Understanding and control of Zener pinning via phase field and ensemble learning
COMPUTATIONAL MATERIALS SCIENCE(2023)
摘要
Zener pinning refers to the dispersion of fine particles which influences grain size distribution via movement of grain boundaries in a polycrystalline material. Grain size distribution in polycrystals has a significant impact on their properties including physical, chemical, mechanical, and optical to name a few. We explore the use of Phase-field modeling and machine-learning techniques to understand and improve the control of grain size distribution via Zener pinning in polycrystalline materials. We develop a machine learning model that determines the relative importance of various parameters to exercise microstructure control via Zener pinning. Our workflow combines high-throughput phase-field simulations and machine learning to address the computational bottlenecks associated with large-scale simulations as well as identify features necessary for microstructure control in polycrystals. A random forest (RF) regression model was developed to predict grain sizes based on five Phase-field model parameters, achieving an average prediction error of 0.72 nm for the training data and 1.44 nm for the test data. The importance of the input parameters is analyzed using the SHapley Additive exPlanations (SHAP) approach which reveals that diffusivity, volume fraction, and particle diameter are the most important parameters in determining the final grain size. These findings will allow us to select the best second-phase particles, optimize grain size distributions and thus design microstructures with the desired properties. The developed method is a highly versatile and generalizable approach that can be used to assess the combined effects of individual features in the presence of multiple variables.
更多查看译文
关键词
Phase field modeling,Zener pinning,Ensemble learning,Machine learning
AI 理解论文
溯源树
样例
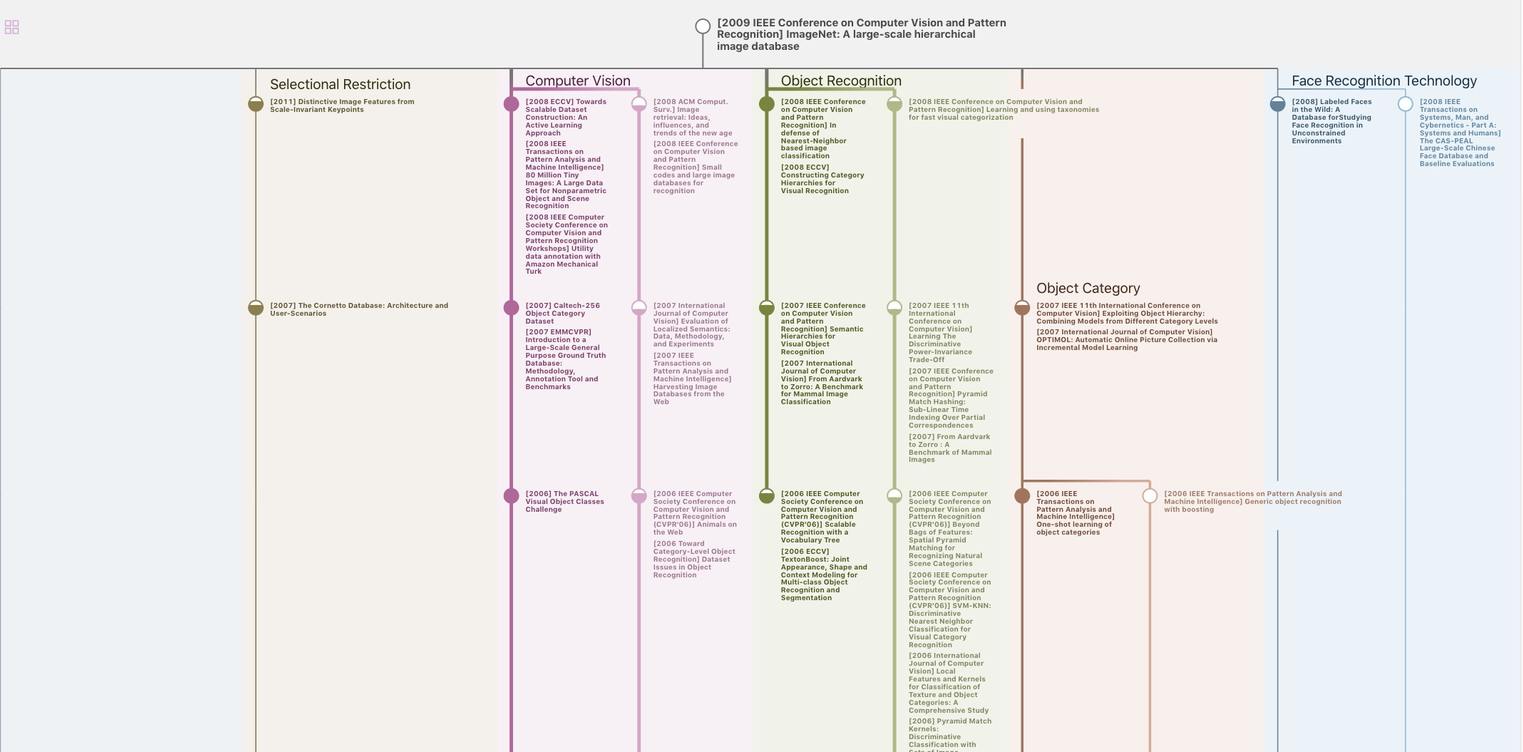
生成溯源树,研究论文发展脉络
Chat Paper
正在生成论文摘要