Encoding Human Visual Perception Into Deep Hashing for Aerial Image Classification.
IEEE J. Sel. Top. Appl. Earth Obs. Remote. Sens.(2023)
摘要
Accurately calculating the labels of each high-resolution image is an unavoidable technique in remote sensing. In this article, we propose a novel image assortment model that personate each aerial image by optimally encoding a gaze shifting path (GSP). At the same time, wrong semantic model can get absent with it. More specifically, for each aerial image, we reference visually/semantically noticeable representational rogue interiors. To encode their analysis attributes, we mean a small graph comprise of spatially conterminous motivational wall, and extract GSPs on it by active literature algorithm rules. GSP can accurately capture humans perception over many aerial image areas when the notice senses are placed in each image. Subsequently, a double deep learning framework is proposed to intelligently exploit the semantics of these GSPs, with three attributes: label noises reduction, visual manner-unchanging semantics, and adaptive data chart updates are seamlessly integrated. The proposed framework can iteratively solved, with each graphlet re-form into a base. Finally, the GSP-compliant summaries in each aerial have shown the quantized vectors for visual understanding. To qualitatively and quantitatively assess how GSP affects information aerial image classification, we notice that the phantom copy of our progress classification is more accurate than its competitors, and the GSPs propagated by Alzheimer's patients are discriminative from those produced by typical observers, making the classification competitive.
更多查看译文
关键词
Aerial image, hashing, label noises, semantics
AI 理解论文
溯源树
样例
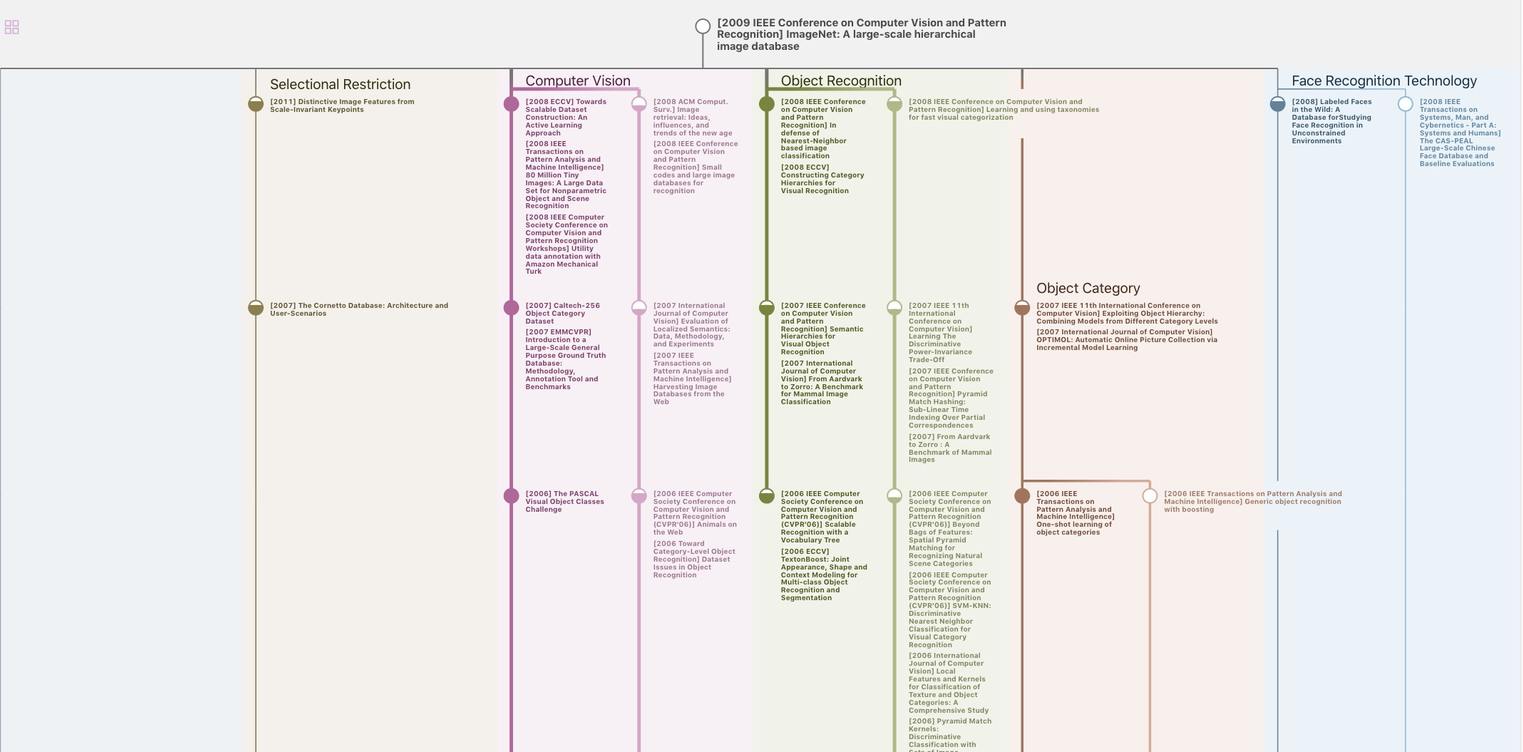
生成溯源树,研究论文发展脉络
Chat Paper
正在生成论文摘要