Looking Closer to the Transferability Between Natural and Medical Images in Deep Learning.
IEEE Access(2023)
摘要
Transfer-learning has rapidly become one of the most sophisticated and effective techniques in dealing with medical datasets. The most common transfer-learning method uses of a state-of-the-art model and its corresponding parameters as the starting point for new tasks. Recent studies have found that transfer-learning between medical and natural images has minimal advantages, attributed to their different characteristics, even with sufficient data and iterations. This study employs a meta-learning technique, building upon the traditional transfer learning approach, to explore the potential of natural tasks as a starting point for analyzing medical images. In addition, this study investigates the performance of transferring the searched augmentation from natural to medical images. Several studies proposing search algorithms for data augmentation argue that the augmentation techniques can be effectively transferred across different datasets. The results revealed that the transferability between natural and medical images leads to reduced performance owing to the characteristic difference between medical and natural searched augmentation.
更多查看译文
关键词
Data augmentation, medical image dataset, meta-learning, natural images dataset, transfer-learning
AI 理解论文
溯源树
样例
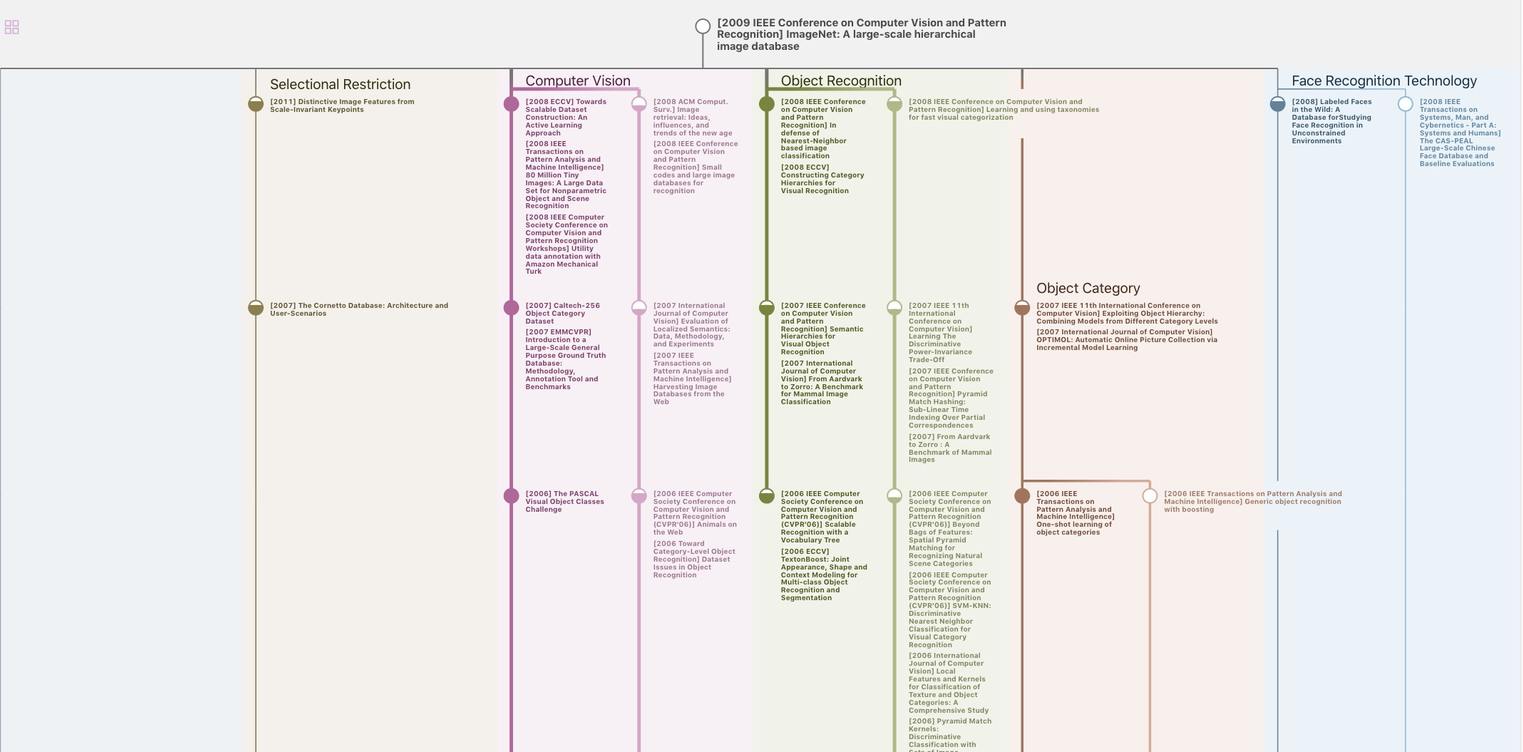
生成溯源树,研究论文发展脉络
Chat Paper
正在生成论文摘要