Qoe-guaranteed distributed offloading decision via partially observable deep reinforcement learning for edge-enabled Internet of Things
Neural Comput. Appl.(2023)
摘要
In edge-enabled Internet of Things (IoT), Quality of Experience (QoE)-guaranteed offloading decision is to decide which IoT tasks can be offloaded to edge servers with QoE guarantee. Centralized QoE-guaranteed offloading decision methods construct a global decision model for all IoT tasks with complete information. However, centralized offloading decision methods entail collecting global information from IoT devices, edge servers, and network environment, which may not be practical in large-scale distributed edge-enabled IoT environments, and it is unrealistic for privacy-critical and heterogeneous IoT tasks in many real-world edge-enabled IoT systems, where IoT devices may refuse to expose their private information and heterogeneous IoT tasks may have different QoE requirements, these issues make the application of centralized offloading decision method limited. To address these limitations, we propose a distributed offloading decision method which enables each IoT device to make decisions by partially observable global information in a decentralized manner. The distributed offloading decision process is modeled as a multi-agent partially observable Markov decision process, and a novel model-free deep reinforcement learning-based distributed algorithm named GRU Fictitious Self-Play Dueling Double Deep Recurrent Q Network(GFSP-D3RQN) is introduced to solve the problem. Furthermore, we measure the QoE of each IoT device based on a combination of latency and energy consumption, which are weighted differently according to the individual preferences of each IoT device, using a non-dimensionalized adjustment to accommodate the varying requirements of these IoT devices. Extensive simulation results show that our algorithm can achieve a higher average QoE and higher success ratio compared with baseline algorithms, which improved by at least 6.38 % and 5.91 % , respectively.
更多查看译文
关键词
Distributed decision,Task offloading,Quality of experience,Edge computing,Deep reinforcement learning
AI 理解论文
溯源树
样例
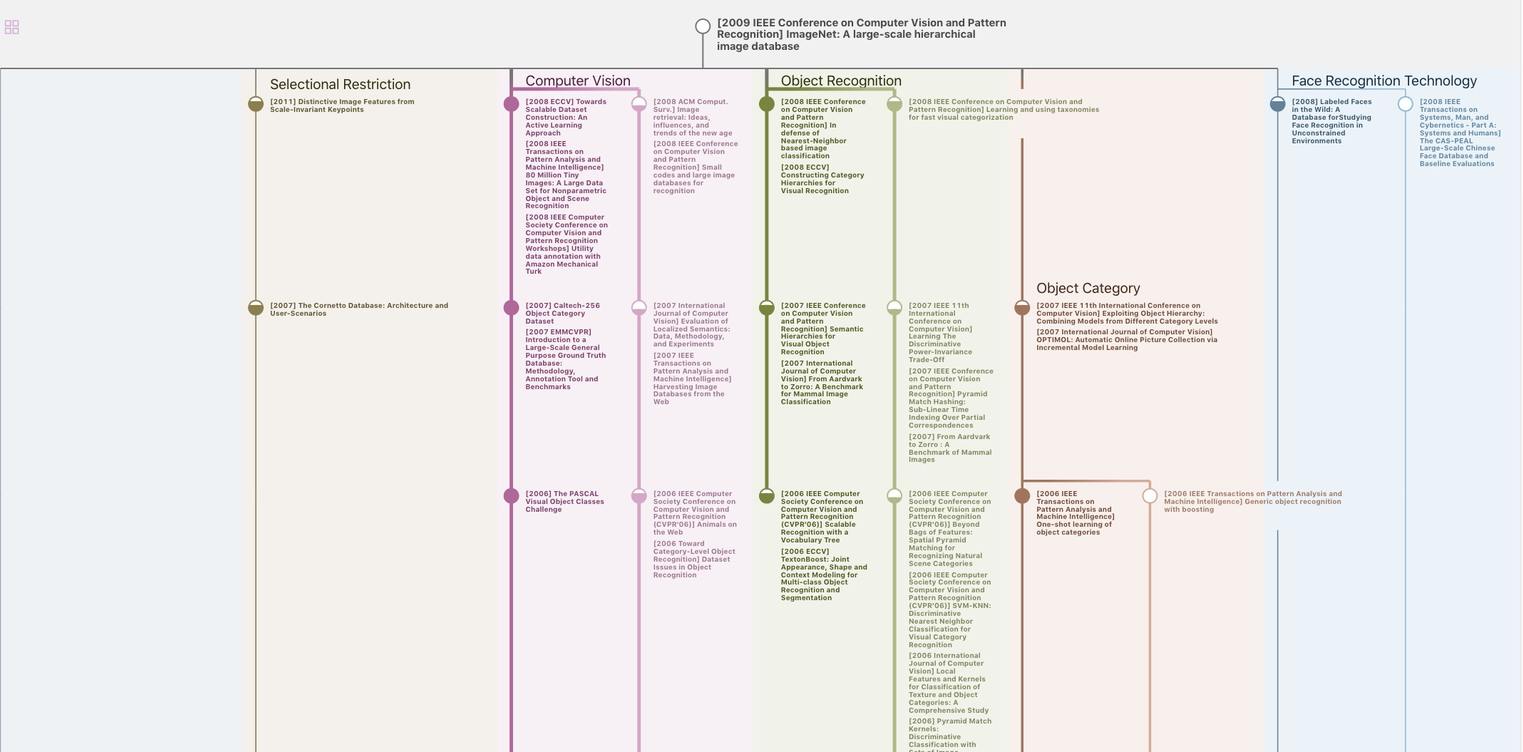
生成溯源树,研究论文发展脉络
Chat Paper
正在生成论文摘要