Zero-shot temporal event localisation: Label-free, training-free, domain-free
IET COMPUTER VISION(2023)
摘要
Temporal event localisation (TEL) has recently attracted increasing attention due to the rapid development of video platforms. Existing methods are based on either fully/weakly supervised or unsupervised learning, and thus they rely on expensive data annotation and time-consuming training. Moreover, these models, which are trained on specific domain data, limit the model generalisation to data distribution shifts. To cope with these difficulties, the authors propose a zero-shot TEL method that can operate without training data or annotations. Leveraging large-scale vision and language pre-trained models, for example, CLIP, we solve the two key problems: (1) how to find the relevant region where the event is likely to occur; (2) how to determine event duration after we find the relevant region. Query guided optimisation for local frame relevance relying on the query-to-frame relationship is proposed to find the most relevant frame region where the event is most likely to occur. Proposal generation method relying on the frame-to-frame relationship is proposed to determine the event duration. The authors also propose a greedy event sampling strategy to predict multiple durations with high reliability for the given event. The authors' methodology is unique, offering a label-free, training-free, and domain-free approach. It enables the application of TEL purely at the testing stage. The practical results show it achieves competitive performance on the standard Charades-STA and ActivityCaptions datasets.
更多查看译文
关键词
computer vision,video retrieval
AI 理解论文
溯源树
样例
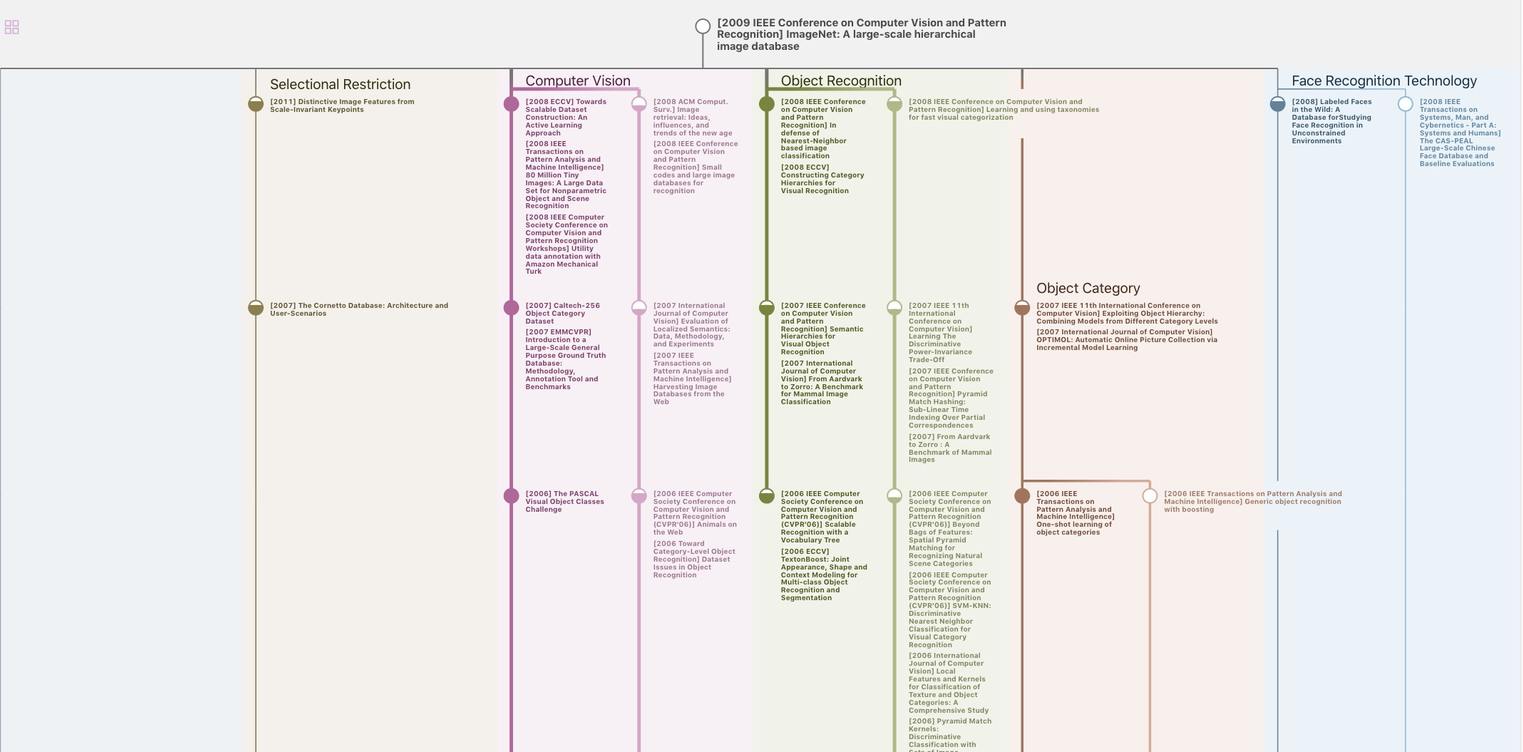
生成溯源树,研究论文发展脉络
Chat Paper
正在生成论文摘要