Multiple Open Switch Fault Diagnosis of Three Phase Voltage Source Inverter Using Ensemble Bagged Tree Machine Learning Technique
IEEE Access(2023)
摘要
Three-phase converters based on insulated-gate bipolar transistors (IGBTs) are widely used in various industrial applications. Faults in IGBTs can significantly affect the operation and safety of the power electronic equipment and loads. It is critical to accurately detect power inverter faults as soon as they occur to ensure system availability and high-power quality. This study provides a novel integration of signal and data-driven fault-diagnosis approaches for detecting open-circuit switch faults in three-phase inverters. The proposed technique uses the average root-mean-square (RMS) ratio of the phase current as the key extraction feature. This feature can be used to estimate the fault types and faulty switches (es) irrespective of changes in the running load. Ensemble-bagged machine learning classification was used to accurately predict the faulty switch of the inverter. The results demonstrate the ability of the proposed fault diagnosis technique to identify single-, double-, and triple-switch fault (s). The experimental results also attested to the simulation of multiple fault diagnosis. A unique feature of this technique is its ability to estimate faulty switches under various inverter-operating conditions.
更多查看译文
关键词
Ensemble bagged,fault diagnosis,open-circuit fault,voltage source inverter,IGBT
AI 理解论文
溯源树
样例
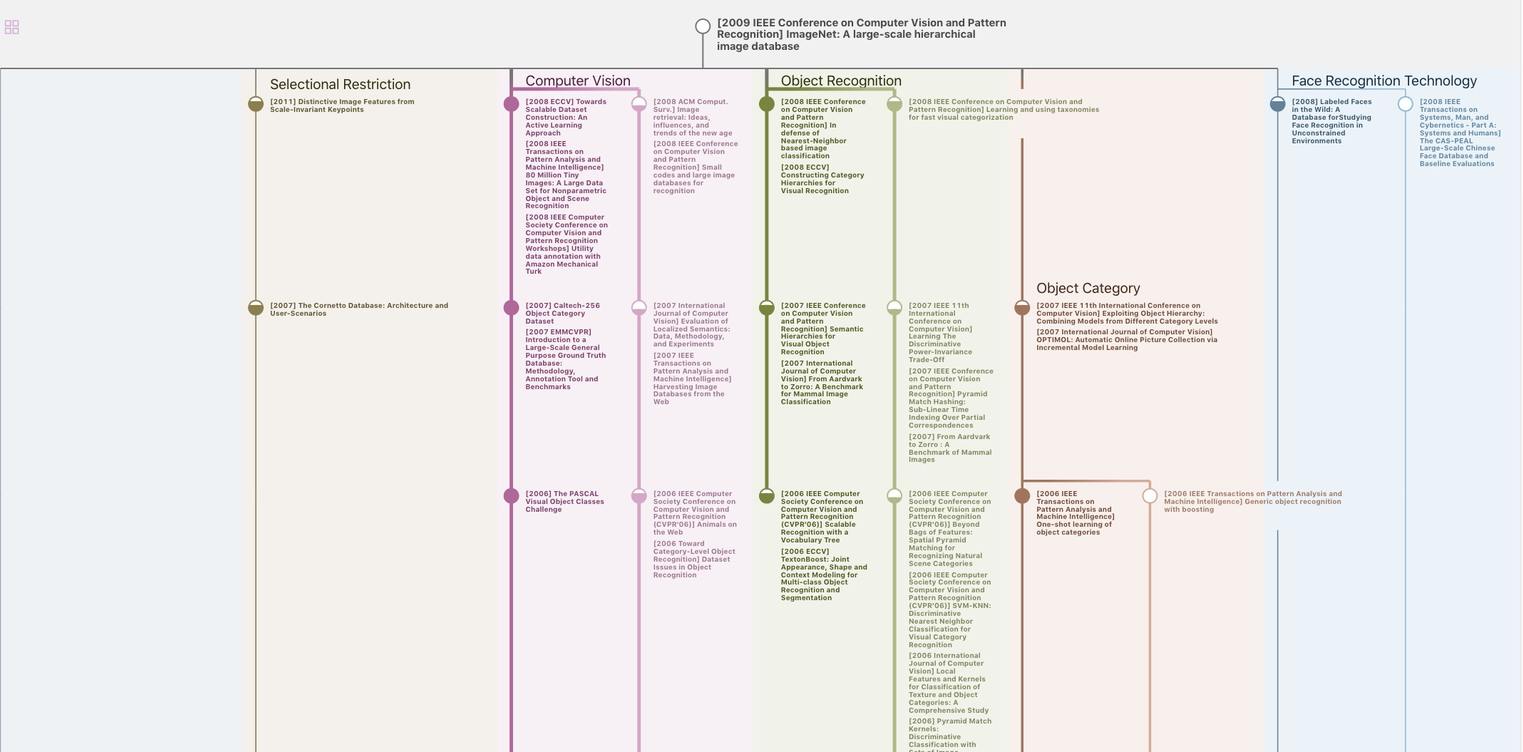
生成溯源树,研究论文发展脉络
Chat Paper
正在生成论文摘要