On the order of derivation in the training of physics-informed neural networks: case studies for non-uniform beam structures
ACTA MECHANICA(2023)
摘要
The potential of the mixed formulation for physics-informed neural networks is investigated in order to find a solution for a non-uniform beam resting on an elastic foundation and subjected to arbitrary external loading. These types of structures are commonly encountered in civil engineering problems at larger scales, as well as in the design of new generation meta-materials at smaller scales. The mixed formulation approach aims to predict not only the primary variable itself but also its higher derivatives. In the context of this work, the primary variable is the beam deflection, while its higher-order derivatives are associated with the shear stress and moment within the beam structure. By employing this new methodology, it becomes possible to reduce the order of the derivatives in the physical constraints used for training the neural networks. As a result, significantly more accurate predictions can be achieved compared to the standard approach. The obtained results are validated by comparison against available analytical solutions or those obtained from the finite element method. Finally, the potential of the proposed method is discussed in terms of its ability to be combined with available data in order to predict the solution for an arbitrary non-uniform beam resting on an elastic foundation.
更多查看译文
关键词
neural networks,beam,derivation,physics-informed,non-uniform
AI 理解论文
溯源树
样例
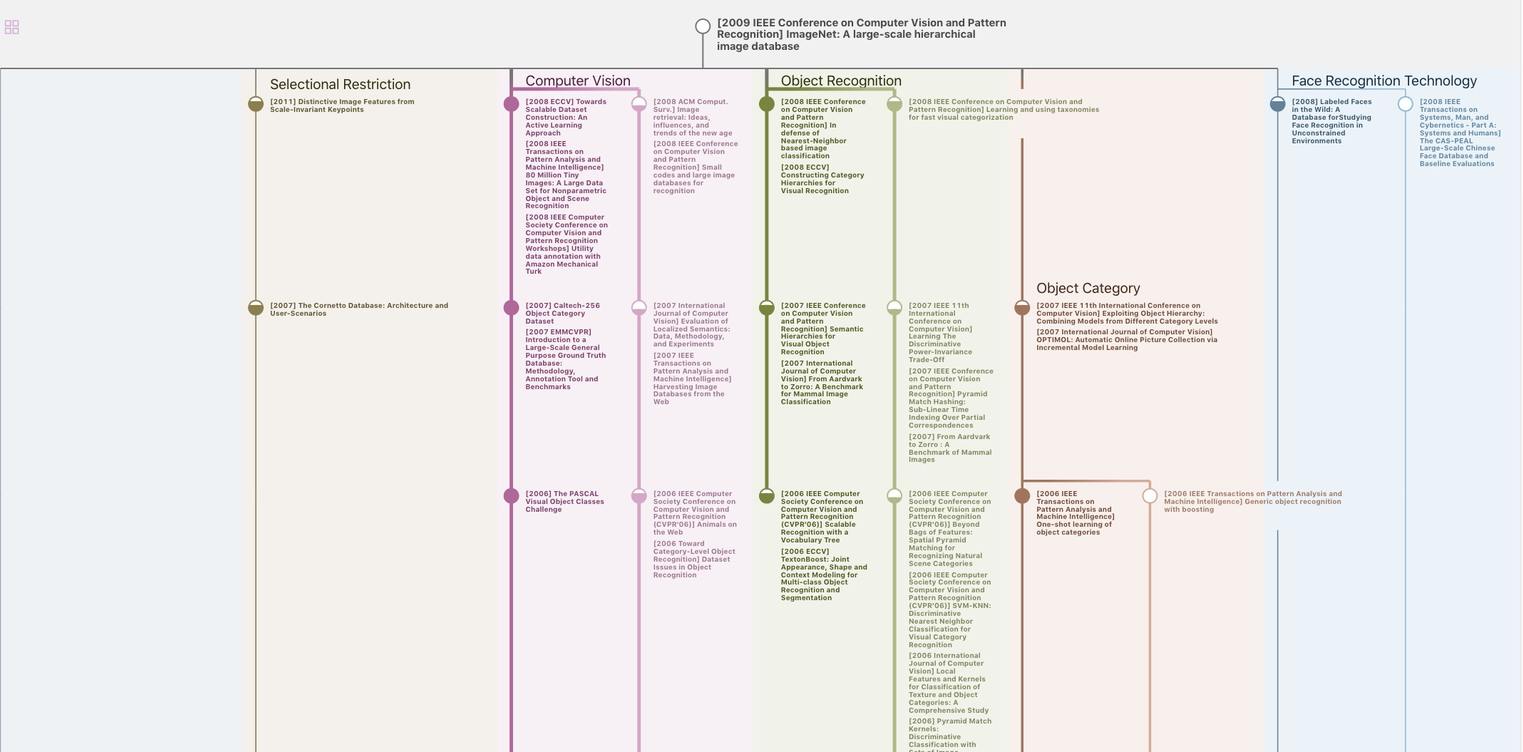
生成溯源树,研究论文发展脉络
Chat Paper
正在生成论文摘要