An introduction to representation learning for single-cell data analysis
Cell reports methods(2023)
摘要
Single-cell-resolved systems biology methods, including omics- and imaging-based measurement modalities, generate a wealth of high-dimensional data characterizing the heterogeneity of cell populations. Representation learning methods are routinely used to analyze these complex, high-dimensional data by projecting them into lower-dimensional embeddings. This facilitates the interpretation and interrogation of the structures, dynamics, and regulation of cell heterogeneity. Reflecting their central role in analyzing diverse single-cell data types, a myriad of representation learning methods exist, with new approaches continually emerging. Here, we contrast general features of representation learning methods spanning statistical, manifold learning, and neural network approaches. We consider key steps involved in representation learning with single-cell data, including data pre-processing, hyperparameter optimization, downstream analysis, and biological validation. Interdependencies and contingencies linking these steps are also highlighted. This overview is intended to guide researchers in the selection, application, and optimization of representation learning strategies for current and future single-cell research applications.
更多查看译文
关键词
deep learning,manifold learning,dimension reduction,hyperparameter,omics,systems microscopy
AI 理解论文
溯源树
样例
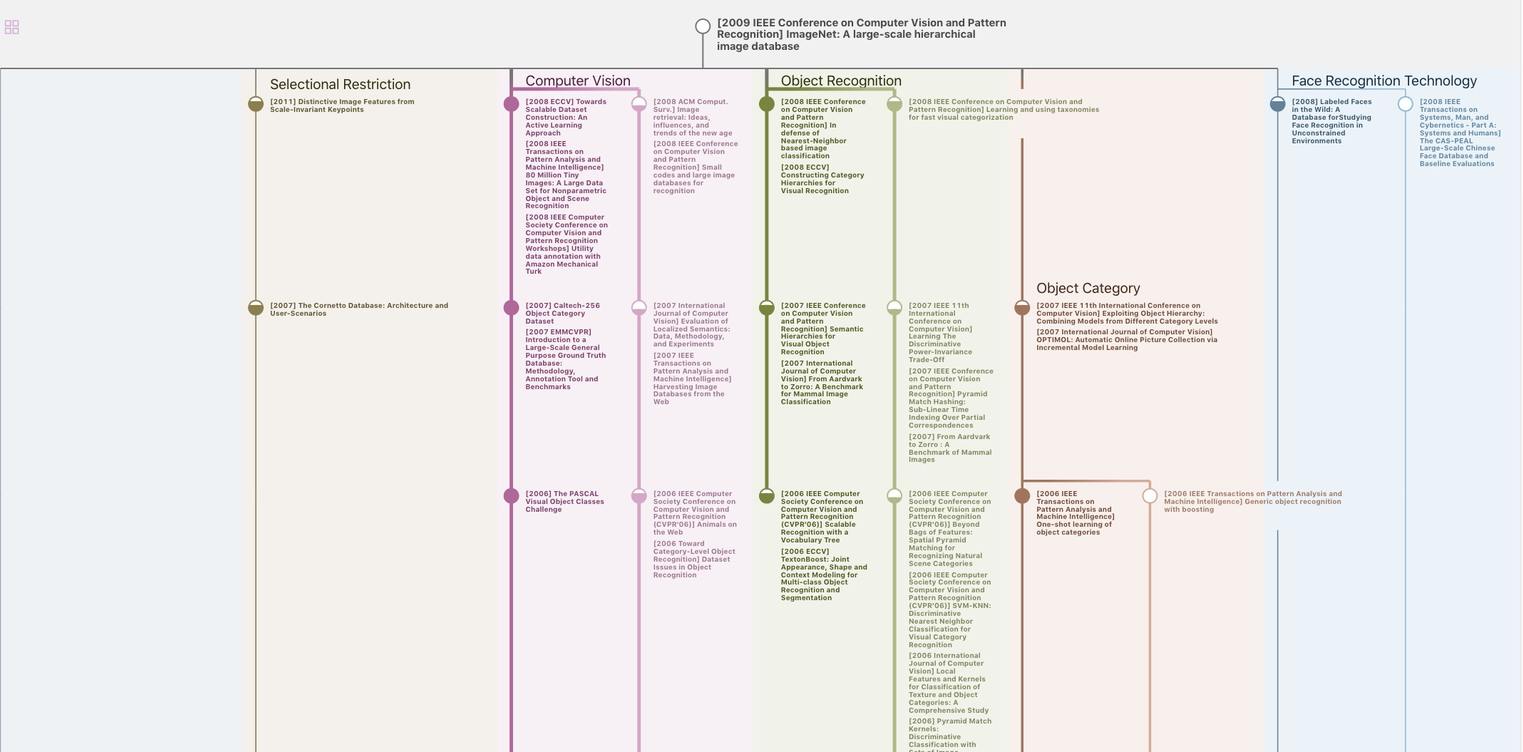
生成溯源树,研究论文发展脉络
Chat Paper
正在生成论文摘要