A Fast Density Peak Clustering Method for Power Data Security Detection Based on Local Outlier Factors
PROCESSES(2023)
摘要
The basic work of power data research is anomaly detection. It is necessary to find a method suitable for processing current power system data. Research proposes an algorithm of fast density peak clustering with Local Outlier Factor (LOF). The algorithm has poor performance in processing datasets with irregular shapes and significant local density changes, and has the disadvantage of strong dependence on truncation distance. This study provides the decision rules for outliers incorporating the idea of LOF. The improved algorithm can fully consider the characteristics of power data and reduce the dependence on truncation distance. In anomaly detection based on the simulation of real power data, the classification accuracy of the improved CFSFDP algorithm is 4.87% higher than that of the traditional algorithm, and the accuracy rate is 97.41%. The missed and false detection rates of the LOF-CFSFDP algorithm are decreased by 2.23% and 2.64%, respectively, compared to the traditional algorithm, and it is ultimately able to reach rates of 1.26% and 1.33%. These results indicate that the algorithm proposed in this study can better describe the characteristics of power data, making the features of outliers and cluster center points more obvious.
更多查看译文
关键词
local outlier factors,density,distance,power data,principal component analysis,anomaly detection
AI 理解论文
溯源树
样例
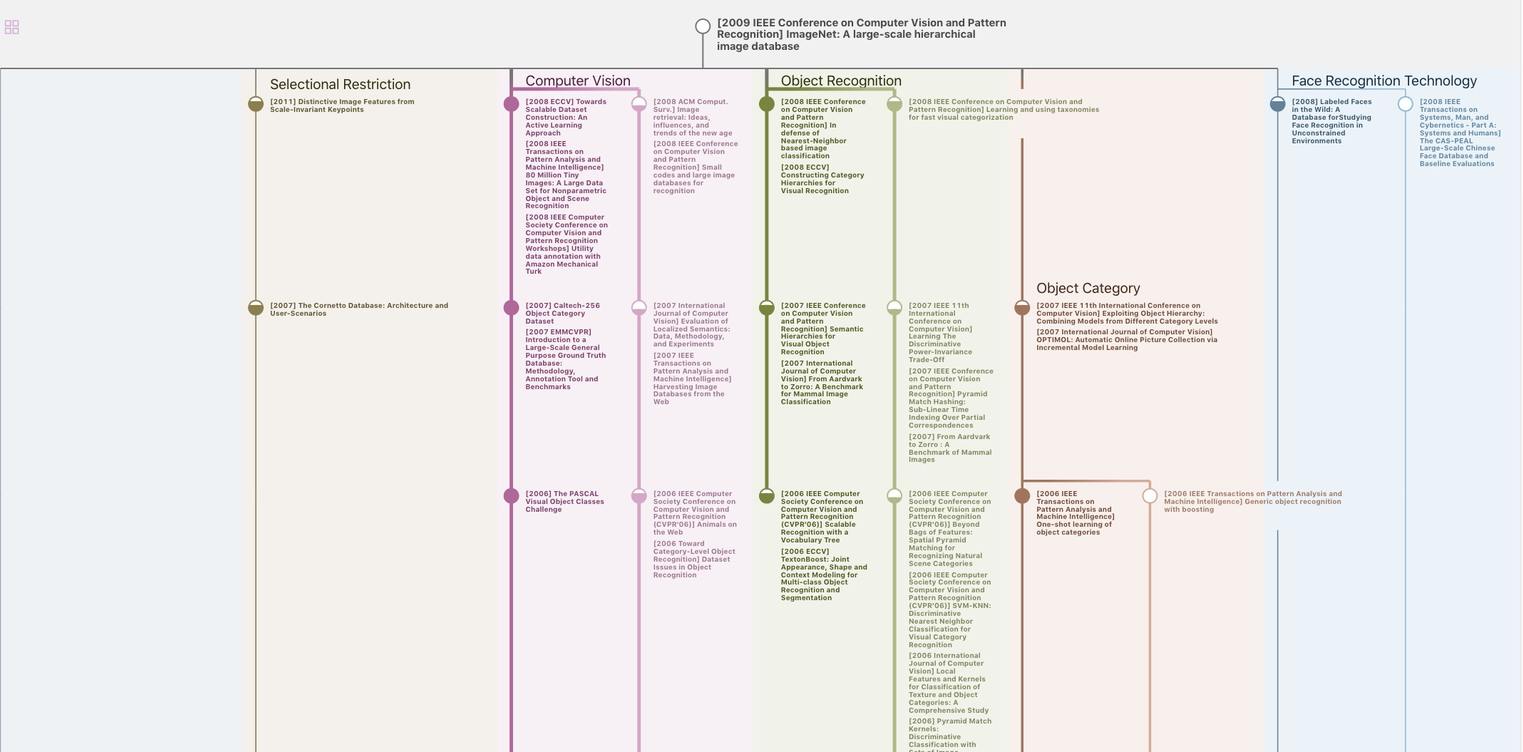
生成溯源树,研究论文发展脉络
Chat Paper
正在生成论文摘要