Utilization of relative context for text non-text region classification in offline documents using multi-scale dilated convolutional neural network
Multimedia Tools and Applications(2024)
摘要
Identification of text and non-text regions in a document image is necessary before feeding it to an Optical character recognition (OCR) engine for the generation of editable version. This is because OCR engines only process text regions. Presence of non-text may constrain their performance. This makes text non-text region classification a necessary step. So far, texture based feature descriptors are widely considered for document region classification. These descriptors mostly consider the local patterns to estimate the region texture. Even the convention neural networks (CNN) also emphasise on the local connectivity. However, for better characterization of the region texture it is necessary to capture the relative context too. To address this issue, in this paper, a multi-scale dilated convolution neural network is designed to classify the document regions as text or non-text. This network can effectively capture the local patterns as well as the relative context at different scale. The proposed method is evaluated on a publicly available dataset AUTNT (Khan and Mollah, Multimed Tools Appl 78(22):32159–32186) where it outperforms the benchmark result by 1.63% by obtaining 97.91% accuracy. The proposed method also outperforms some state-of-the-art methods while evaluated on the said dataset. Additionally, the performance of the proposed network is evaluated using two standard datasets MNIST and Fashion-MNIST to observe its applicability in multi-class problem. The network obtains 99.31% accuracy in MINST dataset and 90.68% accuracy in Fashion-MNIST dataset.
更多查看译文
关键词
Text non-text separation,Dilated convolution,CNN,Classification,Offline document,Multi scale dilation,AUTNT,OCR
AI 理解论文
溯源树
样例
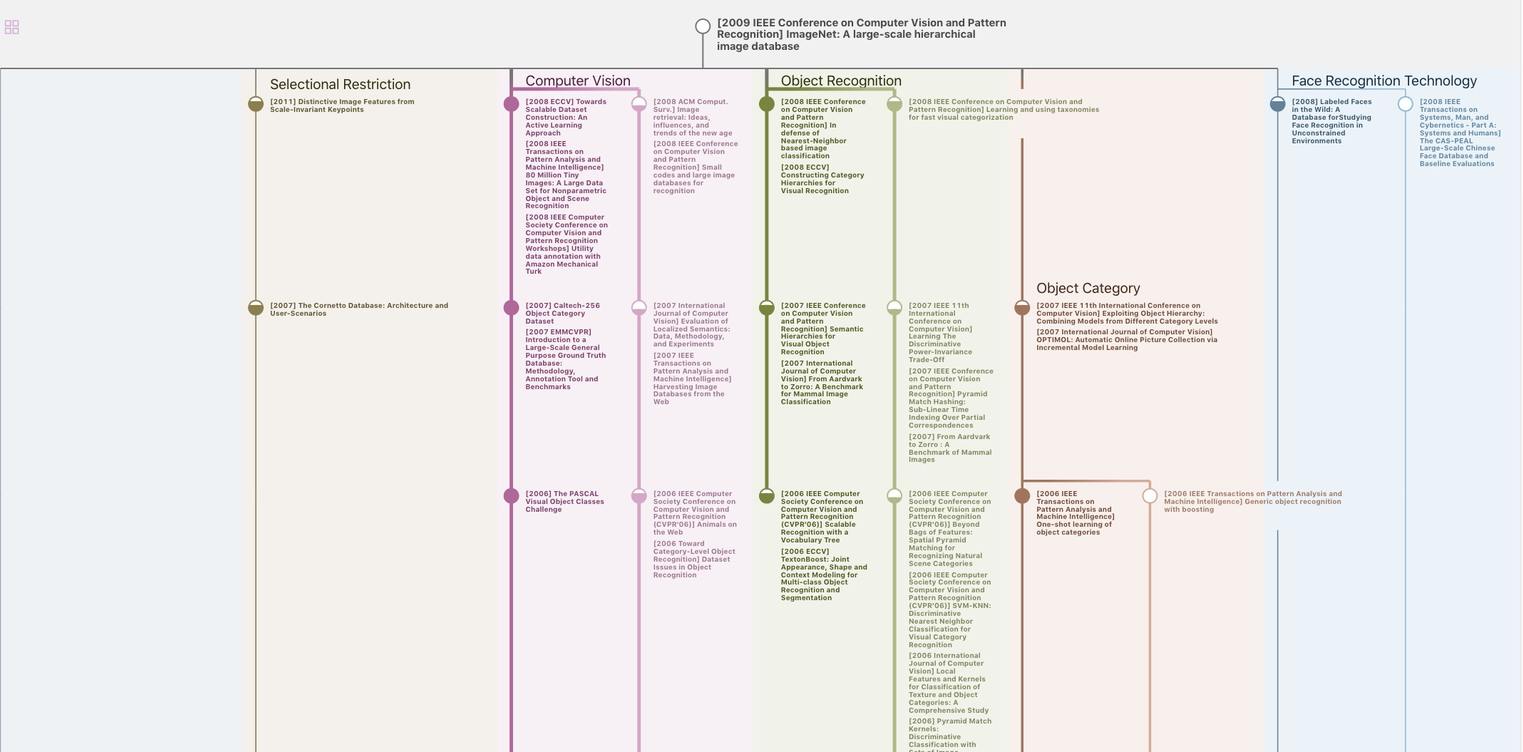
生成溯源树,研究论文发展脉络
Chat Paper
正在生成论文摘要