Exploring the Polarization of Axially Symmetric Supernovae with Unsupervised Deep Learning
arXiv (Cornell University)(2023)
摘要
The measurement of non-zero polarization can be used to infer the presence of departures from spherical symmetry in supernovae (SNe). The origin of the majority of the intrinsic polarization observed in SNe is in electron scattering, which induces a wavelength-independent continuum polarization that is generally observed to be low (<1%) for all SN types. The key indicator of asymmetry in SNe is the polarization observed across spectral lines, in particular the characteristic ``inverse P Cygni'' profile. The results of a suite of 900 Monte Carlo radiative transfer simulations are presented here. These simulations cover a range of possible axisymmetric structures (including unipolar, bipolar and equatorial enhancements) for the line forming region of the Ca II infrared triplet. Using a Variational Autoencoder, 7 key latent parameters are learned that describe the relationship between Stokes I and Stokes q, under the assumption of an axially symmetric line forming region and resonant scattering. Likelihood-free inference techniques are used to invert the Stokes I and q line profiles, in the latent space, to derive the underlying geometries. For axially symmetric structures, that yield an observable ``dominant axis'' on the Stokes $q-u$ plane, we propose the existence of a geometry ``conjugate" (which is indistinguishable under a rotation of $\pi /2$). Using this machine learning infrastructure, we attempt to identify possible geometries associated with spectropolarimetric observations of the Type Ib SN 2017gax.
更多查看译文
关键词
symmetric supernovae,polarization,deep learning
AI 理解论文
溯源树
样例
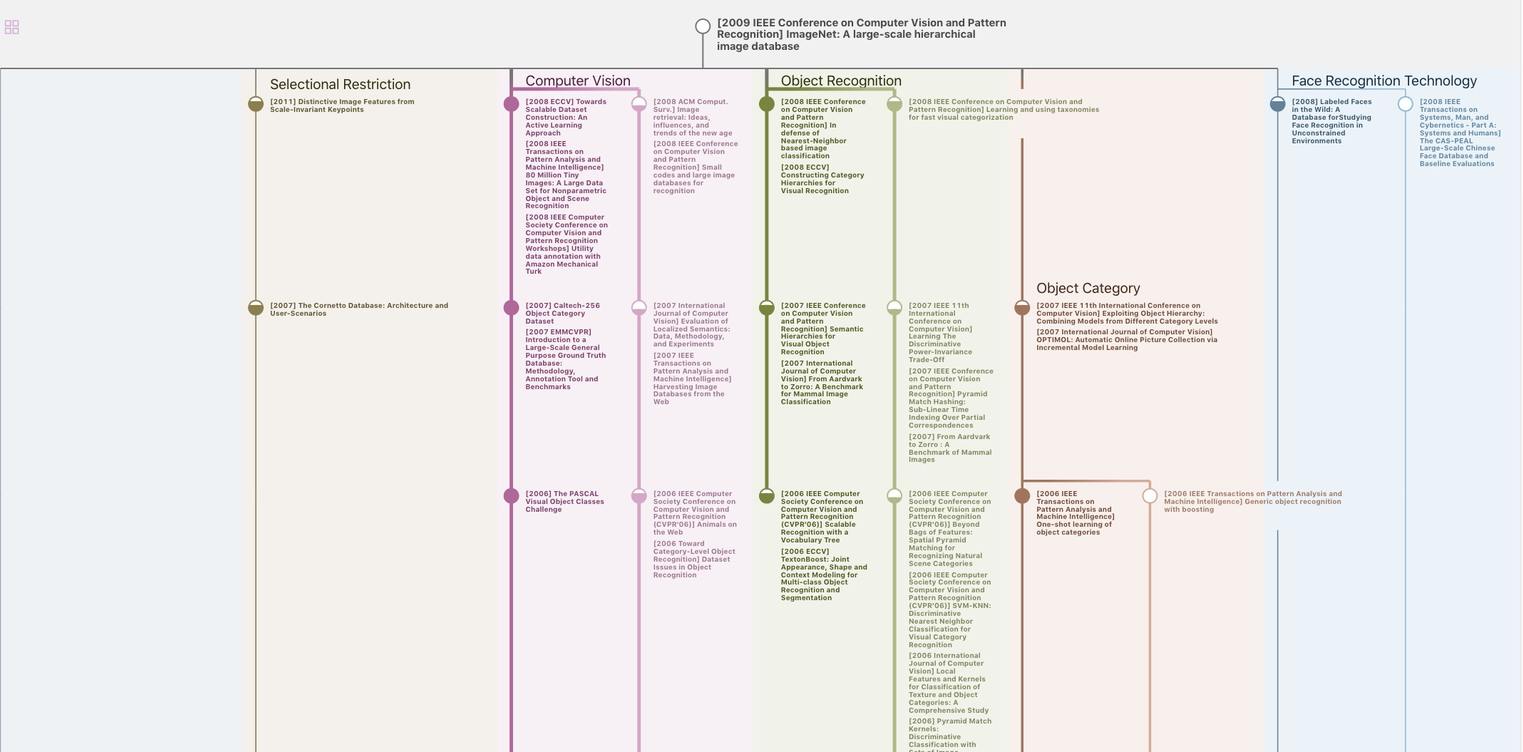
生成溯源树,研究论文发展脉络
Chat Paper
正在生成论文摘要