Neural Reconstruction through Scattering Media with Forward and Backward Losses
2023 IEEE International Conference on Computational Photography (ICCP)(2023)
摘要
Reconstructing an object behind a scattering medium needs to tackle different light scatterings inside the medium and in the free space. Major approaches, e.g., diffuse optical tomography and non-line-of-sight imaging, address either of the light scatterings. Confocal diffuse tomography (CDT) considers the media acting as a diffuse kernel on the free-space scattering and recovers the object by deconvolving the inside-medium scattering. Inspired by CDT, we present a Neural De-Scatterer to solve this challenging problem. We exploit neural implicit fields to represent the the free-space scattering and use a multilayer perceptron (MLP) to learn the density and albedo of the hidden object. Furthermore, we tailor a bi-directional training strategy to optimize the MLP with forward and backward losses and employ hash encoding for memory and computation efficiency. The Neural De-Scatterer enables us to reconstruct objects at arbitrary resolution. Comprehensive experiments with synthetic and real measurements demonstrate that our Neural De-Scatterer outperforms state-of-the-art methods. Our data and code are publicly available.
更多查看译文
关键词
Computational photography,Diffuse optical tomography,Non-line-of-sight imaging,Neural implicit representation
AI 理解论文
溯源树
样例
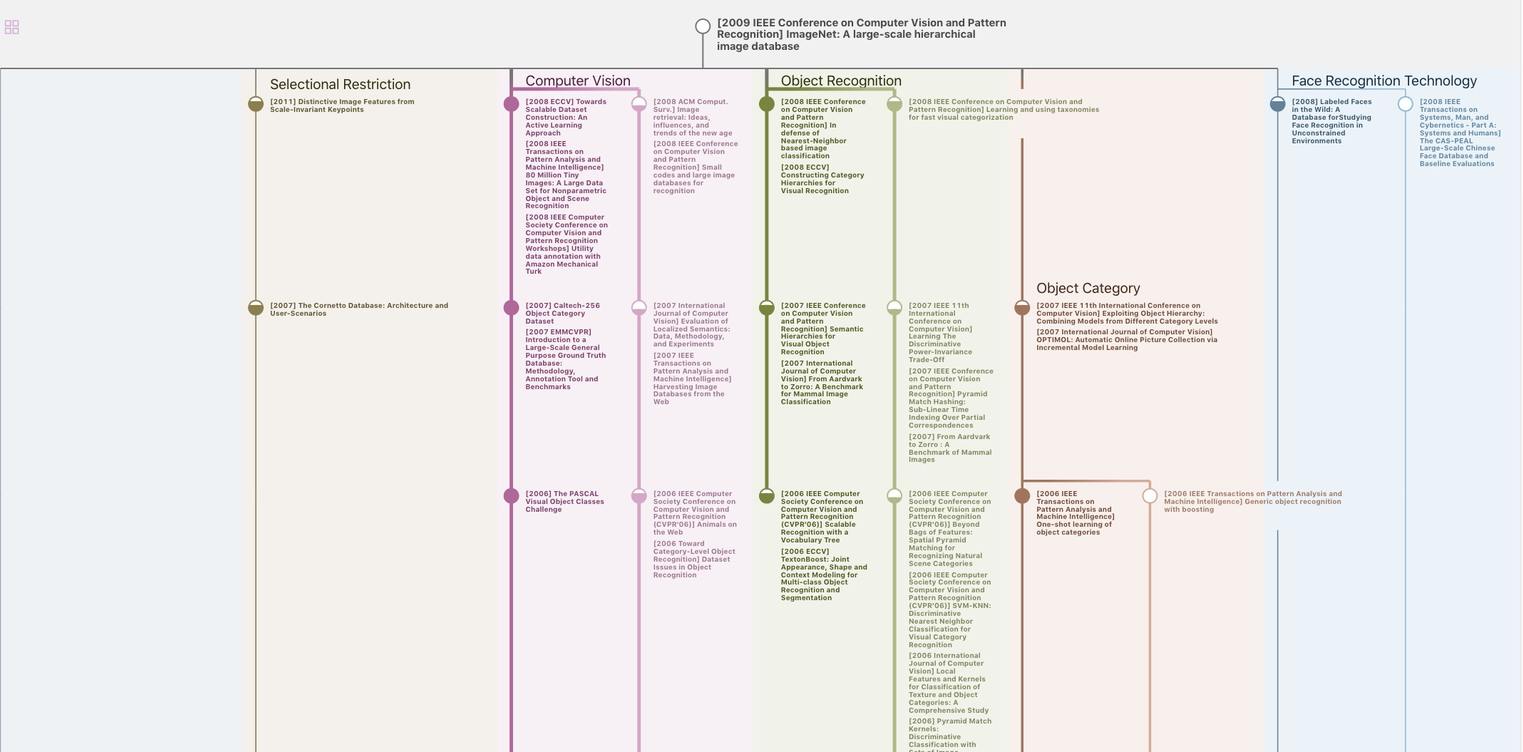
生成溯源树,研究论文发展脉络
Chat Paper
正在生成论文摘要