A Transformer-based computational approach for H&E to multiplexed immunohistochemistry stain translation
MEDICAL IMAGING 2023(2023)
摘要
Understanding the spatial protein expression and cellular interaction processes in tissue is becoming increasingly important to improve understanding of disease biology and develop novel diagnostics. Modern staining methods such as multiplexed immunohistochemistry staining (mIHC) can achieve visualization of multiple-protein fluorescent markers within the tumor microenvironment, however, the preparation process is very costly and complex. Recent works have demonstrated the feasibility of deep learning-based methods to perform virtual stain translation - a process by which protein expression is predicted directly from H&E microscopy images. The majority of prior work is based on traditional convolutional neural networks. Recent studies have demonstrated the strengths of Transformer modules in capturing global information which is essential in inferring a cells context in the wider tissue. In this work, we used H&E images as input and demonstrated the performance of a Transformer-CNN hybrid model as TransUNet to translate the input images into their corresponding mIHC versions which can simultaneously show DAPI, CD3, CD20, and panCK markers. We found that training the model with L1 and L2 losses yields very different results. For the L1 loss, models tend to ignore low-expression markers like CD3 and CD20, however, could produce results with high SSIM scores. The TransUNet model reaches an average SSIM score as 0.862, surpassing U-Net and pix2pix. As for models trained with L2 loss, the final results have shown that the TransUNet model could achieve 0.805 in SSIM score, also better than U-Net and pix2pix model. We also introduced an L1-L2 combined loss function and evaluated its performance. The findings show that transformer-based deep learning methods could potentially be used to infer cell protein expression as part of a fast and cost-effective screening tool for diagnostics and research.
更多查看译文
关键词
Deep learning, mIHC, H&E, stain translation, transformers
AI 理解论文
溯源树
样例
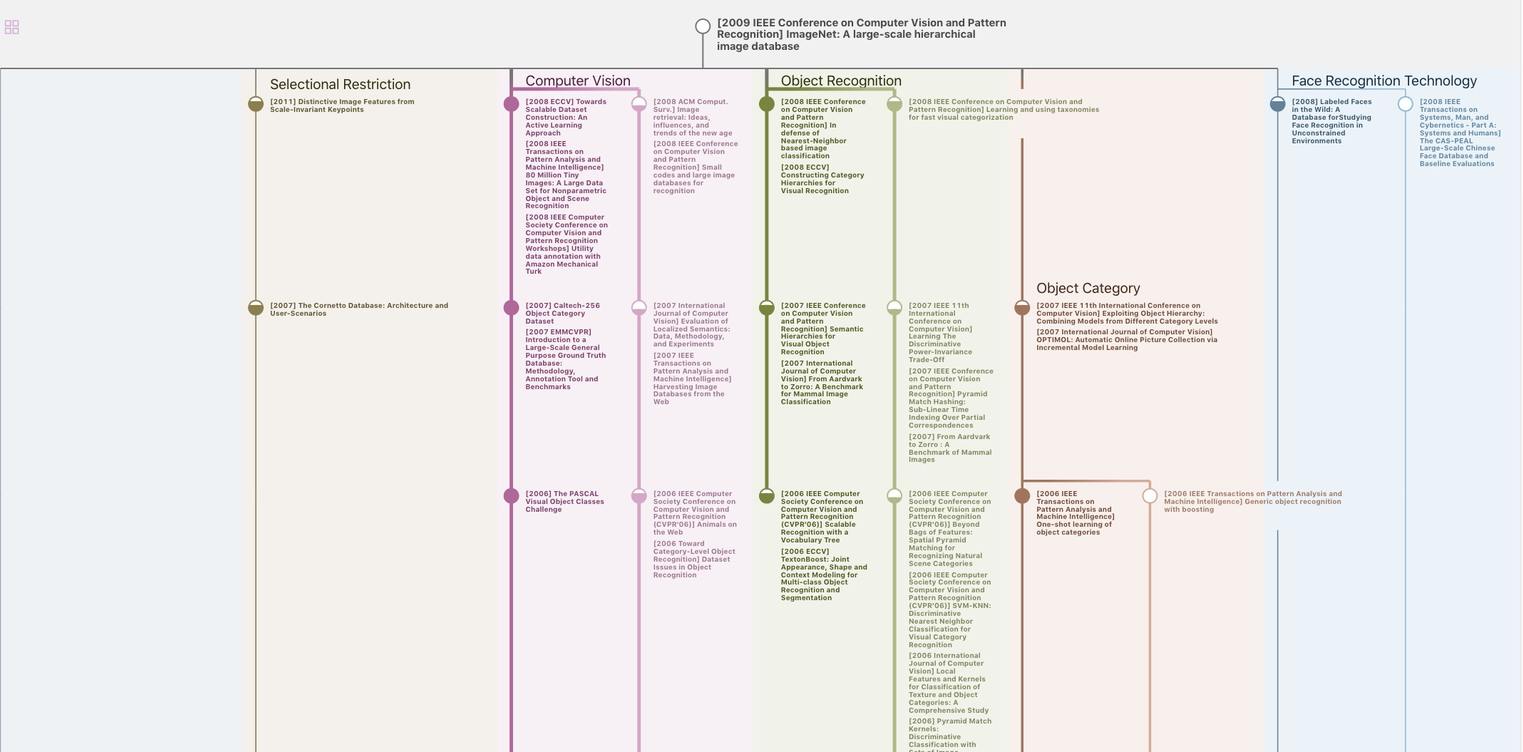
生成溯源树,研究论文发展脉络
Chat Paper
正在生成论文摘要