Ensemble Transfer Learning Based Cross-Domain UAV Actuator Fault Detection
IEEE SENSORS JOURNAL(2023)
摘要
The actuator is critical for controlling the unmanned aerial vehicle (UAV) attitude. With the development of sensing technology, the convenience of collecting high-quality data by sensors has provided favorable conditions for data-driven-based UAV actuator fault detection methods. However, there are less historical data on a new type of UAV, making it challenging to accurately obtain data-driven fault detection models. The data domain distribution of the new UAV (target domain) is different from that of existing UAVs (source domains), and the basic data-driven methods are difficult to achieve cross-domain fault detection. The transfer learning of a single source domain method can solve this problem, but it has a strong dependence on the single source domain data characteristics. Therefore, we propose the ensemble transfer learning method, which learns multiple UAV data characteristics to address the accuracy degradation issue caused by single-source domain insufficient characteristics and realize cross-domain actuator fault detection with limited data for the new type of UAV. In the method, the basic actuator prediction models are established based on the bidirectional long short-term memory (BiLSTM) algorithm. Then, we combine the transfer learning method to realize the cross-domain fault detection model establishment of the new type of UAV. In addition, a cross-domain similarity metric based on R -score is creatively proposed for the ensemble to avoid excessive dependence on a single-source domain. We conduct experiments based on the ArduPilot open-source simulation platform, and the results show that our proposed method can adaptively obtain more accurate fault detection performance.
更多查看译文
关键词
Cross-domain similarity metric,ensemble transfer learning,fault detection,unmanned aerial vehicle (UAV)
AI 理解论文
溯源树
样例
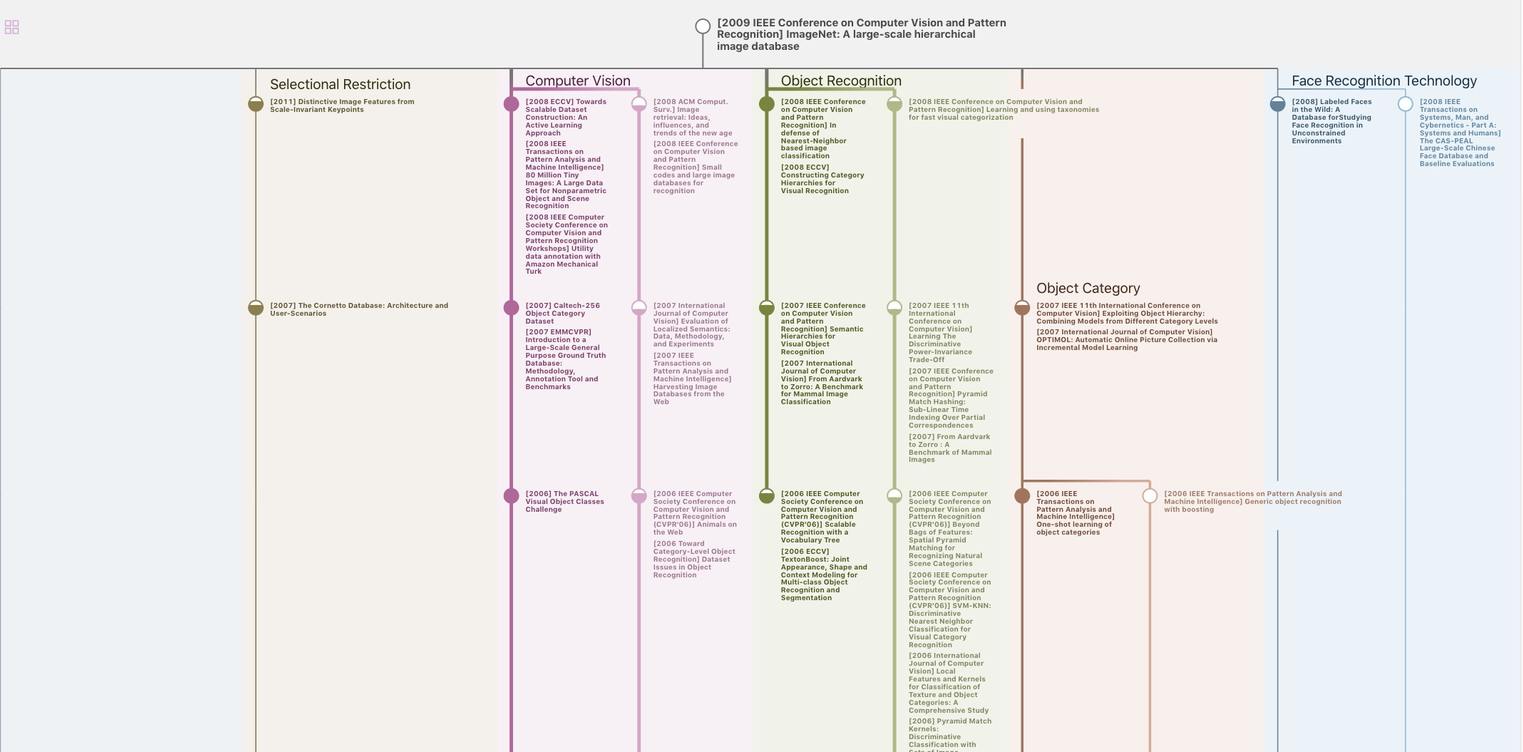
生成溯源树,研究论文发展脉络
Chat Paper
正在生成论文摘要