Uncertainty-Aware Graph Reasoning With Global Collaborative Learning for Remote Sensing Salient Object Detection
IEEE Geosci. Remote. Sens. Lett.(2023)
摘要
Recently, fully convolutional networks (FCNs) have contributed significantly to salient object detection (SOD) in optical remote sensing images (RSIs). However, owing to the limited receptive fields of FCNs, accurate and integral detection of salient objects in RSIs with complex edges and irregular topology is still challenging. Moreover, suffering from the low contrast and complicated background of RSIs, existing models often occur ambiguous or uncertain recognition. To remedy the above problems, we propose a novel hybrid modeling approach, i.e., uncertainty-aware graph reasoning with global collaborative learning (UG2L) framework. Specifically, we propose a graph reasoning pipeline to model the intricate relations among RSI patches instead of pixels and introduce an efficient graph reasoning block (GRB) to build graph representations. On top of it, a global context block (GCB) with a linear attention mechanism is proposed to explore the multiscale and global context collaboratively. Finally, we design a simple yet effective uncertainty-aware loss (UAL) to enhance the model's reliability for better prediction of saliency or nonsaliency. Experimental and visual results on three datasets show the superiority of the proposed UG2L. Code is available at https://github.com/lyf0801/UG2L.
更多查看译文
关键词
Global collaborative learning,graph reasoning,optical remote sensing image (RSI),salient object detection (SOD),uncertainty-aware loss (UAL)
AI 理解论文
溯源树
样例
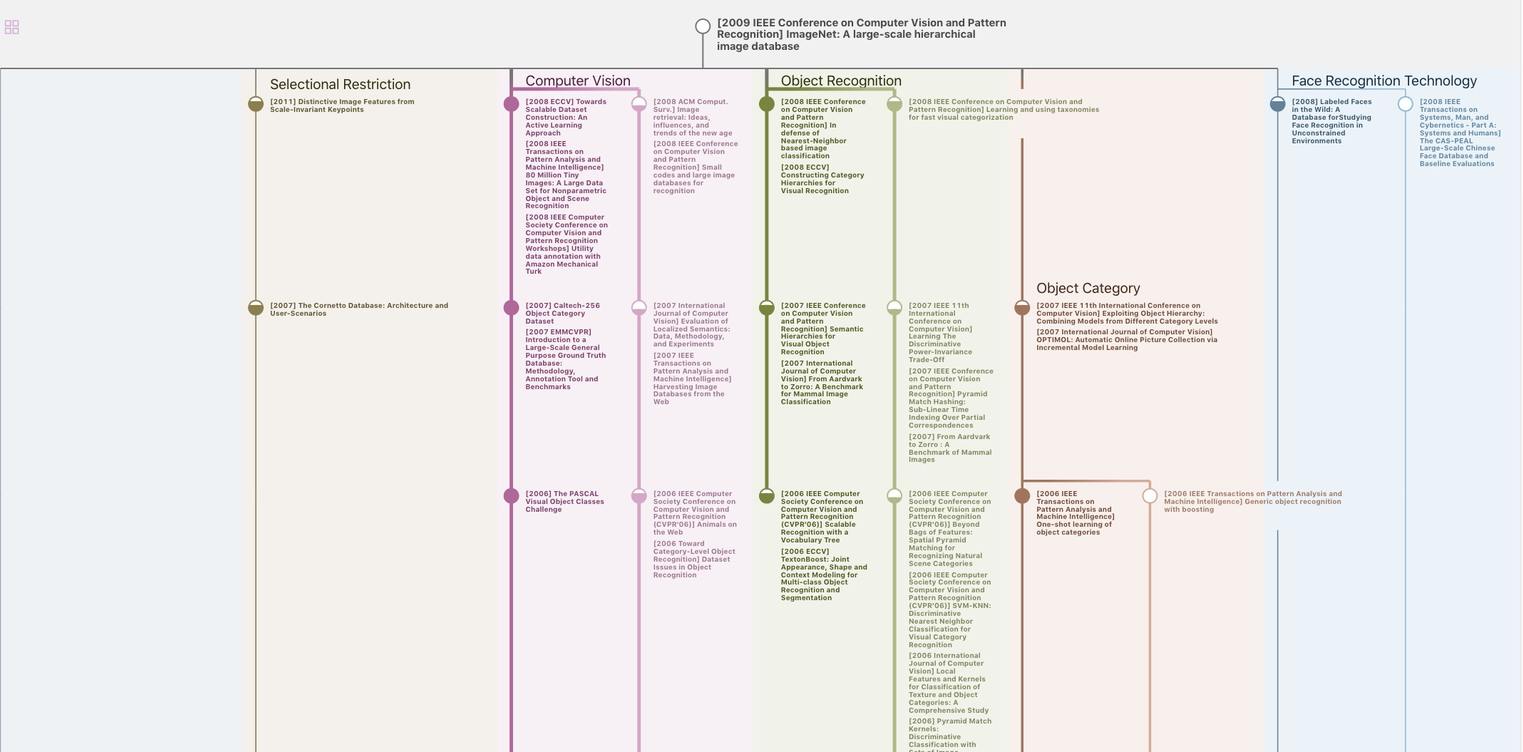
生成溯源树,研究论文发展脉络
Chat Paper
正在生成论文摘要