Dense-scale dynamic network with filter-varying atrous convolution for semantic segmentation
Applied Intelligence(2023)
摘要
Deep convolution neural networks (DCNNs) in deep learning have been widely used in semantic segmentation. However, the filters of most regular convolutions in DCNNs are spatially invariant to local transformations, which reduces localization accuracy and hinders the improvement of semantic segmentation. Dynamic convolution with pixel-level filters can enhance the localization accuracy through its region-awareness, but these are sensitive to objects with large-scale variations in semantic segmentation. To simultaneously address the low localization accuracy and objects with large-scale variations, we propose a filter-varying atrous convolution (FAC) to efficiently enlarge the per-pixel receptive fields pertaining to various objects. FAC mainly consists of a conditional-filter-generating network (CFGN) and a dynamic local filtering operation (DLFO). In the CFGN, a class probability map is used to generate the corresponding filters, making the FAC genuinely dynamic. In the DLFO, by replacing the sliding convolution operation one by one with a one-time dot product operation, the efficiency of the algorithm is greatly improved. Also, a dense scale module (DSM) is constructed to generate denser scales and larger receptive fields for exploring long-range contextual information. Finally, a dense-scale dynamic network (DsDNet) simultaneously enhances the localization accuracy and reduces the effect of large-scale variations of the object, by assigning FAC to different spatial locations at dense scales. In addition, to accelerate network convergence and improve segmentation accuracy, our network employs two pixel-wise cross-entropy loss functions. One is between the Backbone and DSM, and the other is at the network’s end. Extensive experiments on Cityscapes, PASCAL VOC 2012, and ADE20K datasets verify that the performance of our DsDNet is superior to the non-dynamic and multi-scale convolution neural networks.
更多查看译文
关键词
Semantic segmentation,Deep learning,Deep convolution neural networks (DCNNs),Dynamic convolution
AI 理解论文
溯源树
样例
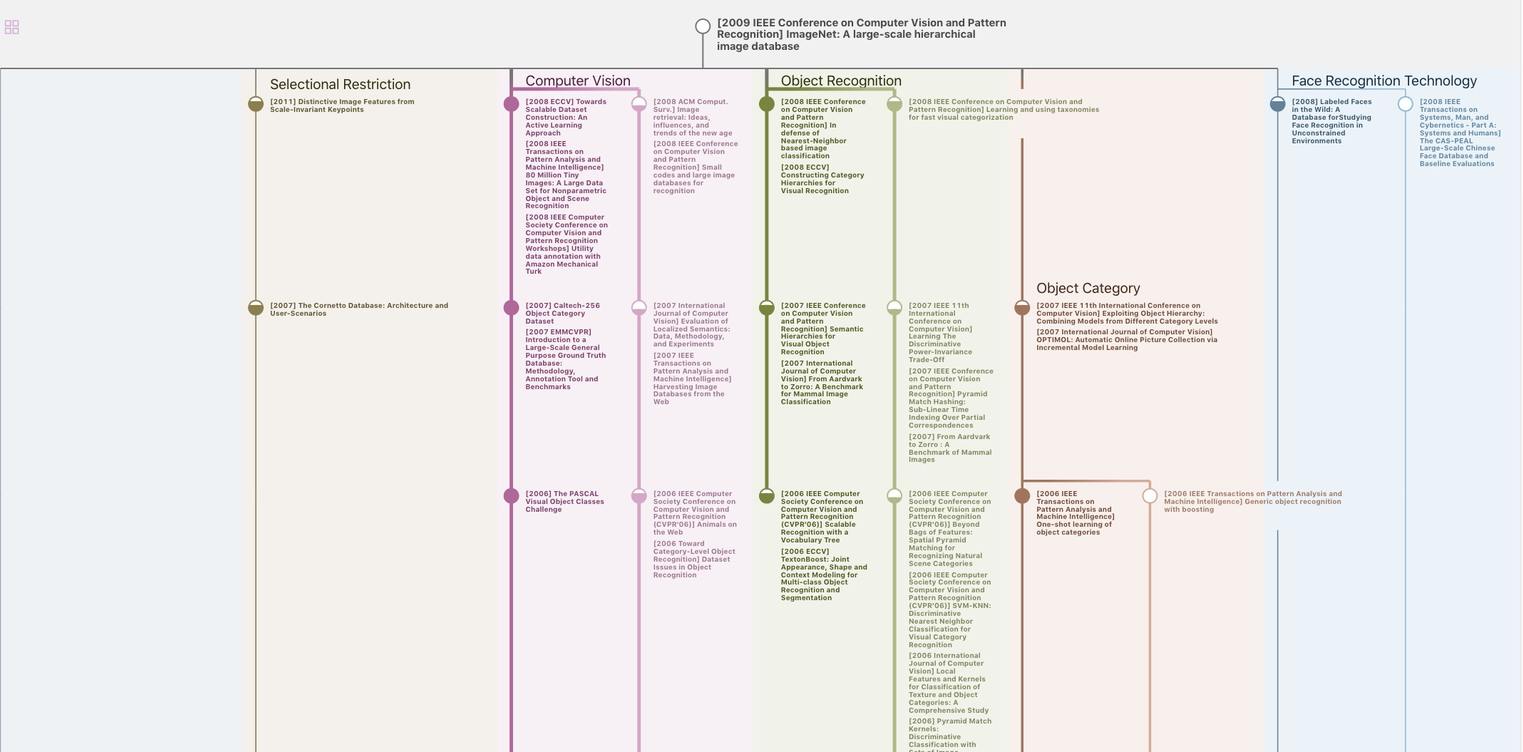
生成溯源树,研究论文发展脉络
Chat Paper
正在生成论文摘要