Learning to Synthesize Photorealistic Dual-pixel Images from RGBD frames
2023 IEEE International Conference on Computational Photography (ICCP)(2023)
摘要
As a special sensor that implicitly provides ordinal depth information, dual-pixel (DP) appears to be beneficial for various tasks such as defocus deblurring and monocular depth estimation. Recent advances in data-driven dual-pixel (DP) research are bottlenecked by the difficulties in reaching large-scale DP datasets, and a photorealistic image synthesis approach appears to be a credible solution. To benchmark the accuracy of various existing DP image simulators and facilitate data-driven DP image synthesis, this work presents a real-world DP dataset consisting of approximately 5000 high-quality pairs of sharp images, DP defocus blur images, detailed imaging parameters, and accurate depth maps. Based on this large-scale dataset, we also propose a holistic data-driven framework to synthesize photorealistic DP images, where a neural network replaces conventional handcrafted imaging models. Experiments show that our neural DP simulator can generate more photorealistic DP images than existing state-of-the-art methods and effectively benefit data-driven DP-related tasks. Our code and dataset are released at https://github.com/SILI1994/Dual-Pixel-Simulator.
更多查看译文
关键词
Dual pixel, Image synthesis, Dataset, Data-driven synthesis
AI 理解论文
溯源树
样例
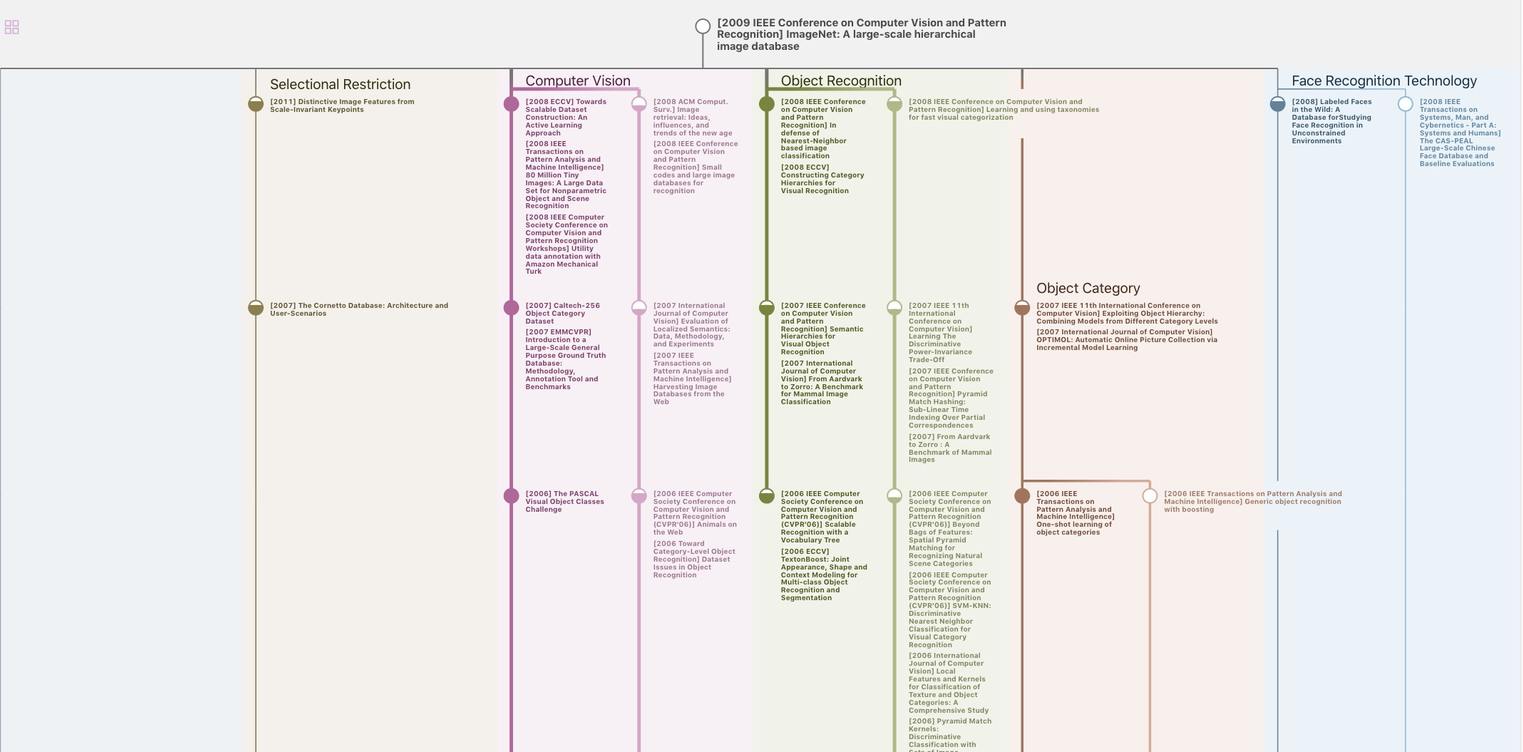
生成溯源树,研究论文发展脉络
Chat Paper
正在生成论文摘要