STint: Self-supervised Temporal Interpolation for Geospatial Data
CoRR(2023)
摘要
Supervised and unsupervised techniques have demonstrated the potential for temporal interpolation of video data. Nevertheless, most prevailing temporal interpolation techniques hinge on optical flow, which encodes the motion of pixels between video frames. On the other hand, geospatial data exhibits lower temporal resolution while encompassing a spectrum of movements and deformations that challenge several assumptions inherent to optical flow. In this work, we propose an unsupervised temporal interpolation technique, which does not rely on ground truth data or require any motion information like optical flow, thus offering a promising alternative for better generalization across geospatial domains. Specifically, we introduce a self-supervised technique of dual cycle consistency. Our proposed technique incorporates multiple cycle consistency losses, which result from interpolating two frames between consecutive input frames through a series of stages. This dual cycle consistent constraint causes the model to produce intermediate frames in a self-supervised manner. To the best of our knowledge, this is the first attempt at unsupervised temporal interpolation without the explicit use of optical flow. Our experimental evaluations across diverse geospatial datasets show that STint significantly outperforms existing state-of-the-art methods for unsupervised temporal interpolation.
更多查看译文
关键词
temporal interpolation,geospatial data,self-supervised
AI 理解论文
溯源树
样例
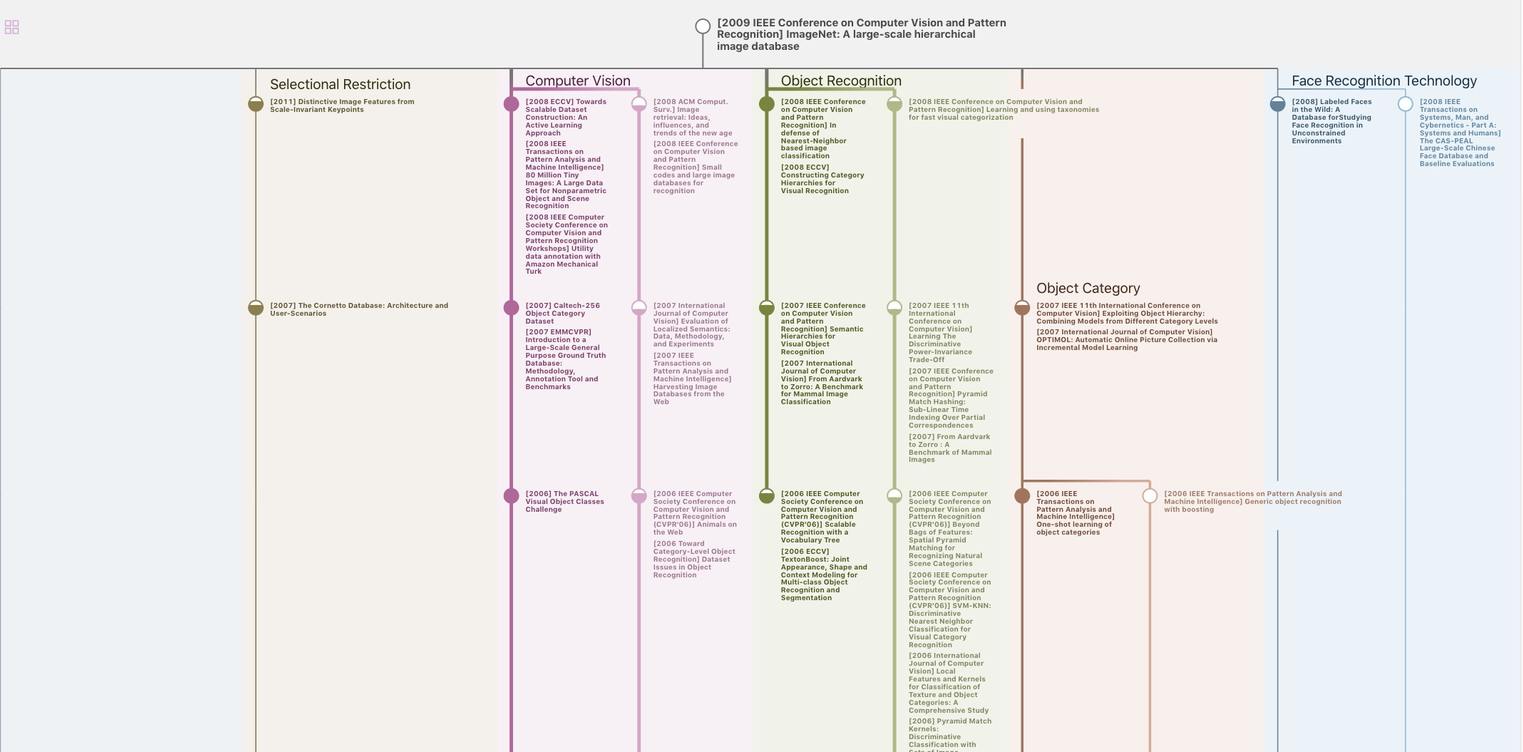
生成溯源树,研究论文发展脉络
Chat Paper
正在生成论文摘要