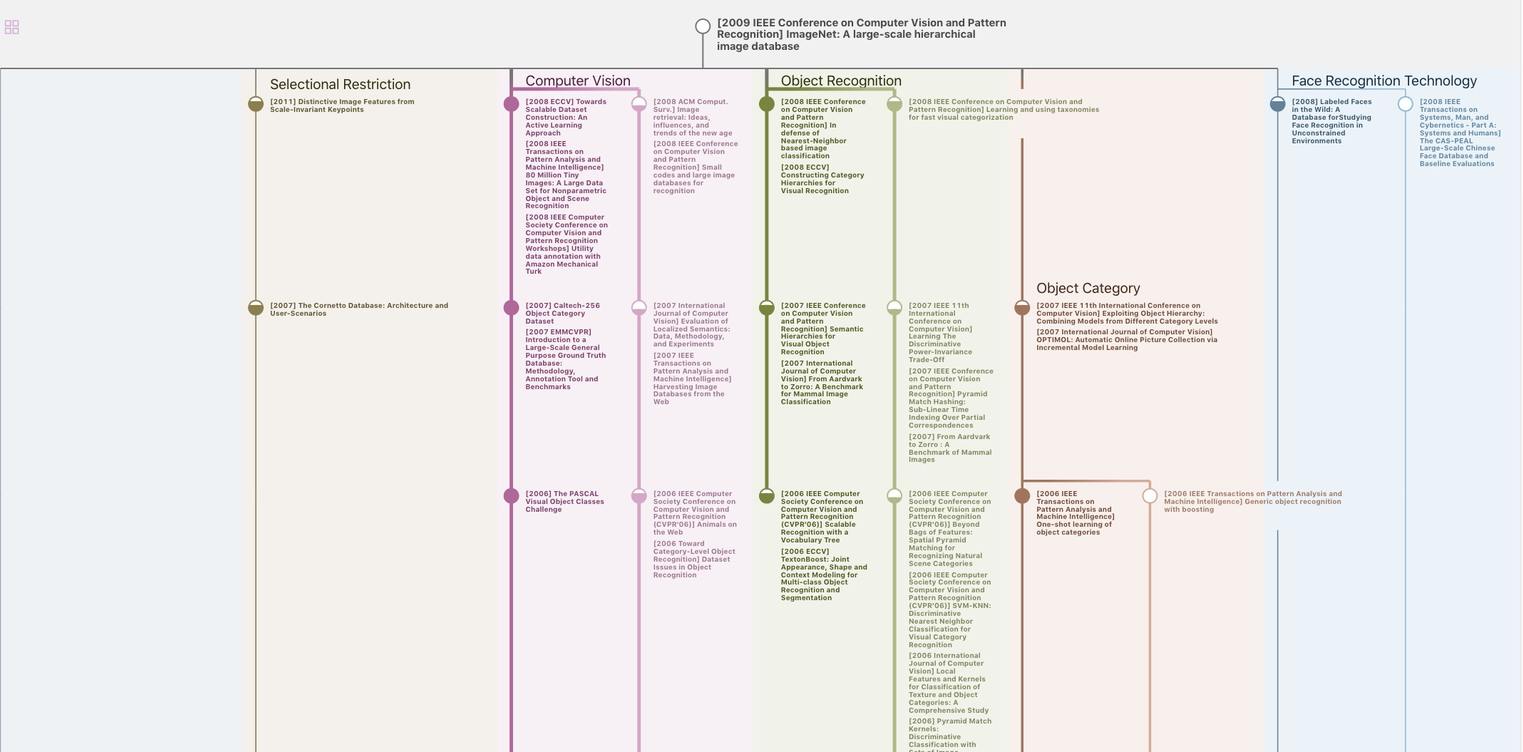
生成溯源树,研究论文发展脉络
Chat Paper
正在生成论文摘要