Tempestas ex machina: A review of machine learning methods for wavefront control
CoRR(2023)
摘要
As we look to the next generation of adaptive optics systems, now is the time to develop and explore the technologies that will allow us to image rocky Earth-like planets; wavefront control algorithms are not only a crucial component of these systems, but can benefit our adaptive optics systems without requiring increased detector speed and sensitivity or more effective and efficient deformable mirrors. To date, most observatories run the workhorse of their wavefront control as a classic integral controller, which estimates a correction from wavefront sensor residuals, and attempts to apply that correction as fast as possible in closed-loop. An integrator of this nature fails to address temporal lag errors that evolve over scales faster than the correction time, as well as vibrations or dynamic errors within the system that are not encapsulated in the wavefront sensor residuals; these errors impact high contrast imaging systems with complex coronagraphs. With the rise in popularity of machine learning, many are investigating applying modern machine learning methods to wavefront control. Furthermore, many linear implementations of machine learning methods (under varying aliases) have been in development for wavefront control for the last 30-odd years. With this work we define machine learning in its simplest terms, explore the most common machine learning methods applied in the context of this problem, and present a review of the literature concerning novel machine learning approaches to wavefront control.
更多查看译文
关键词
machine learning methods,machine learning,control
AI 理解论文
溯源树
样例
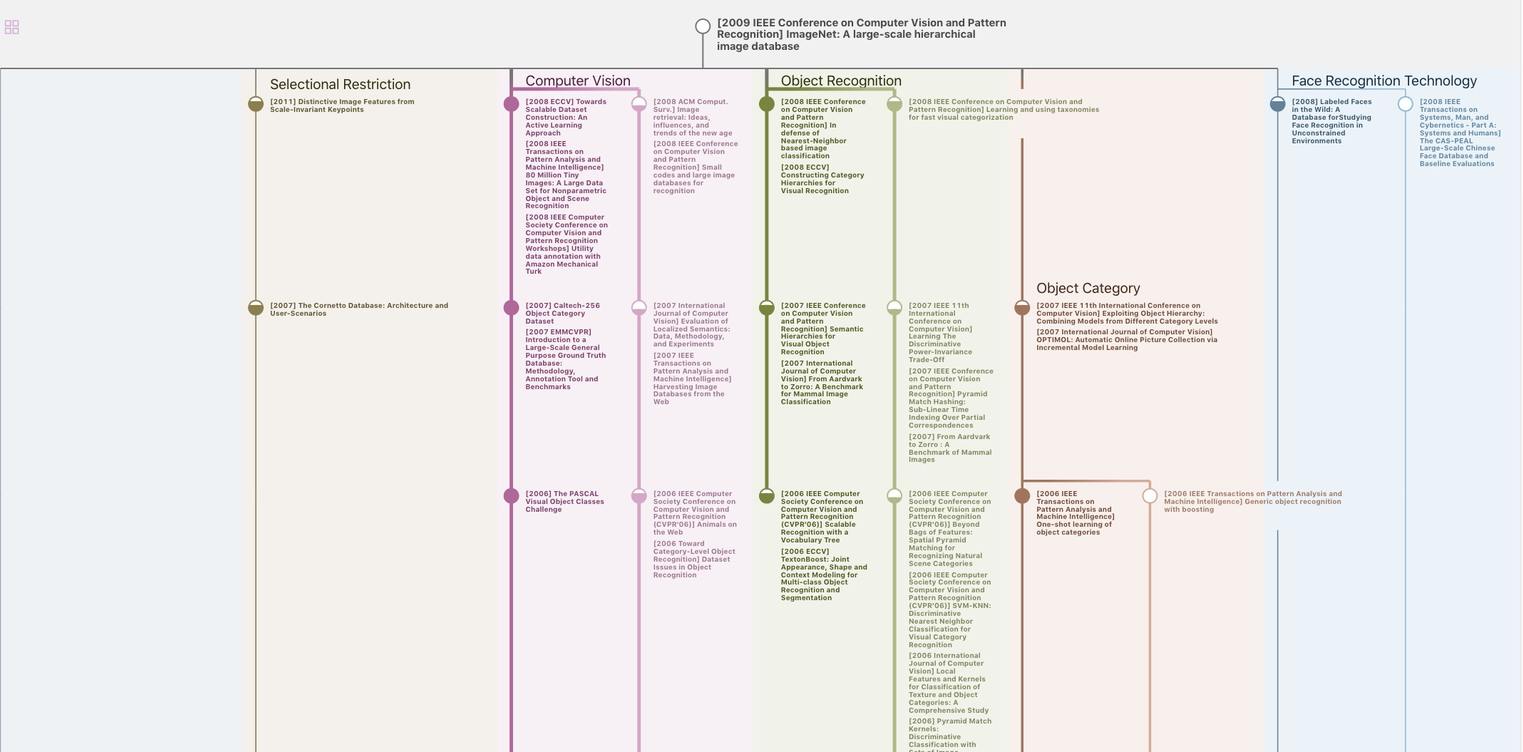
生成溯源树,研究论文发展脉络
Chat Paper
正在生成论文摘要