Application of machine learning and genetic algorithms to the prediction and optimization of biodiesel yield from waste cooking oil
KOREAN JOURNAL OF CHEMICAL ENGINEERING(2023)
摘要
The synthesis and usage of biodiesel have become the focus of extensive research due to the ever-increasing emphasis on the development of sustainable and renewable sources. As biodiesel yield and quality depend on the feedstocks used in transesterification, numerous process variables must be controlled at optimal levels to ensure high productivity throughout the biodiesel synthesis process. This study provides three machine learning-based approaches (Gradient boosting, eXtreme gradient boosting (XGB), and light gradient boosting machine (LGBM) regression) for prediction and genetic algorithm (GA) for the optimization of biodiesel yield from waste cooking oil. Throughout the modeling, training, testing, and cross-validation processes, supervised machine learning methods were used to analyze the datasets exhaustively. Four performance indicators (MAE, RMSE, MAPE, and R 2 ) were used to evaluate and contrast the precision of the algorithms. Based on the findings of the coefficient of determination, the LGBM algorithm has the highest prediction accuracy of 0.94048, followed by XGB with 0.8631 accuracy and gradient boosting with 0.8547 accuracy. The optimal condition for producing biodiesel through GA was a molar ratio of 7.62:1 with a catalyst concentration of 0.5 wt%, a reaction temperature of 65 °C, and a reaction time of 105 min and the corresponding value of the biodiesel yield was 98.98% (by wt.). Experiments confirmed this prediction, and with acceptable error, all results are close to the model’s predicted values. The LGBM coupled with GA can be used as a strategic decision-support and optimization tool for the production of high-quality biodiesel.
更多查看译文
关键词
biodiesel yield,waste cooking oil,genetic algorithms,machine learning,prediction
AI 理解论文
溯源树
样例
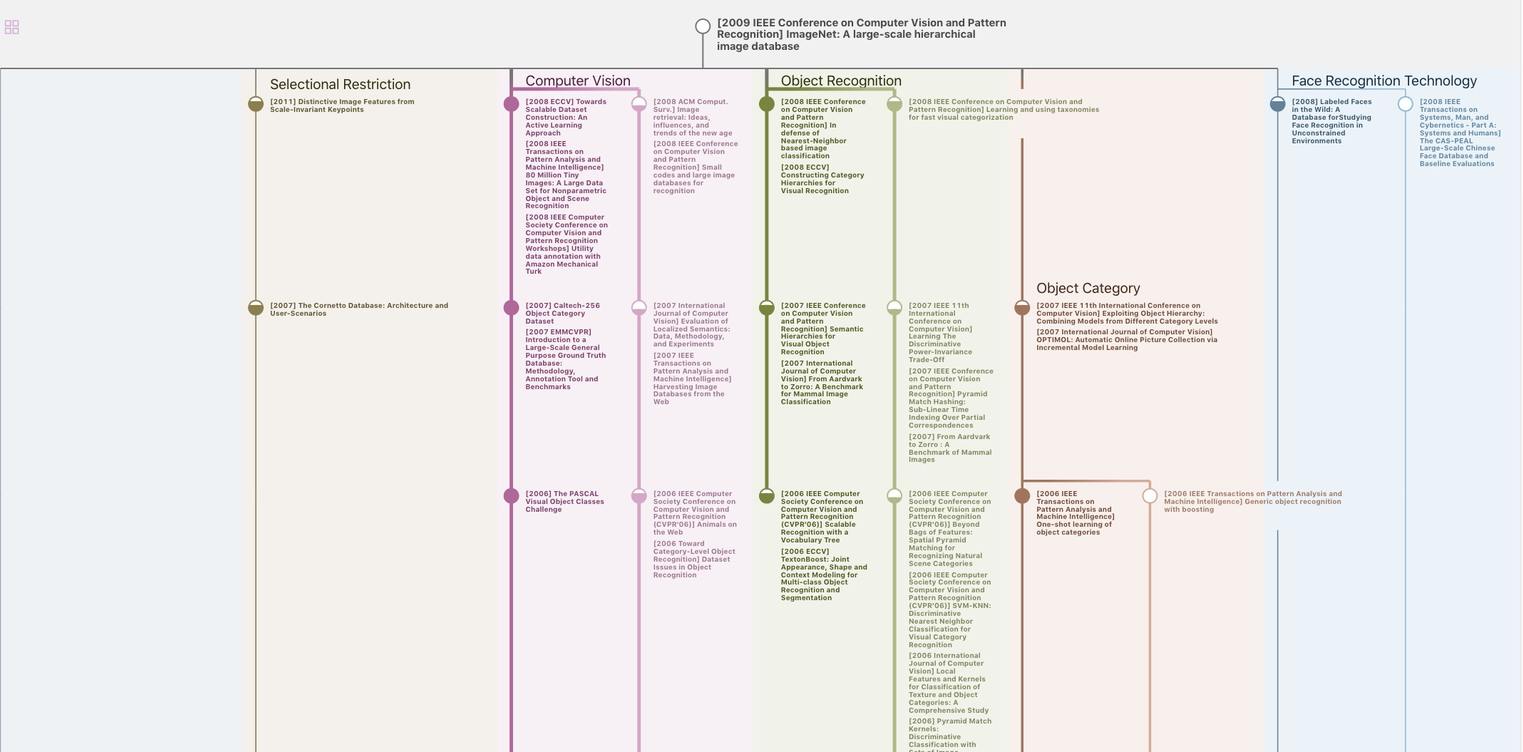
生成溯源树,研究论文发展脉络
Chat Paper
正在生成论文摘要